Trajectory-Tracking-Based Adaptive Neural Network Sliding Mode Controller for Robot Manipulators
JOURNAL OF COMPUTING AND INFORMATION SCIENCE IN ENGINEERING(2020)
摘要
Unpredictable disturbances and chattering are the major challenges of the robot manipulator control. In recent years, trajectory-tracking-based controllers have been recognized by many researchers as the most promising method to understand robot dynamics with uncertainties and improve robot control. However, reliable trajectory-tracking-based controllers require high model precision and complexity. To develop an agile and straightforward method to mitigate the impact caused by uncertain disturbance and chattering, this study proposed an adaptive neural network sliding mode controller based on the super-twisting algorithm. The proposed model not only can minimize the tracking error but also improve the system robustness with a simpler structure. Moreover, the proposed controller has the following two distinctive features: (1) the weights of the radial basis function (RBF network) are designed to be adjusted in real-time and (2) the prior knowledge of the actual robot system is not required. The analytical model of the proposed controller was proved to be stable and ensured by the Lyapunov theory. To validate the proposed model, this study also conducted a comparative simulation on a two-link robot manipulator system with the conventional sliding mode controller and the model-based controller. The results suggest the proposed model improved the control accuracy and had fewer chattering.
更多查看译文
关键词
trajectory-tracking-based controllers,adaptive neural network model,super-twisting algorithm,robot manipulators,artificial intelligence,machine learning for engineering applications,manufacturing automation
AI 理解论文
溯源树
样例
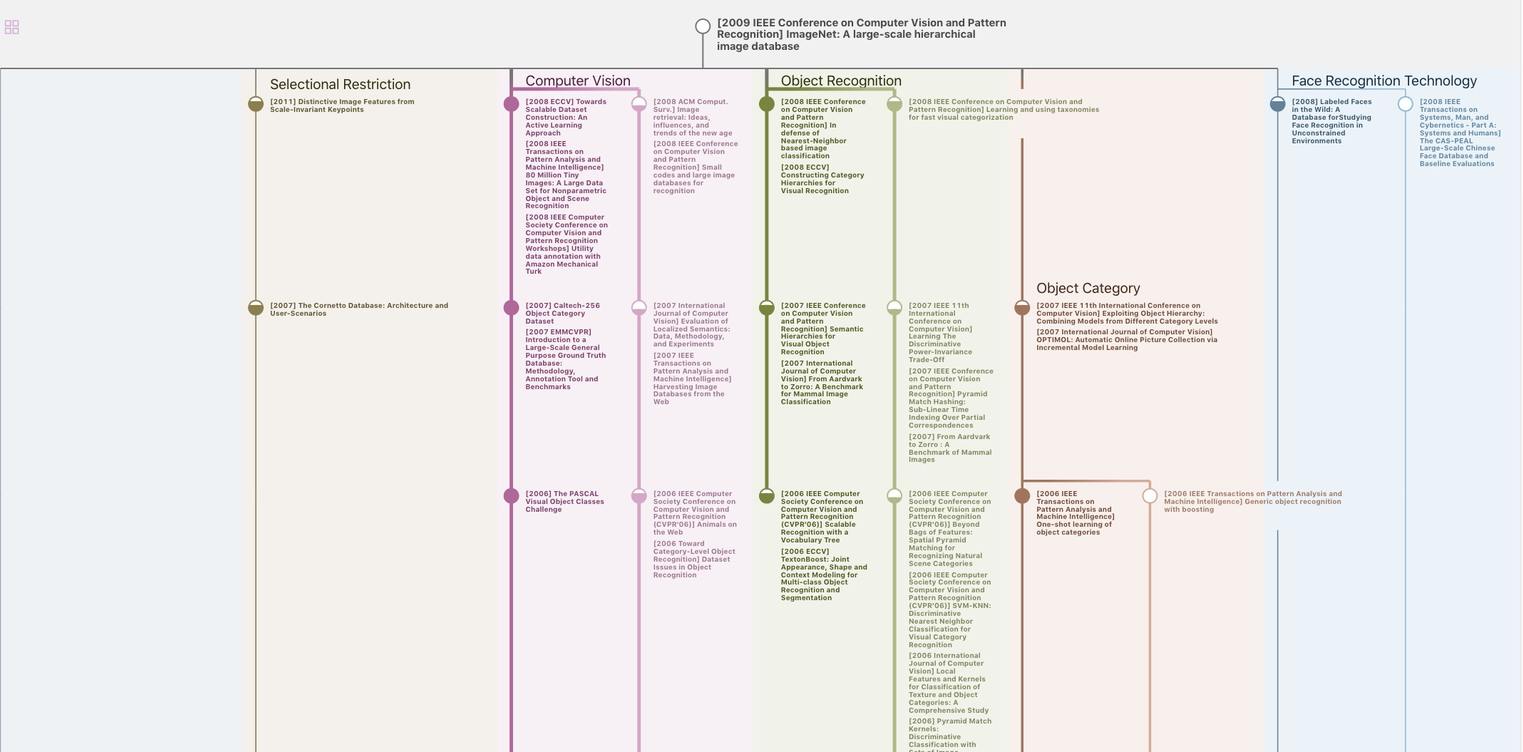
生成溯源树,研究论文发展脉络
Chat Paper
正在生成论文摘要