Classification of High-Spatial-Resolution Remote Sensing Scenes Method Using Transfer Learning and Deep Convolutional Neural Network
IEEE JOURNAL OF SELECTED TOPICS IN APPLIED EARTH OBSERVATIONS AND REMOTE SENSING(2020)
摘要
The deep convolutional neural network (DeCNN) is considered one of promising techniques for classifying the high-spatial-resolution remote sensing (HSRRS) scenes, due to its powerful feature extraction capabilities. It is well-known that huge high-quality labeled datasets are required for achieving the better classification performances and preventing overfitting, during the training DeCNN model process. However, the lack of high-quality datasets limits the applications of DeCNN. In order to solve this problem, in this article, we propose a HSRRS image scene classification method using transfer learning and the DeCNN (TL-DeCNN) model in a few shot HSRRS scene samples. Specifically, three typical DeCNNs of VGG19, ResNet50, and InceptionV3, trained on the ImageNet2015, the weights of their convolutional layer for that of the TL-DeCNN are transferred, respectively. Then, TL-DeCNN just needs to fine-tune its classification module on the few shot HSRRS scene samples in a few epochs. Experimental results indicate that our proposed TL-DeCNN method provides absolute dominance results without overfitting, when compared with the VGG19, ResNet50, and InceptionV3, directly trained on the few shot samples.
更多查看译文
关键词
Deep convolutional neural network (DeCNN),few shot,high-spatial-resolution remote sensing (HSRRS),scene classification,transfer learning
AI 理解论文
溯源树
样例
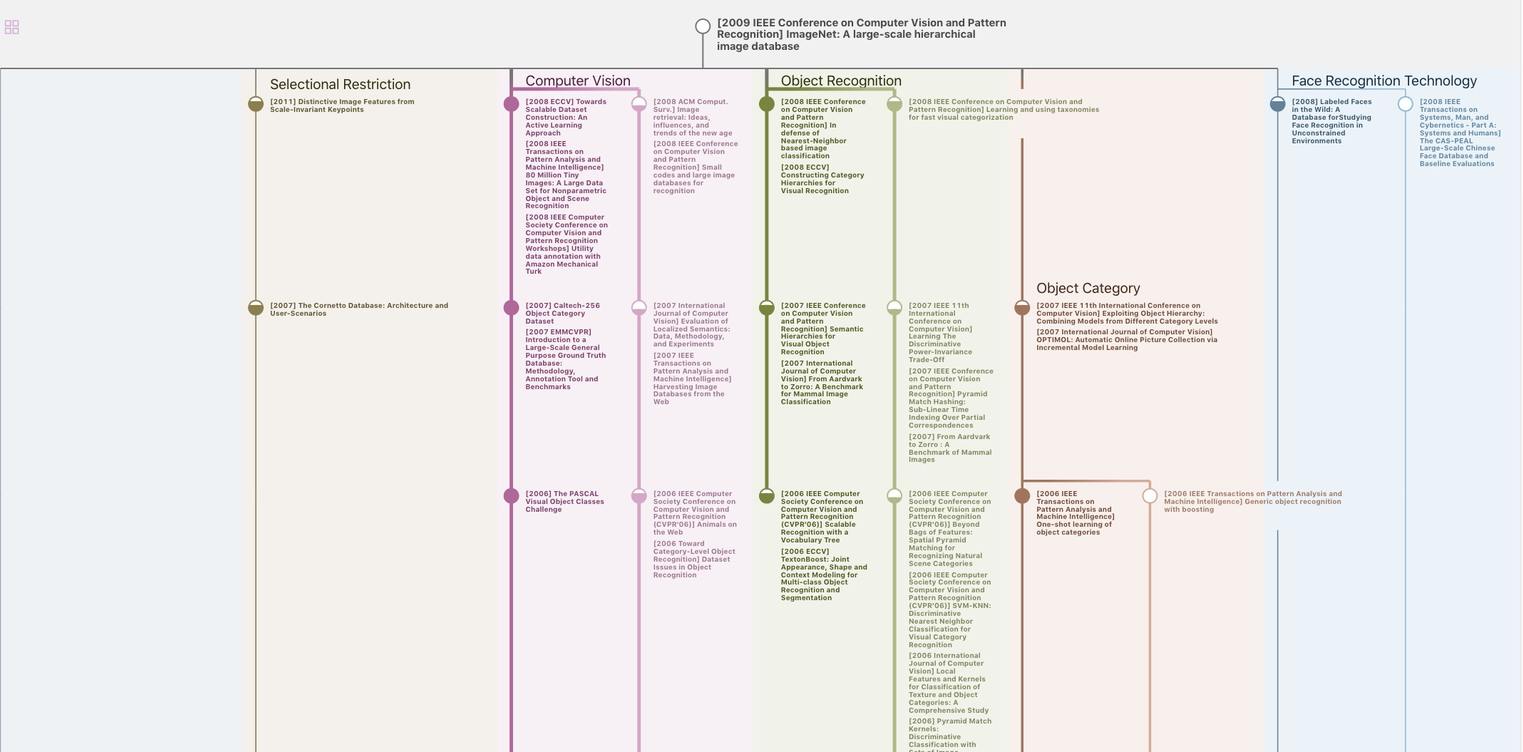
生成溯源树,研究论文发展脉络
Chat Paper
正在生成论文摘要