From eye-blinks to state construction: Diagnostic benchmarks for online representation learning
arxiv(2023)
摘要
We present three new diagnostic prediction problems inspired by classical-conditioning experiments to facilitate research in online prediction learning. Experiments in classical conditioning show that animals such as rabbits, pigeons, and dogs can make long temporal associations that enable multi-step prediction. To replicate this remarkable ability, an agent must construct an internal state representation that summarizes its interaction history. Recurrent neural networks can automatically construct state and learn temporal associations. However, the current training methods are prohibitively expensive for online prediction-continual learning on every time step-which is the focus of this paper. Our proposed problems test the learning capabilities that animals readily exhibit and highlight the limitations of the current recurrent learning methods. While the proposed problems are nontrivial, they are still amenable to extensive testing and analysis in the small-compute regime, thereby enabling researchers to study issues in isolation, ultimately accelerating progress towards scalable online representation learning methods.
更多查看译文
关键词
State construction,classical conditioning,diagnostic benchmarks,reinforcement learning
AI 理解论文
溯源树
样例
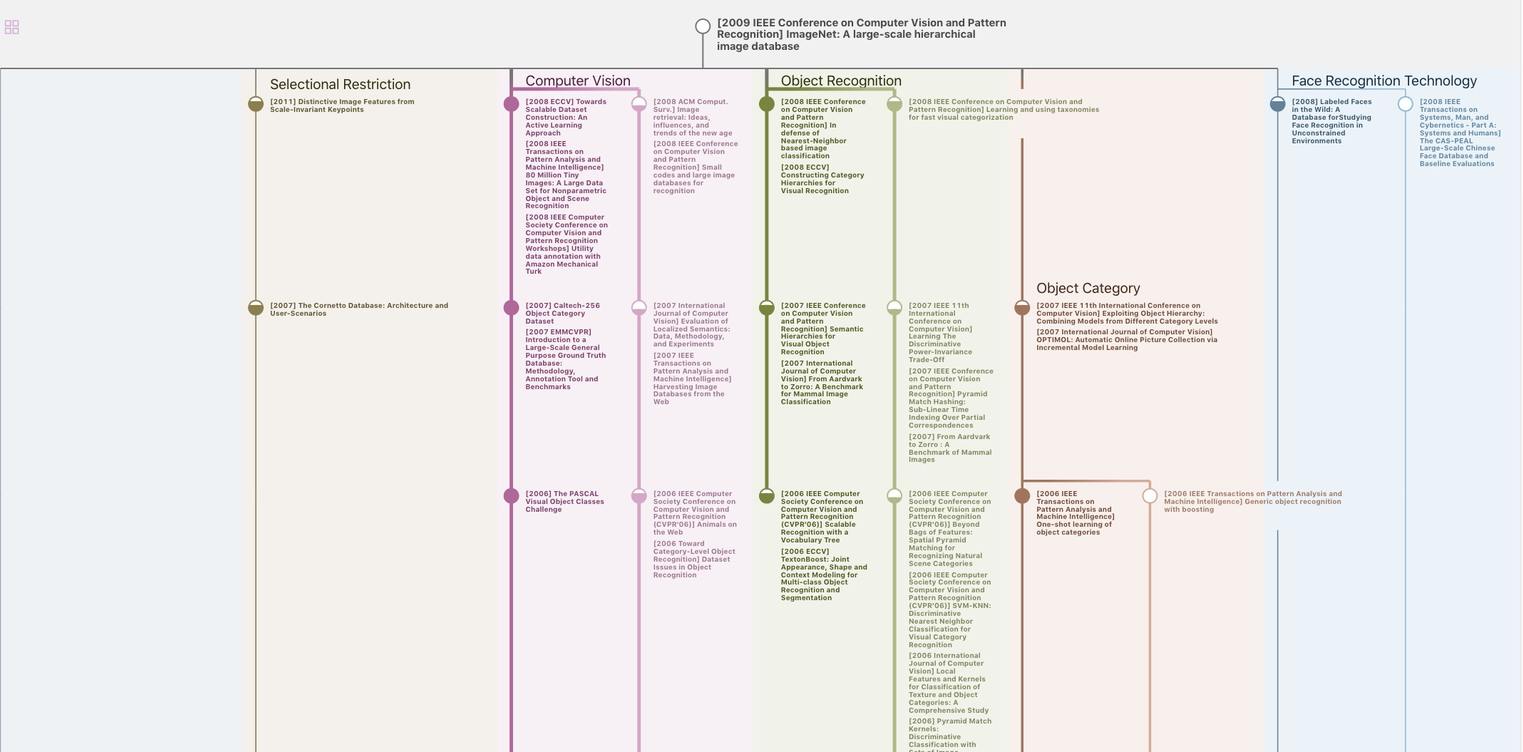
生成溯源树,研究论文发展脉络
Chat Paper
正在生成论文摘要