Universal Embeddings for Spatio-Temporal Tagging of Self-Driving Logs.
CoRL(2020)
摘要
In this paper, we tackle the problem of spatio-temporal tagging of self-driving scenes from raw sensor data. Our approach learns a universal embedding for all tags, enabling efficient tagging of many attributes and faster learning of new attributes with limited data. Importantly, the embedding is spatio-temporally aware, allowing the model to naturally output spatio-temporal tag values. Values can then be pooled over arbitrary regions, in order to, for example, compute the pedestrian density in front of the SDV, or determine if a car is blocking another car at a 4-way intersection. We demonstrate the effectiveness of our approach on a new large scale self-driving dataset, SDVScenes, containing 15 attributes relating to vehicle and pedestrian density, the actions of each actor, the speed of each actor, interactions between actors, and the topology of the road map.
更多查看译文
关键词
spatio-temporal,self-driving
AI 理解论文
溯源树
样例
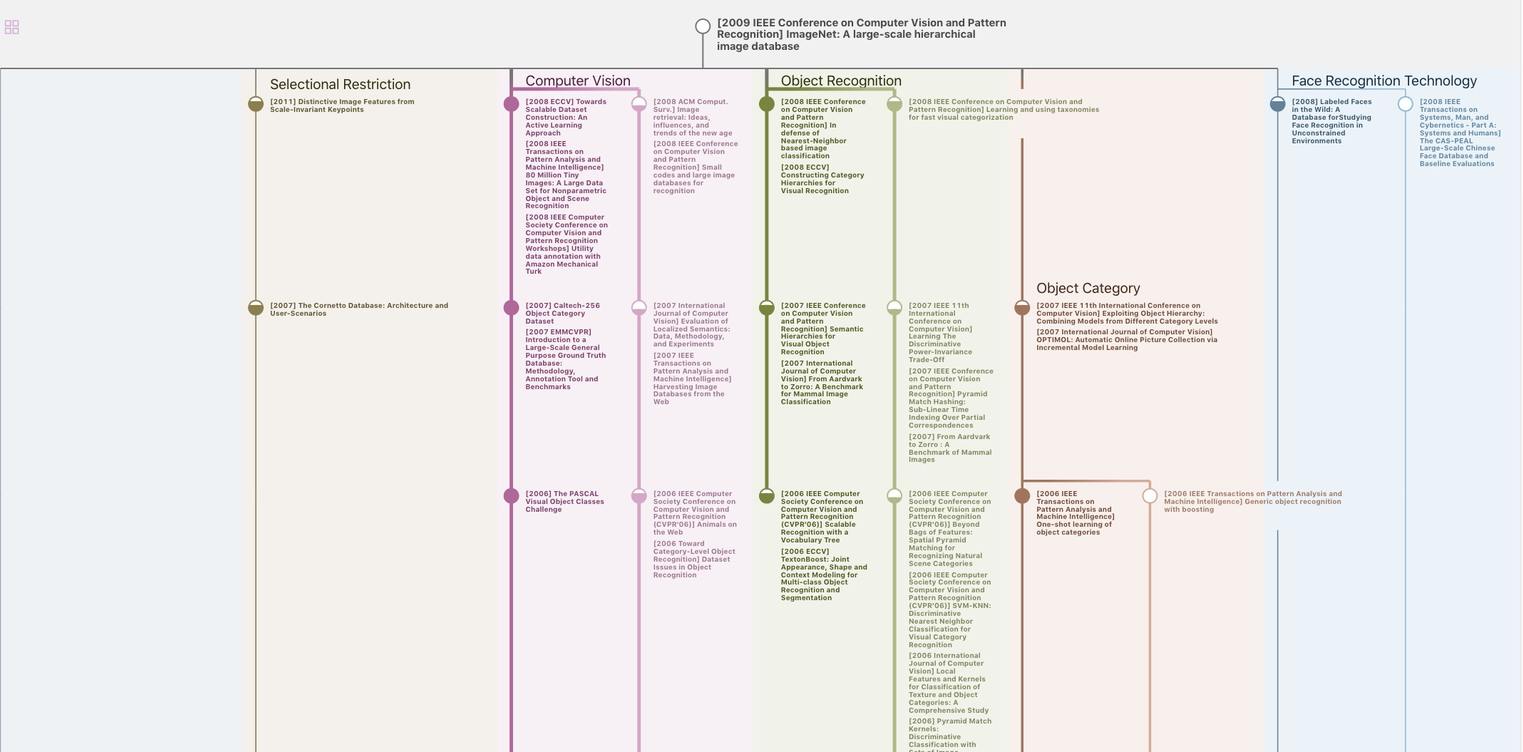
生成溯源树,研究论文发展脉络
Chat Paper
正在生成论文摘要