Speaker Verification by Partial AUC Optimization With Mahalanobis Distance Metric Learning
IEEE-ACM TRANSACTIONS ON AUDIO SPEECH AND LANGUAGE PROCESSING(2020)
摘要
Receiver operating characteristic (ROC) and detection error tradeoff (DET) curves are two widely used evaluation metrics for speaker verification. They are equivalent since the latter can be obtained by transforming the former's true positive y-axis to false negative y-axis and then re-scaling both axes by a probit operator. Real-world speaker verification systems, however, usually work on part of the ROC curve instead of the entire ROC curve given an application. Therefore, we propose in this article to use the area under part of the ROC curve (pAUC) as a more efficient evaluation metric for speaker verification. A Mahalanobis distance metric learning based back-end is applied to optimize pAUC, where the Mahalanobis distance metric learning guarantees that the optimization objective of the back-end is a convex one so that the global optimum solution is achievable. To improve the performance of the state-of-the-art speaker verification systems by the proposed back-end, we further propose two feature preprocessing techniques based on length-normalization and probabilistic linear discriminant analysis respectively. We evaluate the proposed systems on the major languages of NIST SRE16 and the core tasks of SITW. Experimental results show that the proposed back-end outperforms the state-of-the-art speaker verification back-ends in terms of seven evaluation metrics.
更多查看译文
关键词
Measurement,Feature extraction,Acoustics,Detection algorithms,Training,Speech processing,Optimization,Metric learning,pAUC,speaker verification,squared Mahalanobis distance
AI 理解论文
溯源树
样例
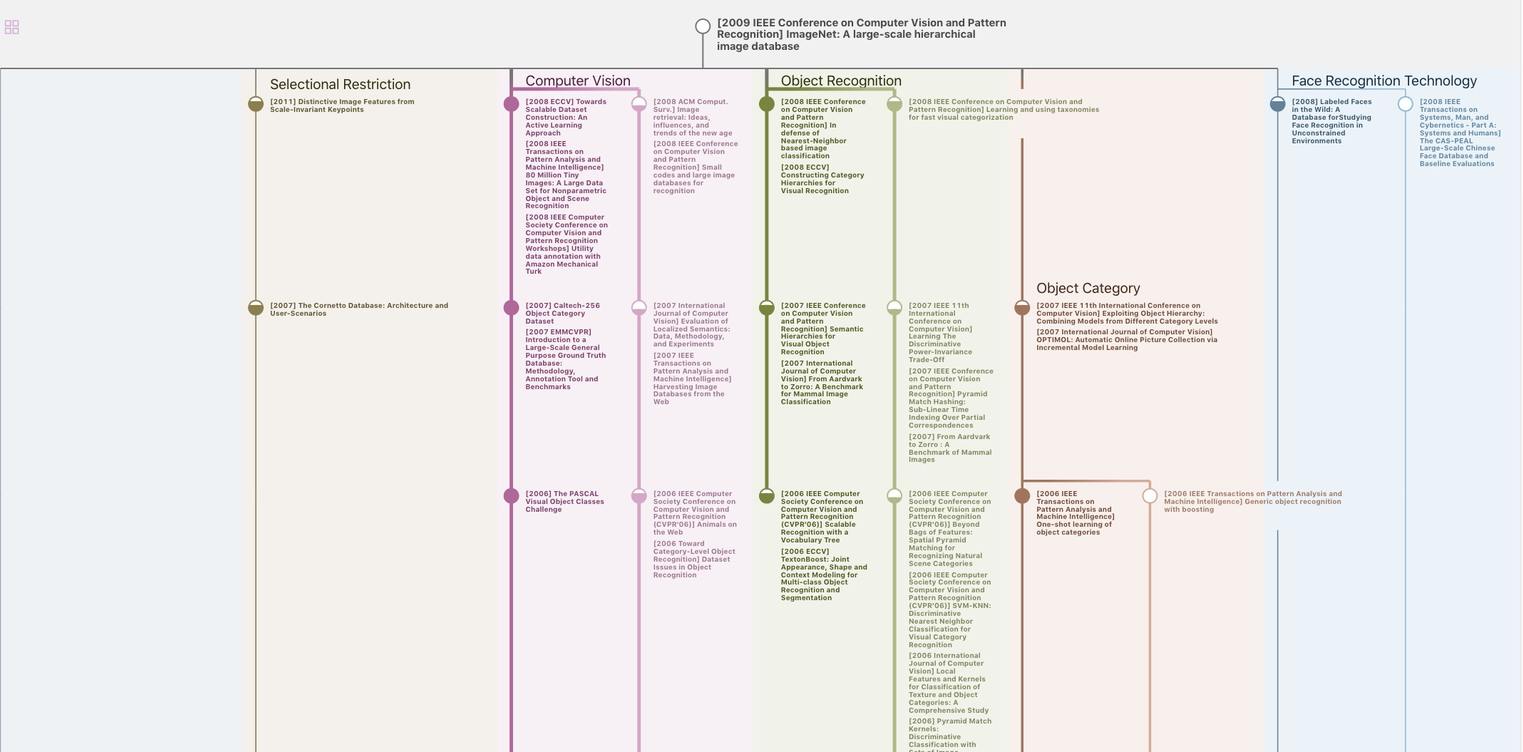
生成溯源树,研究论文发展脉络
Chat Paper
正在生成论文摘要