Dynamic Plane Convolutional Occupancy Networks
2021 IEEE WINTER CONFERENCE ON APPLICATIONS OF COMPUTER VISION (WACV 2021)(2021)
摘要
Learning-based 3D reconstruction using implicit neural representations has shown promising progress not only at the object level but also in more complicated scenes. In this paper, we propose Dynamic Plane Convolutional Occupancy Networks, a novel implicit representation pushing further the quality of 3D surface reconstruction. The input noisy point clouds are encoded into per-point features that are projected onto multiple 2D dynamic planes. A fully-connected network learns to predict plane parameters that best describe the shapes of objects or scenes. To further exploit translational equivariance, convolutional neural networks are applied to process the plane features. Our method shows superior performance in surface reconstruction from unoriented point clouds in ShapeNet as well as an indoor scene dataset. Moreover, we also provide interesting observations on the distribution of learned dynamic planes.
更多查看译文
关键词
plane features,surface reconstruction,implicit neural representations,implicit representation,multiple 2D dynamic planes,plane parameters,convolutional neural networks,noisy point clouds,dynamic plane convolutional occupancy networks,ShapeNet,translational equivariance
AI 理解论文
溯源树
样例
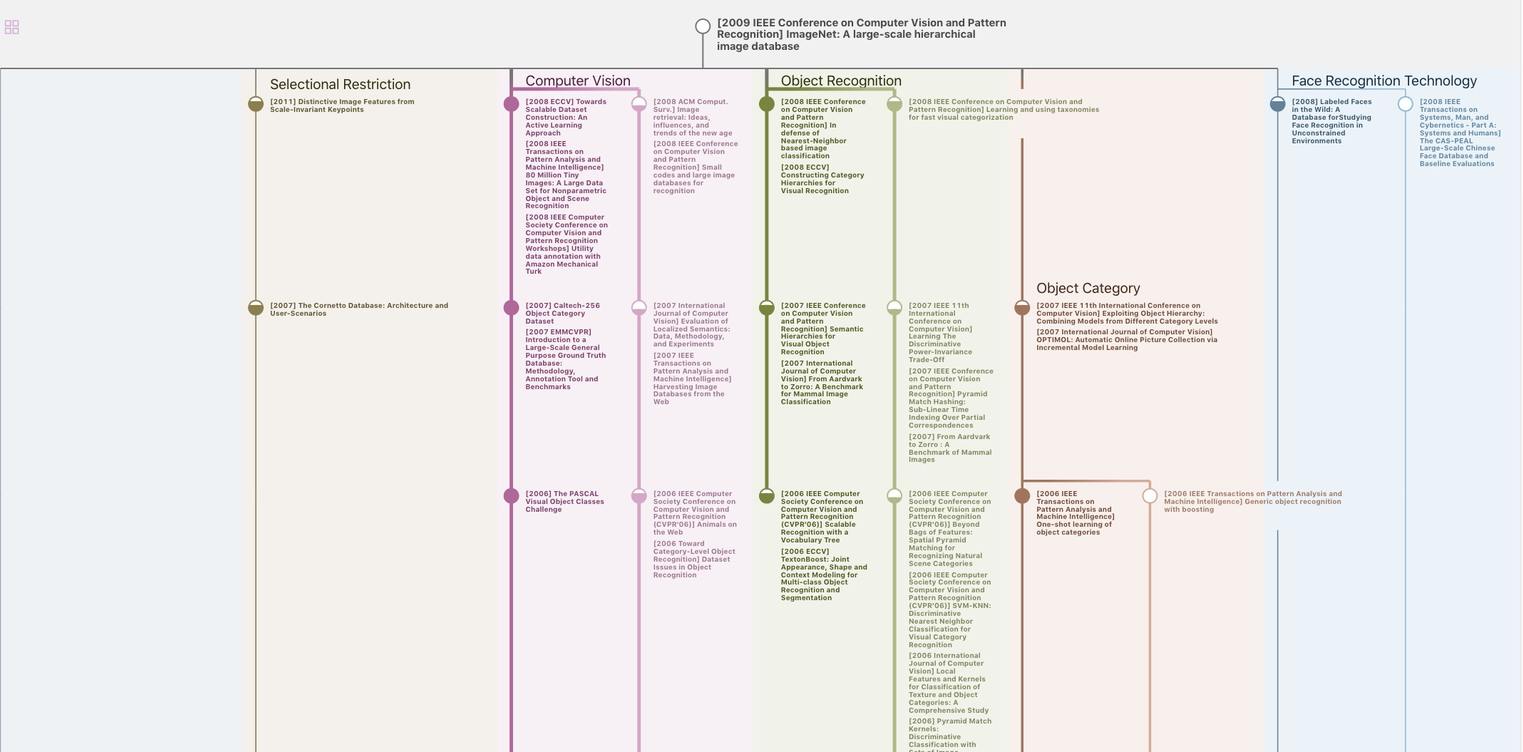
生成溯源树,研究论文发展脉络
Chat Paper
正在生成论文摘要