Multimodal Analytics To Understand Self-Regulation Process Of Cognitive And Behavioral Strategies In Real-World Learning
IEICE TRANSACTIONS ON INFORMATION AND SYSTEMS(2020)
摘要
Recent studies suggest that learning "how to learn" is important because learners must be self-regulated to take more responsibility for their own learning processes, meta-cognitive control, and other generative learning thoughts and behaviors. The mechanism that enables a learner to self-regulate his/her learning strategies has been actively studied in classroom settings, but has seldom been studied in the area of real-world learning in out-of-school settings (e.g., environmental learning in nature). A feature of real-world learning is that a learner's cognition of the world is updated by his/her behavior to investigate the world, and vice versa. This paper models the mechanism of real-world learning for executing and selfregulating a learner's cognitive and behavioral strategies to self-organize his/her internal knowledge space. Furthermore, this paper proposes multimodal analytics to integrate heterogeneous data resources of the cognitive and behavioral features of real-world learning, to structure and archive the time series of strategies occurring through learner-environment interactions, and to assess how learning should be self-regulated for better understanding of the world. Our analysis showed that (1) intellectual achievements are built by self-regulating learning to chain the execution of cognitive and behavioral strategies, and (2) a clue to predict learning outcomes in the world is analyzing the quantity and frequency of strategies that a learner uses and self-regulates. Assessment based on these findings can encourage a learner to reflect and improve his/her way of learning in the world.
更多查看译文
关键词
real-world learning, learning analytics, self-regulation, multi-modal behavior sensing, knowledge representation
AI 理解论文
溯源树
样例
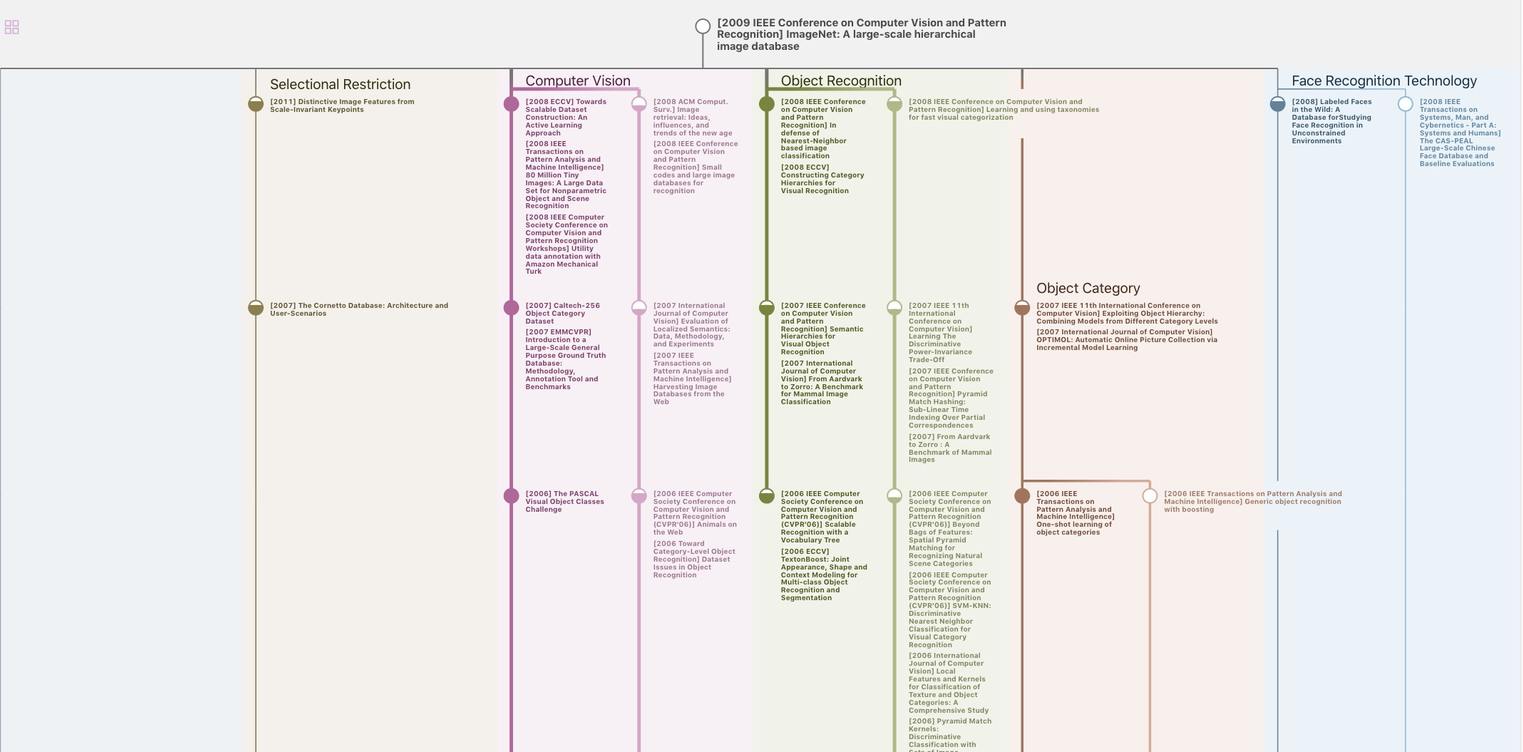
生成溯源树,研究论文发展脉络
Chat Paper
正在生成论文摘要