Fast Hybrid Cascade for Voxel-based 3D Object Classification
arxiv(2020)
摘要
Voxel-based 3D object classification has been frequently studied in recent years. The previous methods often directly convert the classic 2D convolution into a 3D form applied to an object with binary voxel representation. In this paper, we investigate the reason why binary voxel representation is not very suitable for 3D convolution and how to simultaneously improve the performance both in accuracy and speed. We show that by giving each voxel a signed distance value, the accuracy will gain about 30% promotion compared with binary voxel representation using a two-layer fully connected network. We then propose a fast fully connected and convolution hybrid cascade network for voxel-based 3D object classification. This threestage cascade network can divide 3D models into three categories: easy, moderate and hard. Consequently, the mean inference time (0.3ms) can speedup about 5x and 2x compared with the state-of-the-art point cloud and voxel based methods respectively, while achieving the highest accuracy in the latter category of methods (92%). Experiments with ModelNet andMNIST verify the performance of the proposed hybrid cascade network.
更多查看译文
关键词
3d object classification,voxel-based
AI 理解论文
溯源树
样例
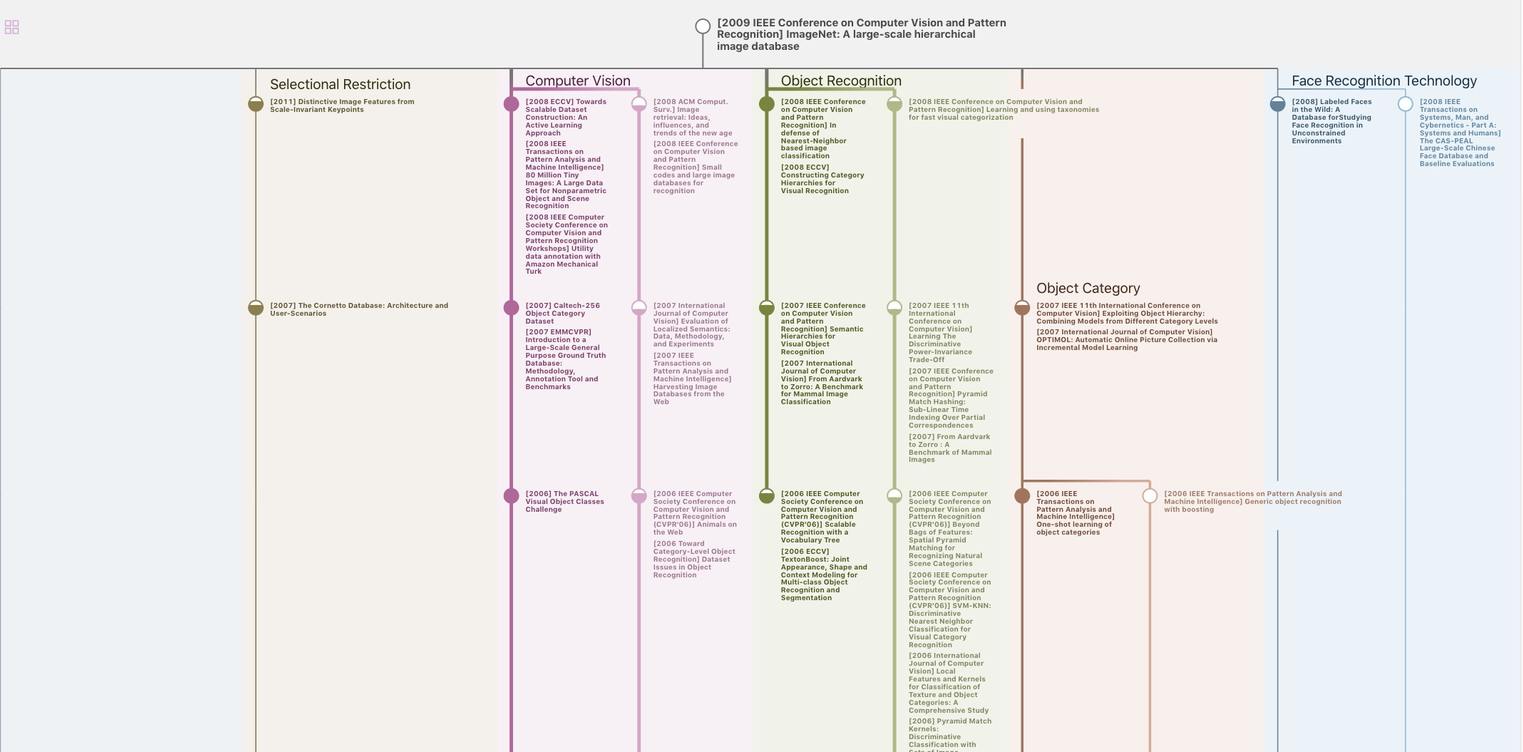
生成溯源树,研究论文发展脉络
Chat Paper
正在生成论文摘要