Double graphs-based discriminant projections for dimensionality reduction
NEURAL COMPUTING & APPLICATIONS(2020)
摘要
Graph embedding plays an important role in dimensionality reduction for processing the high-dimensional data. In graph embedding, its keys are the different kinds of graph constructions that determine the performance of dimensionality reduction. Inspired by this fact, in this article we propose a novel graph embedding method named the double graphs-based discriminant projections (DGDP) by integrating two designed discriminative global graph constructions. The proposed DGDP can well discover the discriminant and geometrical structures of the high-dimensional data through the informative graph constructions. In two global graph constructions, we consider the geometrical distribution of each point on each edge of the graphs to define the adjacent weights with class information. Moreover, in the weight definition of one graph construction, we further strengthen pattern discrimination among all the classes to design the weights of the corresponding adjacent graph. To demonstrate the effectiveness of the proposed DGDP, we experimentally compare it with the state-of-the-art graph embedding methods on several data sets. The experimental results show that the proposed graph embedding method outperforms the competing methods with more power of data representation and pattern discrimination in the embedded subspace.
更多查看译文
关键词
Dimensionality reduction, Graph embedding, Graph construction, Pattern classification
AI 理解论文
溯源树
样例
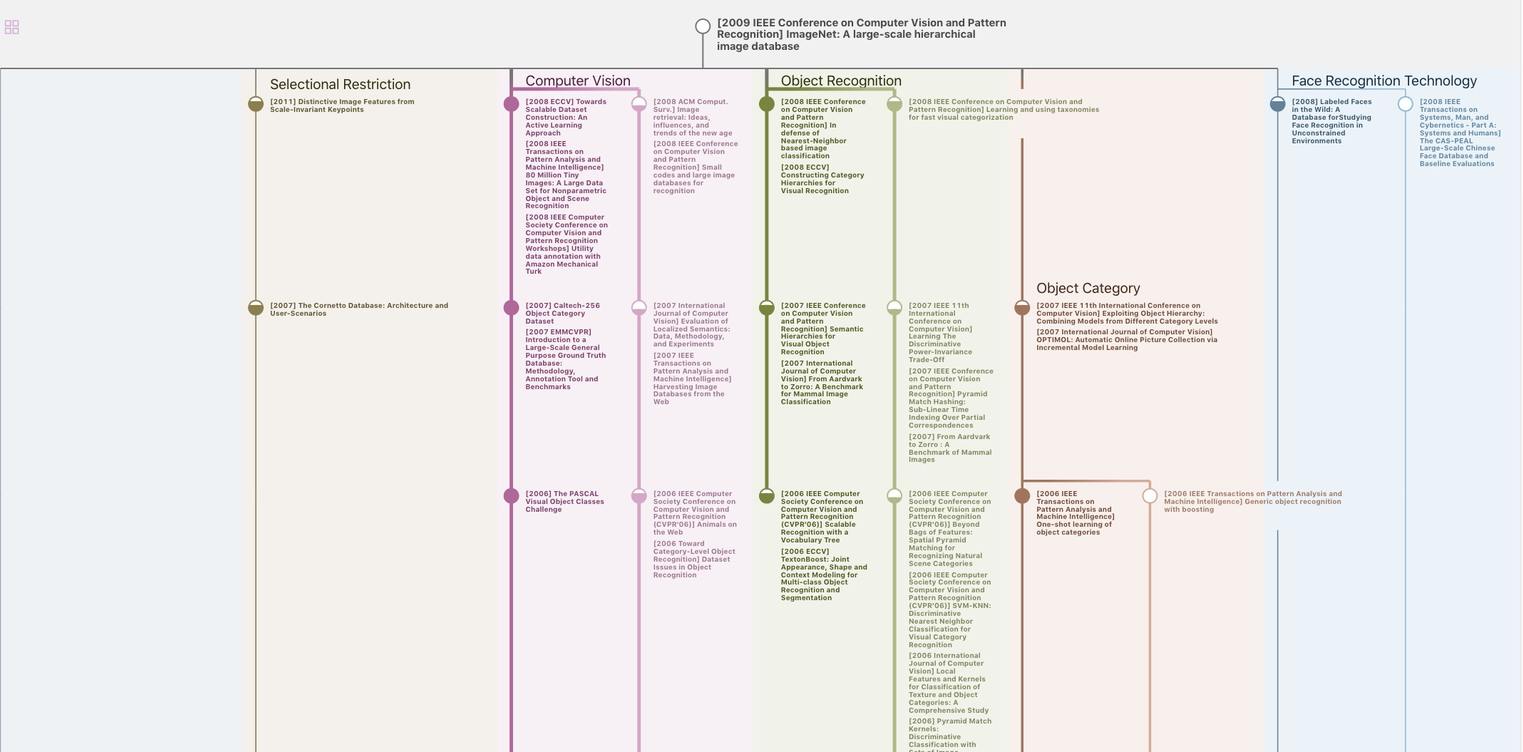
生成溯源树,研究论文发展脉络
Chat Paper
正在生成论文摘要