A Lightweight GAN Network for Large Scale Fingerprint Generation
IEEE ACCESS(2020)
摘要
Generating fingerprint images for biometric purposes is both necessary and challenging. In this study, we presented a fingerprint generation approach based on generative adversarial network. To ensure GAN training stability, we have introduced conditional loss doping that allows a continuous flow of gradients. Our study utilizes a careful combination of a residual network and spectral normalization to generate fingerprints. The proposed average residual connection shows more immunity against vanishing gradients than a simple residual connection. Spectral normalization allows our network to enjoy reduced variance in weight generation, which further stabilizes the training. Proposed scheme uses spectral bounding only in the input and the fully connected layers. Our network synthesized fingerprints up to 256 by 256 in size. We used the multi-scale structural similarity (MS-SSIM) metric for measuring the diversity of the generated samples. Our model has achieved 0.23 MS-SSIM scores for the generated fingerprints. The MS-SSIM score indicates that the proposed scheme is more likely to produce more diverse images and less likely to face mode collapse.
更多查看译文
关键词
Gallium nitride,Training,Generative adversarial networks,Generators,Stability analysis,Image resolution,Measurement,Skip connection,spectral normalization,loss doping,mode collapse,diversity
AI 理解论文
溯源树
样例
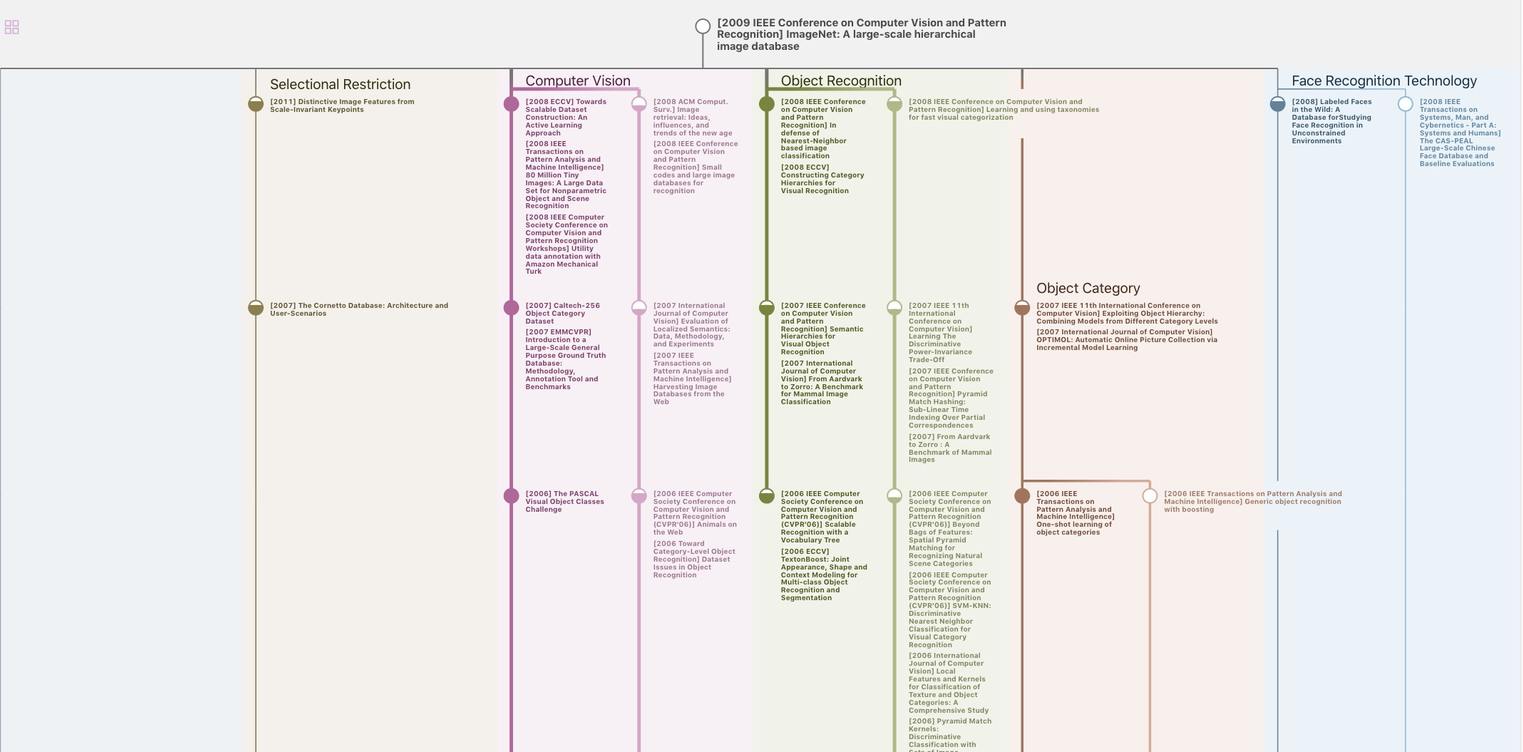
生成溯源树,研究论文发展脉络
Chat Paper
正在生成论文摘要