Learning User Representations with Hypercuboids for Recommender Systems
WSDM(2021)
摘要
ABSTRACTModeling user interests is crucial in real-world recommender systems. In this paper, we present a new user interest representation model for personalized recommendation. Specifically, the key novelty behind our model is that it explicitly models user interests as a hypercuboid instead of a point in the space. In our approach, the recommendation score is learned by calculating a compositional distance between the user hypercuboid and the item. This helps to alleviate the potential geometric inflexibility of existing collaborative filtering approaches, enabling a greater extent of modeling capability. Furthermore, we present two variants of hypercuboids to enhance the capability in capturing the diversities of user interests. A neural architecture is also proposed to facilitate user hypercuboid learning by capturing the activity sequences (e.g., buy and rate) of users. We demonstrate the effectiveness of our proposed model via extensive experiments on both public and commercial datasets. Empirical results show that our approach achieves very promising results, outperforming existing state-of-the-art.
更多查看译文
关键词
Recommender Systems,Hypercuboids,User Representation
AI 理解论文
溯源树
样例
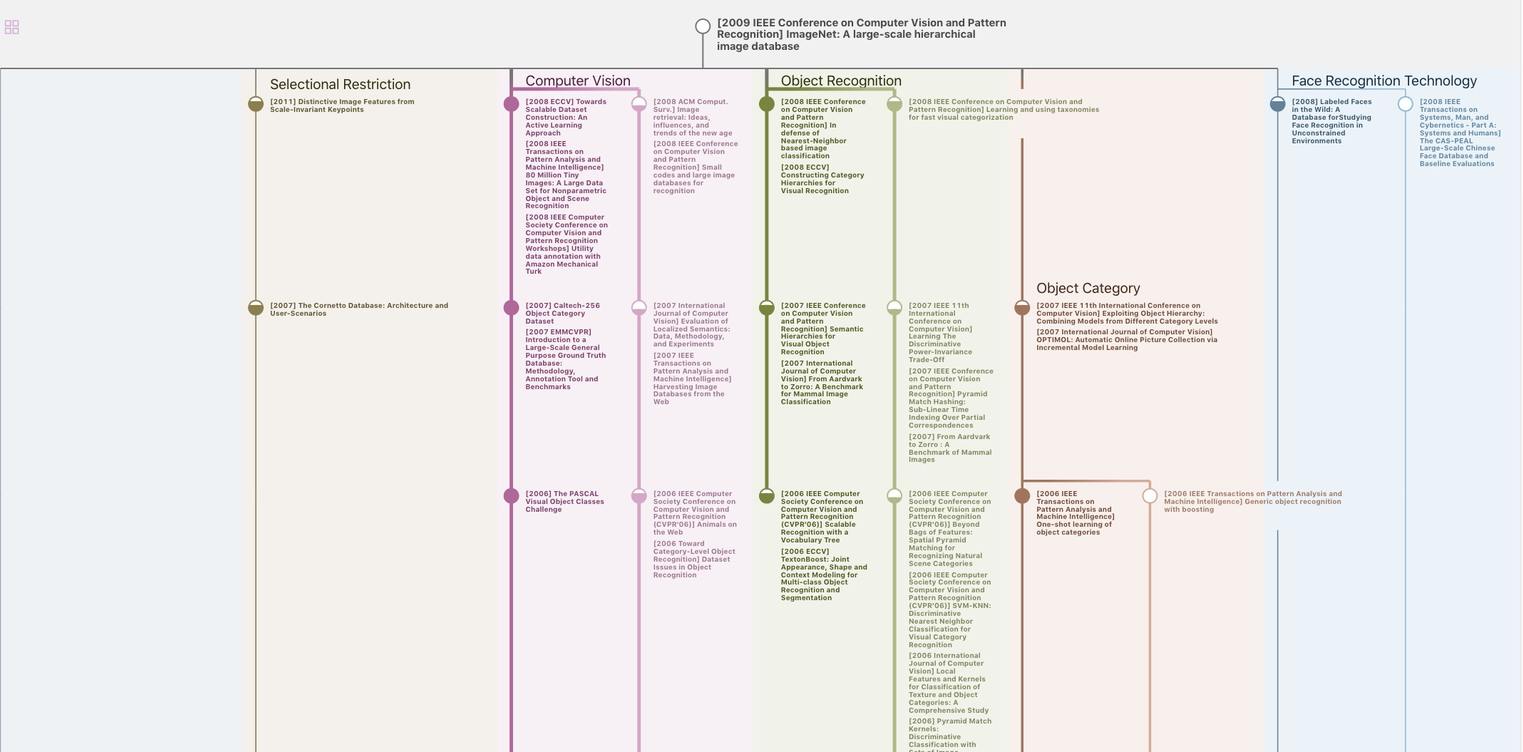
生成溯源树,研究论文发展脉络
Chat Paper
正在生成论文摘要