Self-supervised Learning for Semi-supervised Time Series Classification.
PAKDD (1)(2020)
摘要
Self-supervised learning is a promising new technique for learning representative features in the absence of manual annotations. It is particularly efficient in cases where labeling the training data is expensive and tedious, naturally linking it to the semi-supervised learning paradigm. In this work, we propose a new semi-supervised time series classification model that leverages features learned from the self-supervised task on unlabeled data. The idea is to exploit the unlabeled training data with a forecasting task which provides a strong surrogate supervision signal for feature learning. We draw from established multi-task learning approaches and model forecasting as an auxiliary task to be optimized jointly with the main task of classification. We evaluate our proposed method on benchmark time series classification datasets in semi-supervised setting and are able to show that it significantly outperforms the state-of-the-art baselines.
更多查看译文
关键词
classification,time series,learning,self-supervised,semi-supervised
AI 理解论文
溯源树
样例
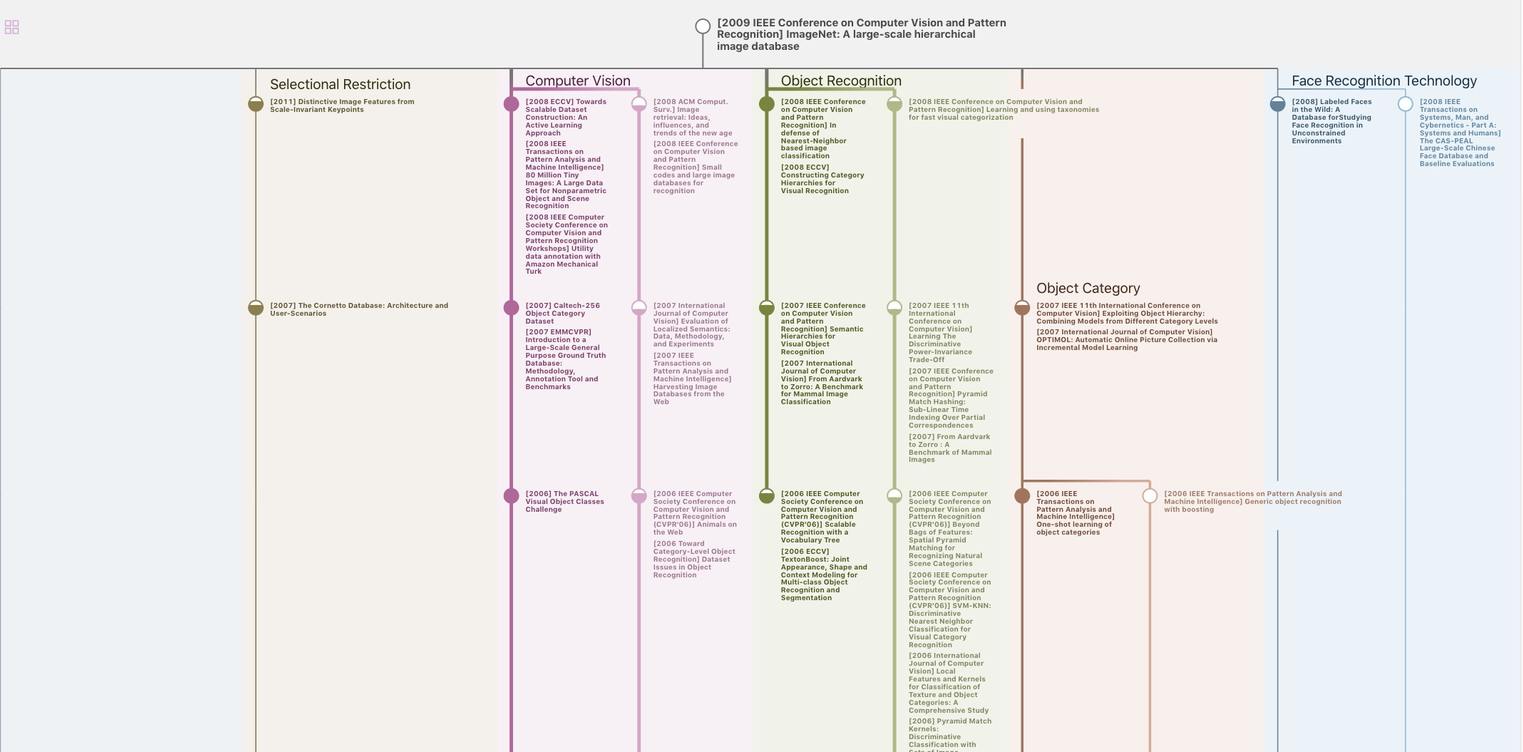
生成溯源树,研究论文发展脉络
Chat Paper
正在生成论文摘要