L0-norm Constrained Autoencoders for Unsupervised Outlier Detection.
PAKDD (2)(2020)
摘要
Unsupervised outlier detection is commonly performed using reconstruction-based methods such as Principal Component Analysis. A recent problem in this field is the learning of low-dimensional nonlinear manifolds under L0-norm constraints for error terms. Despite significant efforts, no method that consistently treats such features exists. We propose a novel unsupervised outlier detection method, L0-norm Constrained Autoencoders (L0-AE), based on an autoencoder-based detector with L0-norm constraints for error terms. Unlike existing methods, the proposed optimization procedure of L0-AE provably guarantees the convergence of the objective function under a mild condition, while neither the relaxation of the L0-norm constraint nor the linearity of the latent manifold is enforced. Experimental results show that the proposed L0-AE is more robust and accurate than other reconstruction-based methods, as well as conventional methods such as Isolation Forest.
更多查看译文
关键词
outlier detection
AI 理解论文
溯源树
样例
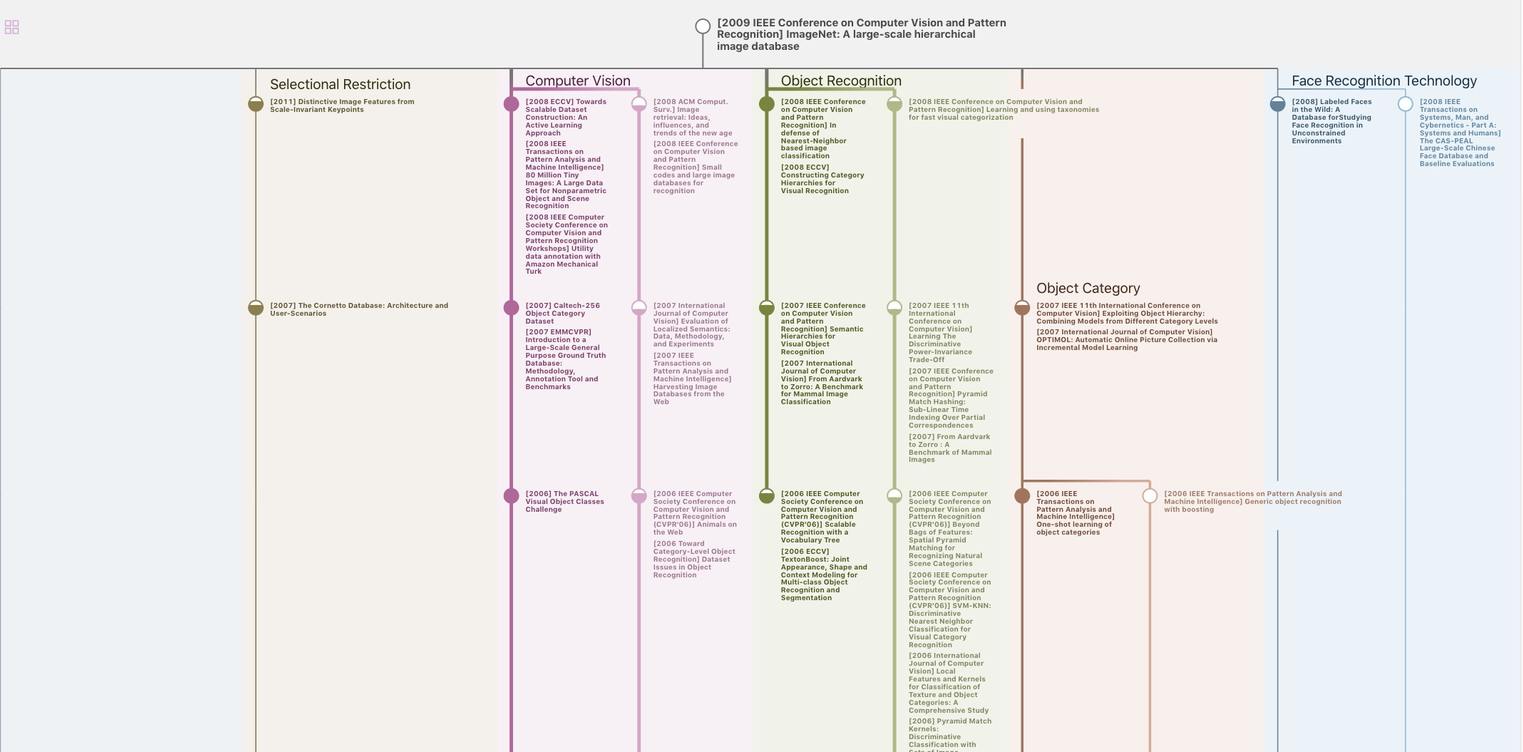
生成溯源树,研究论文发展脉络
Chat Paper
正在生成论文摘要