An Analysis of Dataset Overlap on Winograd-Style Tasks
international conference on computational linguistics(2020)
摘要
The Winograd Schema Challenge (WSC) and variants inspired by it have become important benchmarks for common-sense reasoning (CSR). Model performance on the WSC has quickly progressed from chance-level to near-human using neural language models trained on massive corpora. In this paper, we analyze the effects of varying degrees of overlap between these training corpora and the test instances in WSC-style tasks. We find that a large number of test instances overlap considerably with the corpora on which state-of-the-art models are (pre)trained, and that a significant drop in classification accuracy occurs when we evaluate models on instances with minimal overlap. Based on these results, we develop the KnowRef-60K dataset, which consists of over 60k pronoun disambiguation problems scraped from web data. KnowRef-60K is the largest corpus to date for WSC-style common-sense reasoning and exhibits a significantly lower proportion of overlaps with current pretraining corpora.
更多查看译文
关键词
dataset overlap,winograd-style
AI 理解论文
溯源树
样例
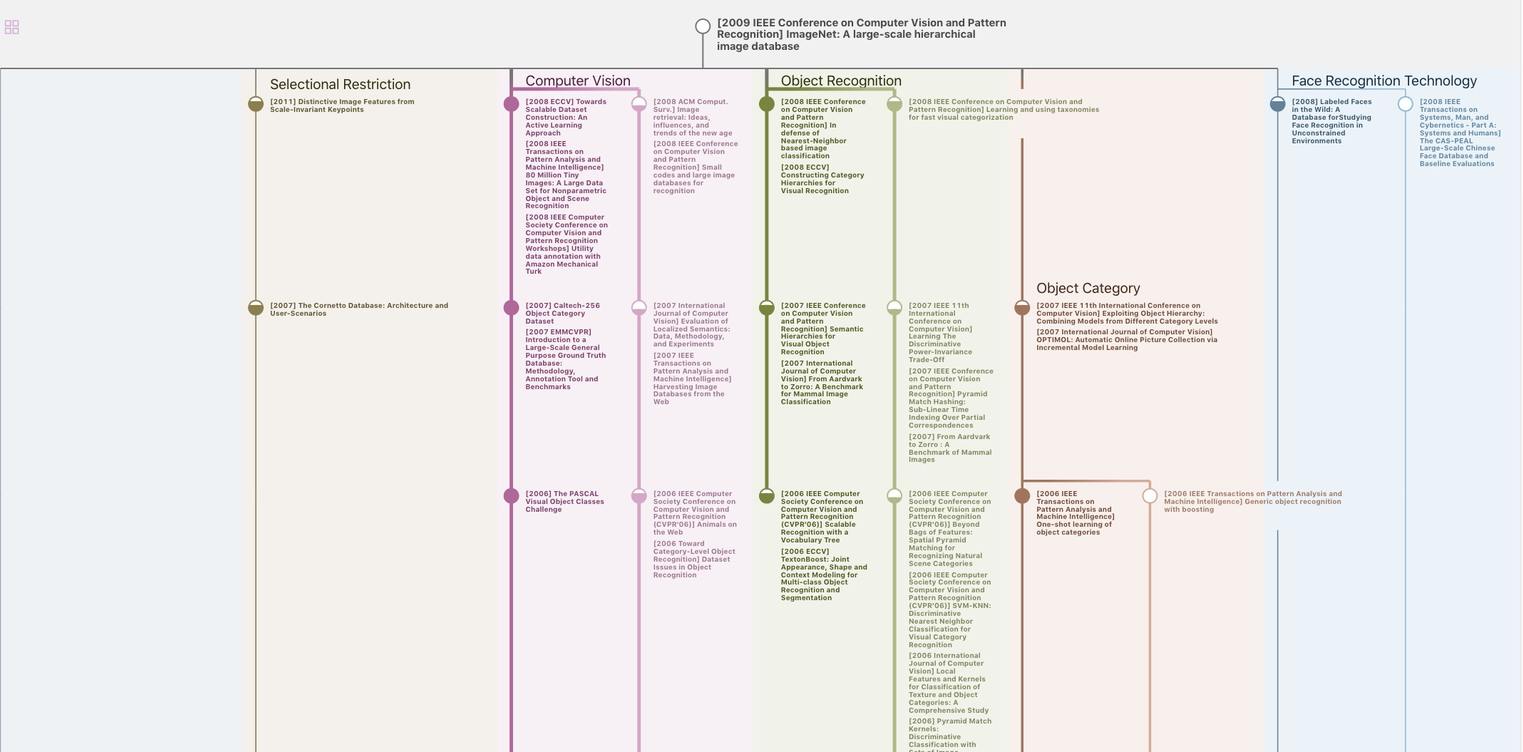
生成溯源树,研究论文发展脉络
Chat Paper
正在生成论文摘要