Topological regularization via persistence-sensitive optimization
Computational Geometry(2024)
摘要
Optimization, a key tool in machine learning and statistics, relies on regularization to reduce overfitting. Traditional regularization methods control a norm of the solution to ensure its smoothness. Recently, topological methods have emerged as a way to provide a more precise and expressive control over the solution, relying on persistent homology to quantify and reduce its roughness. All such existing techniques back-propagate gradients through the persistence diagram, which is a summary of the topological features of a function. Their downside is that they provide information only at the critical points of the function. We propose a method that instead builds on persistence-sensitive simplification and translates the required changes to the persistence diagram into changes on large subsets of the domain, including both critical and regular points. This approach enables a faster and more precise topological regularization, the benefits of which we illustrate with experimental evidence.
更多查看译文
关键词
optimization,persistence-sensitive
AI 理解论文
溯源树
样例
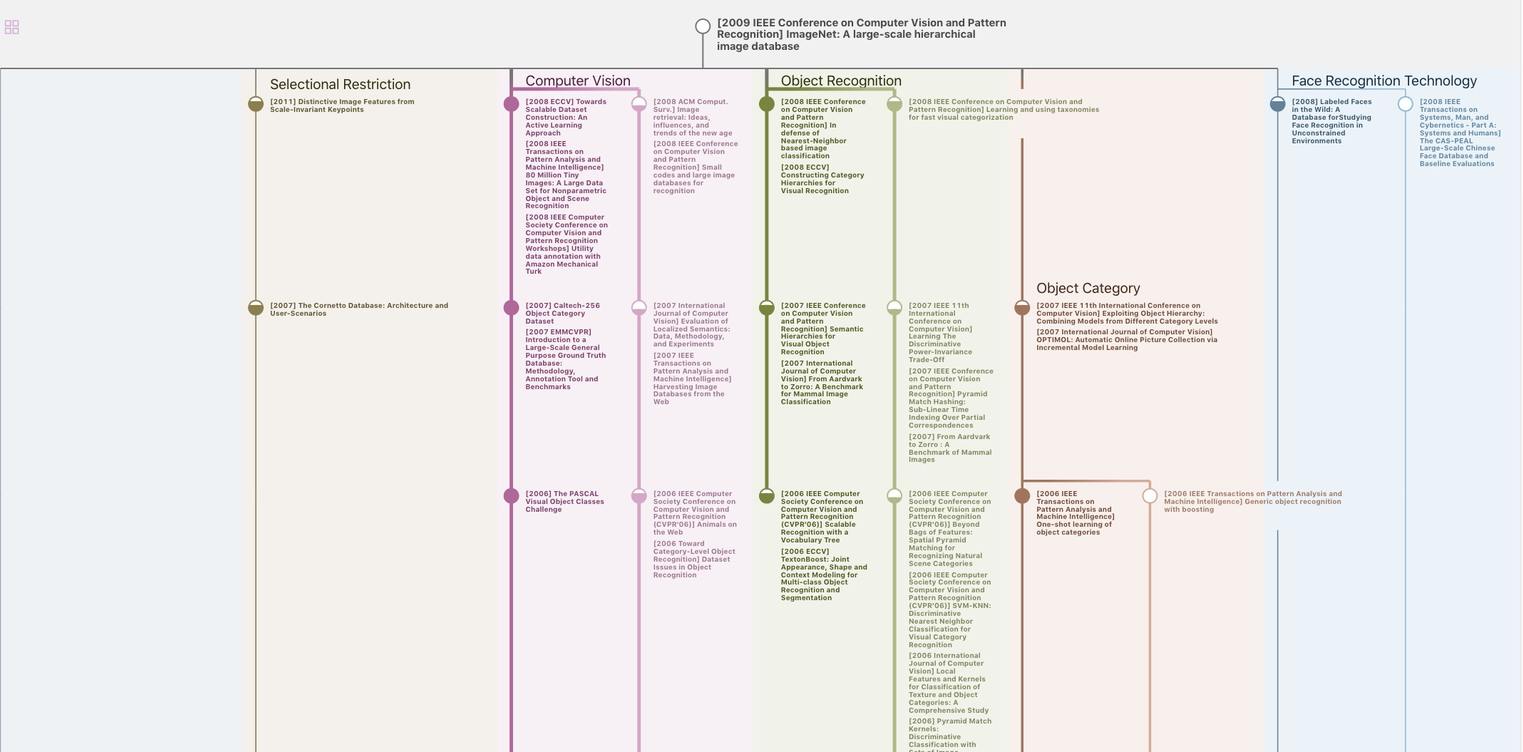
生成溯源树,研究论文发展脉络
Chat Paper
正在生成论文摘要