Digital Twin Enhanced Dynamic Job-Shop Scheduling
JOURNAL OF MANUFACTURING SYSTEMS(2021)
摘要
For dynamic scheduling, which is daily decision-making in a job-shop, machine availability prediction, distur-bance detection and performance evaluation are always common bottlenecks. Previous research efforts on addressing the bottlenecks primarily emphasize on the analysis of data from the physical job-shop, but with little connection and convergence with its virtual models and simulated data. By introducing digital twin (DT), further convergence between physical and virtual spaces of the job-shop can be achieved, which greatly enables dynamic scheduling. DT fuses both real and simulated data to provide more information for the prediction of machine availability on one hand; and on the other hand, it helps to detect disturbances through comparing the physical machine with its continuously updated digital counterpart in real time, triggering timely rescheduling when needed. It also enables comprehensive performance evaluation for rescheduling using multiple-dimension models, which can describe geometric properties, physics parameters and behaviors of the machines. In the paper, a five-dimension DT for a machine in the job-shop is introduced first, then the DT-based machine availability prediction, disturbance detection and performance evaluation methods are explored. Based on this, a DT-enhanced dynamic scheduling methodology is proposed. A scheduling process of making hydraulic valves in a machining job-shop is taken as a case study to illustrate the effectiveness and advantages of the proposed method.
更多查看译文
关键词
Digital Twin, Dynamic scheduling, Rescheduling, Modeling
AI 理解论文
溯源树
样例
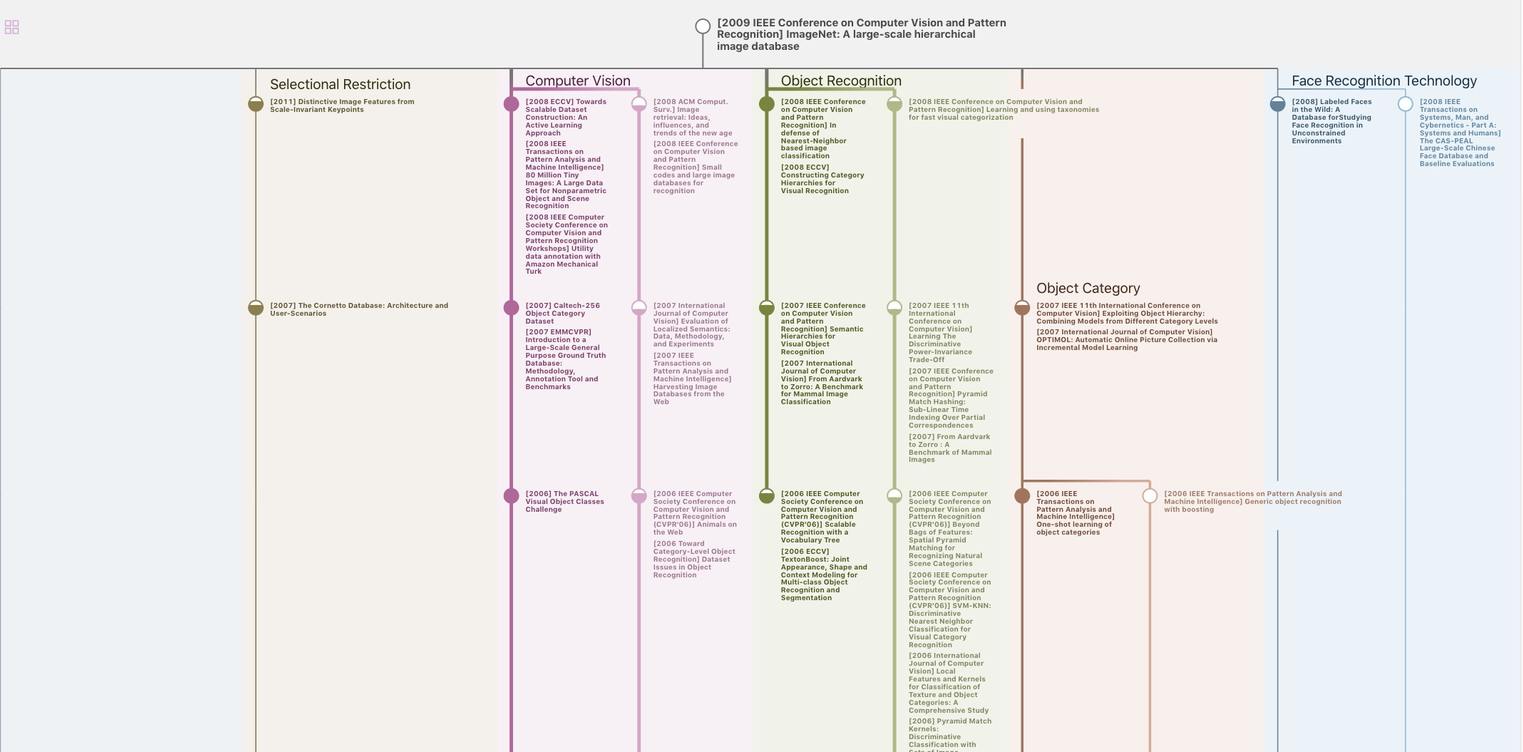
生成溯源树,研究论文发展脉络
Chat Paper
正在生成论文摘要