Facegan: Facial Attribute Controllable Reenactment Gan
2021 IEEE WINTER CONFERENCE ON APPLICATIONS OF COMPUTER VISION (WACV 2021)(2021)
摘要
The face reenactment is a popular facial animation method where the person's identity is taken from the source image and the facial motion from the driving image. Recent works have demonstrated high quality results by combining the facial landmark based motion representations with the generative adversarial networks. These models perform best if the source and driving images depict the same person or if the facial structures are otherwise very similar. However, if the identity differs, the driving facial structures leak to the output distorting the reenactment result. We propose a novel Facial Attribute Controllable rEenactment GAN (FACEGAN), which transfers the facial motion from the driving face via the Action Unit (AU) representation. Unlike facial landmarks, the AUs are independent of the facial structure preventing the identity leak. Moreover, AUs provide a human interpretable way to control the reenactment. FACEGAN processes background and face regions separately for optimized output quality. The extensive quantitative and qualitative comparisons show a clear improvement over the state-of-the-art in a single source reenactment task. The results are best illustrated in the reenactment video provided in the supplementary material. The source code will be made available upon publication of the paper.
更多查看译文
关键词
FACEGAN,face reenactment,popular facial animation method,person,source image,facial motion,driving image,high quality results,facial landmark,motion representations,generative adversarial networks,driving images,facial structure,identity differs,driving facial structures,reenactment result,novel Facial Attribute Controllable rEenactment GAN,driving face,identity leak,face regions,optimized output quality,single source reenactment task,reenactment video,source code
AI 理解论文
溯源树
样例
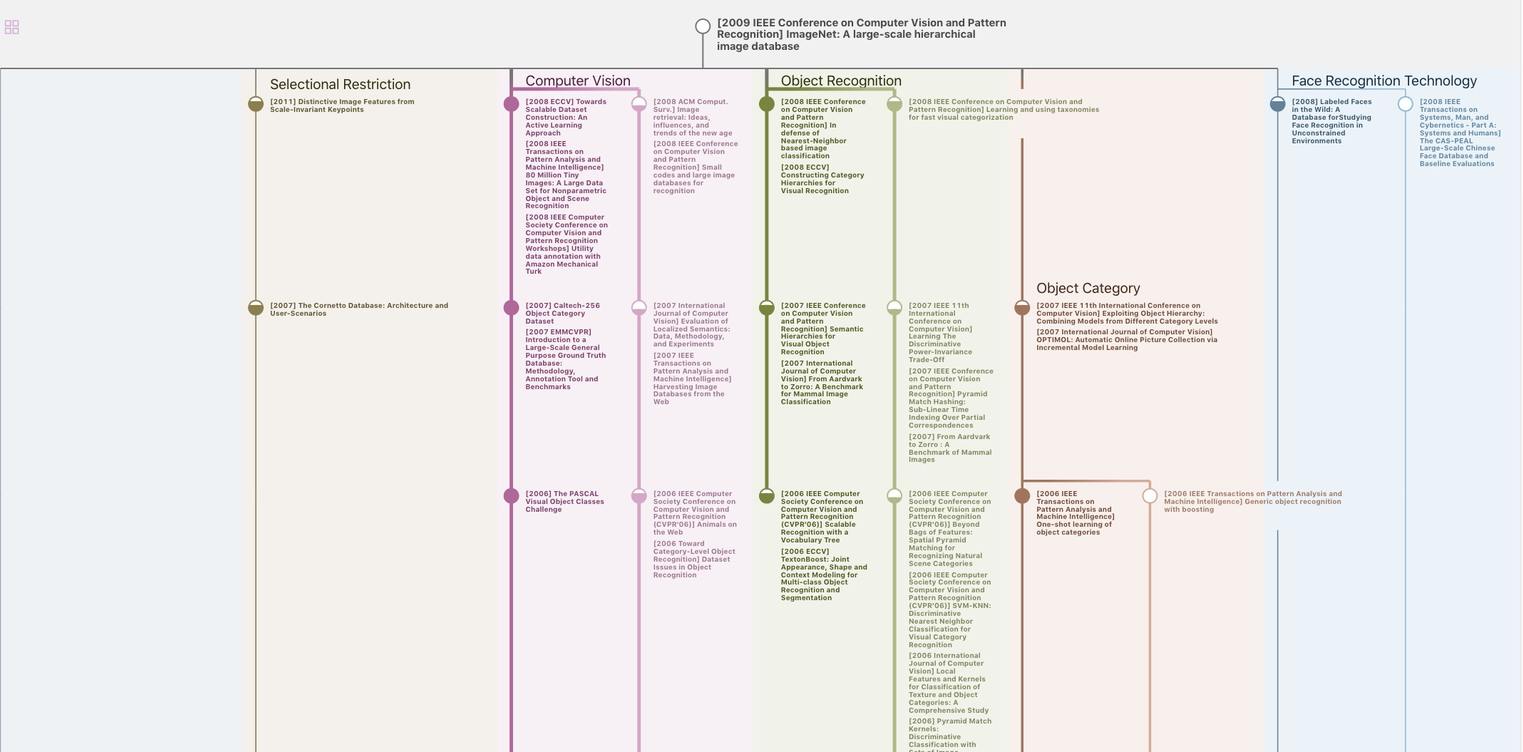
生成溯源树,研究论文发展脉络
Chat Paper
正在生成论文摘要