On the Performance of Oversampling Techniques for Class Imbalance Problems.
PAKDD (2)(2020)
摘要
Although over 90 oversampling approaches have been developed in the imbalance learning domain, most of the empirical study and application work are still based on the “classical” resampling techniques. In this paper, several experiments on 19 benchmark datasets are set up to study the efficiency of six powerful oversampling approaches, including both “classical” and new ones. According to our experimental results, oversampling techniques that consider the minority class distribution (new ones) perform better in most cases and RACOG gives the best performance among the six reviewed approaches. We further validate our conclusion on our real-world inspired vehicle datasets and also find applying oversampling techniques can improve the performance by around 10%. In addition, seven data complexity measures are considered for the initial purpose of investigating the relationship between data complexity measures and the choice of resampling techniques. Although no obvious relationship can be abstracted in our experiments, we find F1v value, a measure for evaluating the overlap which most researchers ignore, has a strong negative correlation with the potential AUC value (after resampling).
更多查看译文
关键词
Class imbalance, Minority class distribution, Data complexity measures
AI 理解论文
溯源树
样例
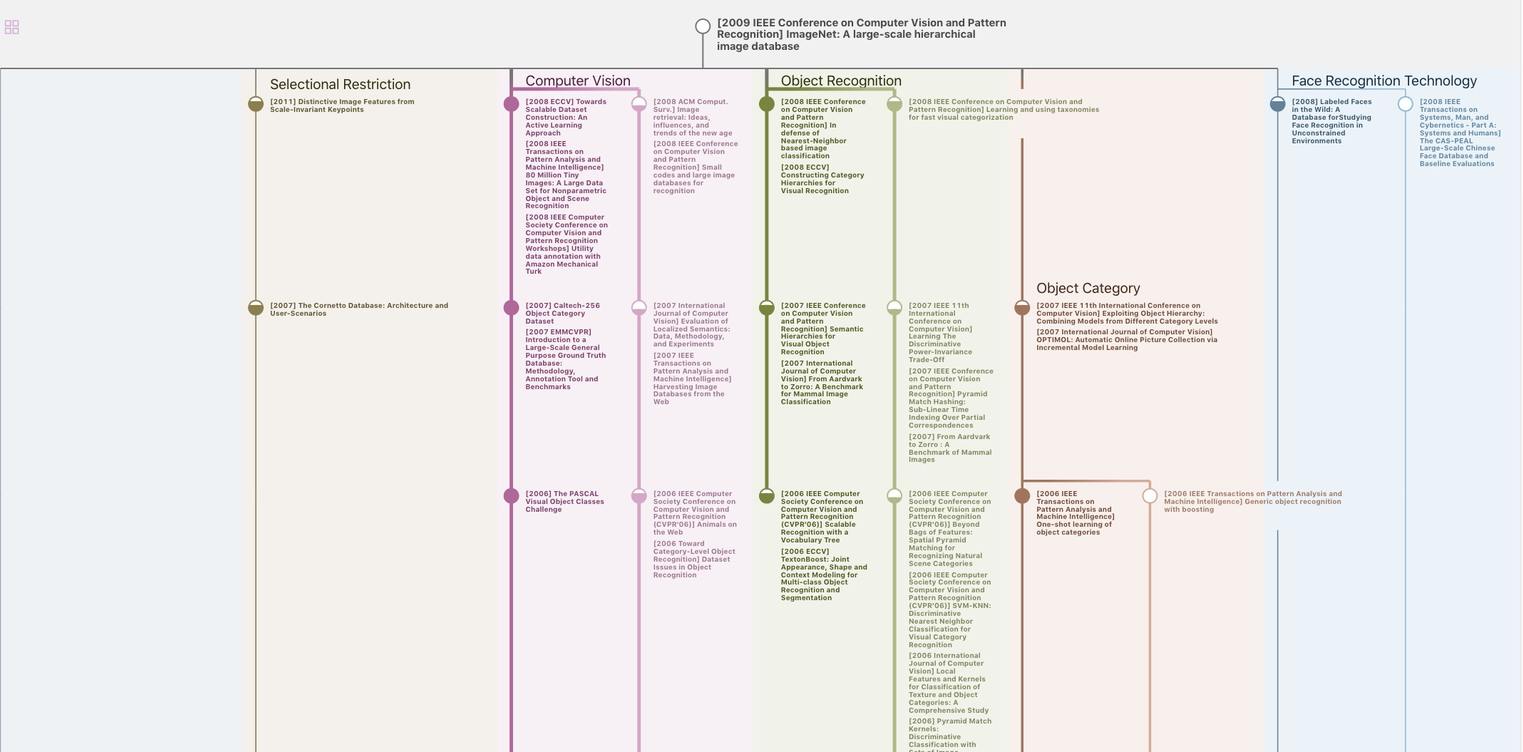
生成溯源树,研究论文发展脉络
Chat Paper
正在生成论文摘要