Mp68-12 predicting intraoperative complications and 30-days morbidity using machine learning techniques for patients undergoing robotic partial nephrectomy
Journal of Urology(2020)
摘要
You have accessJournal of UrologyKidney Cancer: Localized: Surgical Therapy VI (MP68)1 Apr 2020MP68-12 PREDICTING INTRAOPERATIVE COMPLICATIONS AND 30-DAYS MORBIDITY USING MACHINE LEARNING TECHNIQUES FOR PATIENTS UNDERGOING ROBOTIC PARTIAL NEPHRECTOMY Mahendra Bhandari*, Anubhav Reddy Nallabasannagari, Madhu Reddiboina, James Porter, Wooju Jeong, Alexandre Mottrie, Prokar Dasgupta, Ben Challacombe, Ronney Abaza, Koon Ho Rha, Dipen Parekh, Rajesh Ahlawat, Umberto Capitanio, Thyavihally Yuvaraja, Sudhir Rawal, Daniel Moon, Nicolò Buffi's, Ananthakrishnan Sivaraman, Kris Maes, Luz Sáude, Gagan Gautam, Levent Turkeri, Preethi Patil, Mani Menon, and Craig Rogers Mahendra Bhandari*Mahendra Bhandari* More articles by this author , Anubhav Reddy NallabasannagariAnubhav Reddy Nallabasannagari More articles by this author , Madhu ReddiboinaMadhu Reddiboina More articles by this author , James PorterJames Porter More articles by this author , Wooju JeongWooju Jeong More articles by this author , Alexandre MottrieAlexandre Mottrie More articles by this author , Prokar DasguptaProkar Dasgupta More articles by this author , Ben ChallacombeBen Challacombe More articles by this author , Ronney AbazaRonney Abaza More articles by this author , Koon Ho RhaKoon Ho Rha More articles by this author , Dipen ParekhDipen Parekh More articles by this author , Rajesh AhlawatRajesh Ahlawat More articles by this author , Umberto CapitanioUmberto Capitanio More articles by this author , Thyavihally YuvarajaThyavihally Yuvaraja More articles by this author , Sudhir RawalSudhir Rawal More articles by this author , Daniel MoonDaniel Moon More articles by this author , Nicolò Buffi'sNicolò Buffi's More articles by this author , Ananthakrishnan SivaramanAnanthakrishnan Sivaraman More articles by this author , Kris MaesKris Maes More articles by this author , Luz SáudeLuz Sáude More articles by this author , Gagan GautamGagan Gautam More articles by this author , Levent TurkeriLevent Turkeri More articles by this author , Preethi PatilPreethi Patil More articles by this author , Mani MenonMani Menon More articles by this author , and Craig RogersCraig Rogers More articles by this author View All Author Informationhttps://doi.org/10.1097/JU.0000000000000948.012AboutPDF ToolsAdd to favoritesDownload CitationsTrack CitationsPermissionsReprints ShareFacebookLinked InTwitterEmail Abstract INTRODUCTION AND OBJECTIVE: Personalization of a patient risk profile using instruments such as RENAL, PADUA, and MAP scores have limited clinical value. Predictive models built using Machine Learning (ML) could play a significant role in preempting events, timely intervention and improving patient outcomes. Our objective was to predict Intraoperative Complications (IOC) and 30-day Morbidity (M) as a prelude to the prospective deployment of models in a clinical setting at VCQI collaborating institutions to evolve personalized management strategies. METHODS: Predictive models were developed using Logistic Regression, Random Forest, and Neural Networks (Fig 1). Models to predict IOC were trained using patient demographics and preoperative data. In addition to the above data, intraoperative data was used to build models to predict M. Model performance on the test dataset was assessed using Area Under Receiver Operating Curve (AUC-ROC), and Area Under Precision-Recall Curve (PR-AUC). We used bootstrapping to generate confidence intervals for the scores and performed permutation test to assess if the observed difference in AUC-ROC and PR-AUC was significant. RESULTS: Models for predicting IOC were constructed using data from 1690 patients and 38 variables; the best model had AUC-ROC of 0.825 (95% CI 0.717,0.919), and PR-AUC of 0.585 (95% CI 0.394,0.756). Models for predicting M were trained using data from 1455 patients and 59 variables; the best model had AUC-ROC of 0.868 (95% CI 0.827,0.906), and PR-AUC 0.697 (95% CI 0.603,0.781). Dataset with pre-defined variables does not account for the temporal shift in patient characteristics, a key limitation. CONCLUSIONS: These models can be used to predict complications during, and after surgery with good accuracy; paving the way for application in clinical practice to predict, intervene at an opportune time, avert complications and improve patient outcomes (Fig 2). Further validation in a clinical setting would be necessary to establish their clinical value. Source of Funding: RediMinds Inc. © 2020 by American Urological Association Education and Research, Inc.FiguresReferencesRelatedDetails Volume 203Issue Supplement 4April 2020Page: e1039-e1039 Advertisement Copyright & Permissions© 2020 by American Urological Association Education and Research, Inc.MetricsAuthor Information Mahendra Bhandari* More articles by this author Anubhav Reddy Nallabasannagari More articles by this author Madhu Reddiboina More articles by this author James Porter More articles by this author Wooju Jeong More articles by this author Alexandre Mottrie More articles by this author Prokar Dasgupta More articles by this author Ben Challacombe More articles by this author Ronney Abaza More articles by this author Koon Ho Rha More articles by this author Dipen Parekh More articles by this author Rajesh Ahlawat More articles by this author Umberto Capitanio More articles by this author Thyavihally Yuvaraja More articles by this author Sudhir Rawal More articles by this author Daniel Moon More articles by this author Nicolò Buffi's More articles by this author Ananthakrishnan Sivaraman More articles by this author Kris Maes More articles by this author Luz Sáude More articles by this author Gagan Gautam More articles by this author Levent Turkeri More articles by this author Preethi Patil More articles by this author Mani Menon More articles by this author Craig Rogers More articles by this author Expand All Advertisement PDF downloadLoading ...
更多查看译文
AI 理解论文
溯源树
样例
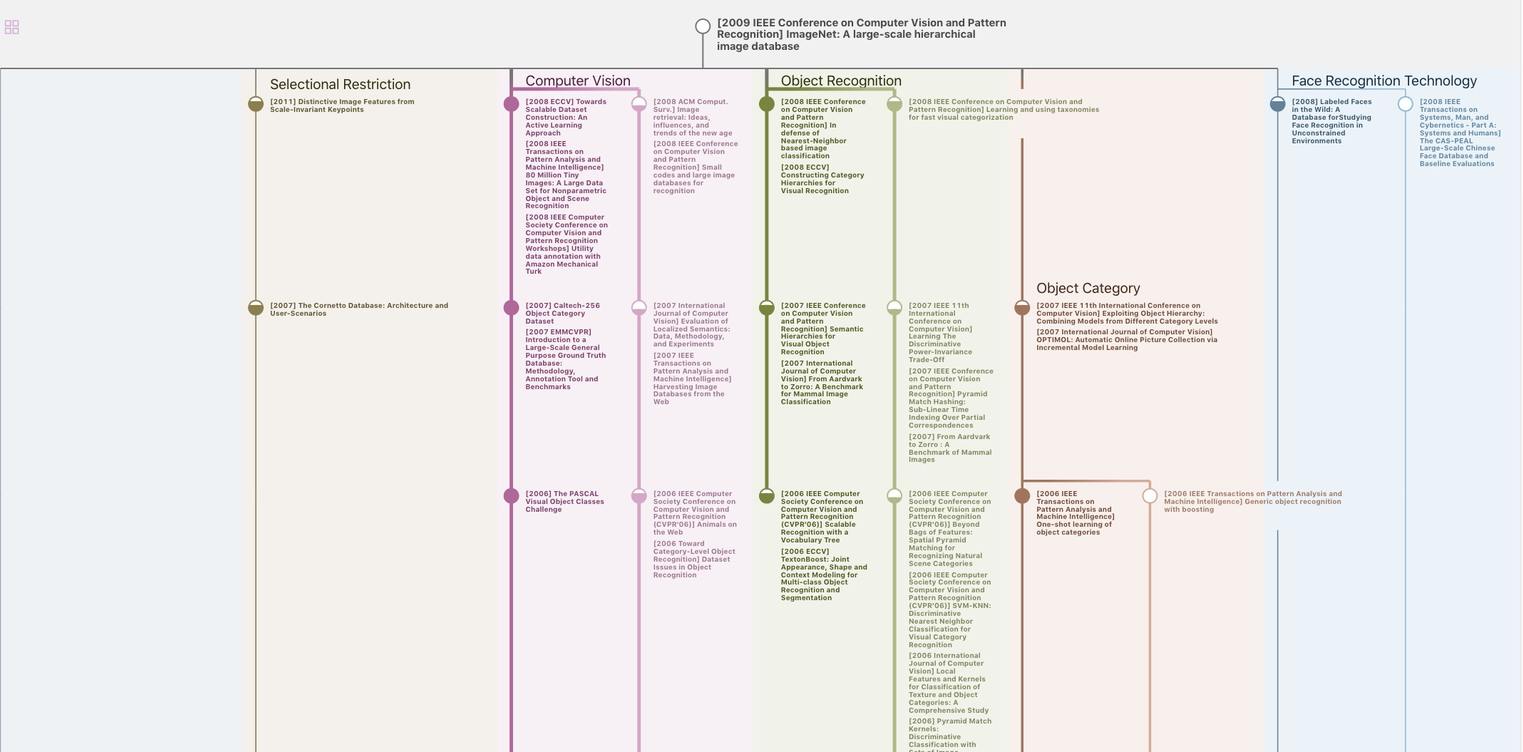
生成溯源树,研究论文发展脉络
Chat Paper
正在生成论文摘要