Adaptive Community Search in Dynamic Networks
2020 IEEE INTERNATIONAL CONFERENCE ON BIG DATA (BIG DATA)(2020)
摘要
Community search is a well-studied problem which, given a static graph and a query set of vertices, requires to find a cohesive (or dense) subgraph containing the query vertices. In this paper we study the problem of community search in temporal dynamic networks. We adapt to the temporal setting the notion of \emph{network inefficiency} which is based on the pairwise shortest-path distance among all the vertices in a solution. For this purpose we define the notion of \emph{shortest-fastest-path distance}: a linear combination of the temporal and spatial dimensions governed by a user-defined parameter. We thus define the \textsc{Minimum Temporal-Inefficiency Subgraph} problem and show that it is \NPhard. We develop an algorithm which exploits a careful transformation of the temporal network to a static directed and weighted graph, and some recent approximation algorithm for finding the minimum Directed Steiner Tree. We finally generalize our framework to the streaming setting in which new snapshots of the temporal graph keep arriving continuously and our goal is to produce a community search solution for the temporal graph corresponding to a sliding time window.
更多查看译文
关键词
dynamic,adaptive,networks,community,search
AI 理解论文
溯源树
样例
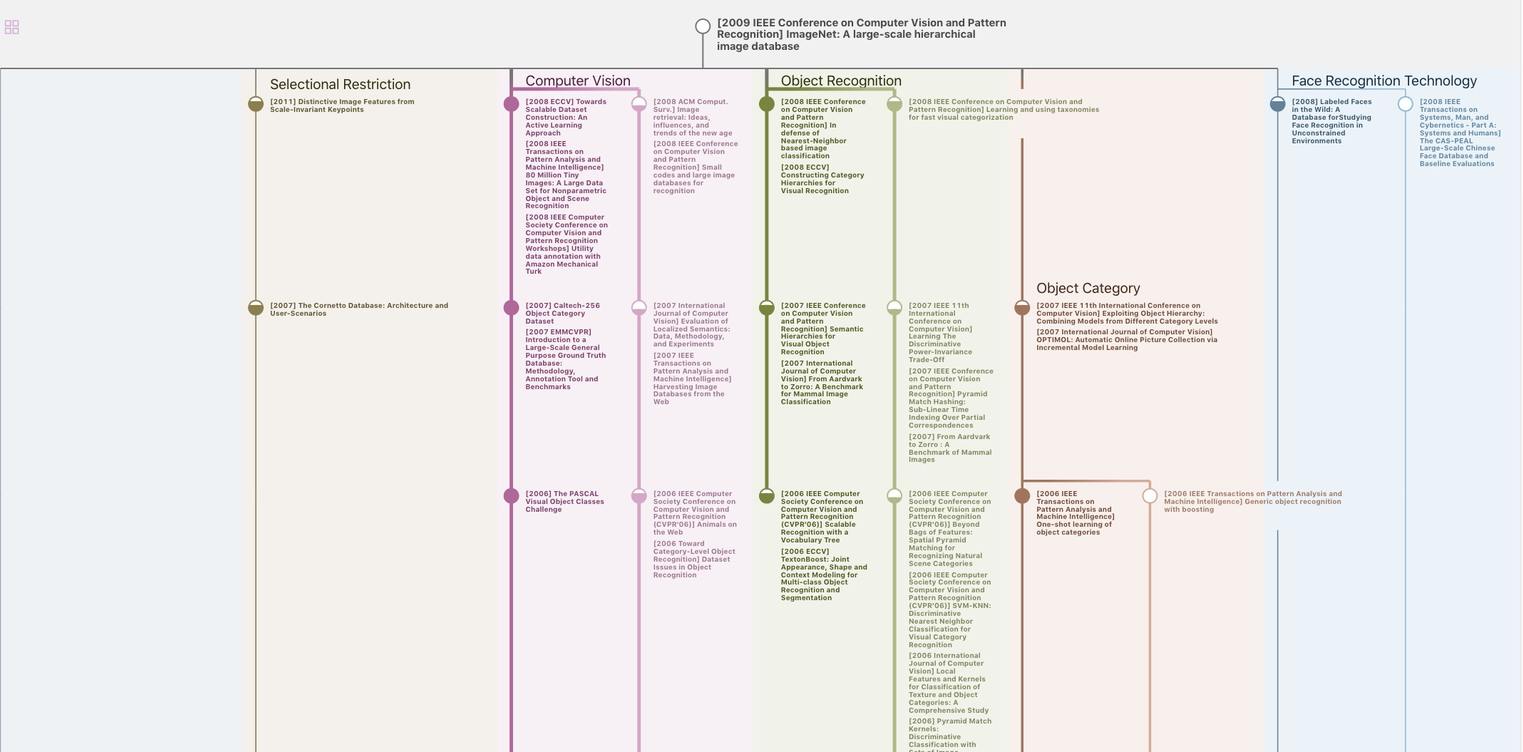
生成溯源树,研究论文发展脉络
Chat Paper
正在生成论文摘要