Leveraging Forward Model Prediction Error for Learning Control
2021 IEEE INTERNATIONAL CONFERENCE ON ROBOTICS AND AUTOMATION (ICRA 2021)(2021)
摘要
Learning for model based control can be sample-efficient and generalize well, however successfully learning models and controllers that represent the problem at hand can be challenging for complex tasks. Using inaccurate models for learning can lead to sub-optimal solutions that are unlikely to perform well in practice. In this work, we present a learning approach which iterates between model learning and data collection and leverages forward model prediction error for learning control. We show how using the controller's prediction as input to a forward model can create a differentiable connection between the controller and the model, allowing us to formulate a loss in the state space. This lets us include forward model prediction error during controller learning and we show that this creates a loss objective that significantly improves learning on different motor control tasks. We provide empirical and theoretical results that show, the benefits of our method and present evaluations in simulation for learning control on a 7 DoF manipulator and an underactuated 12 DoF quadruped. We show that our approach successfully learns controllers for challenging motor control tasks involving contact switching.
更多查看译文
关键词
controller learning,motor control tasks,learning control,forward model prediction error,model based control,data collection,7 DoF manipulator
AI 理解论文
溯源树
样例
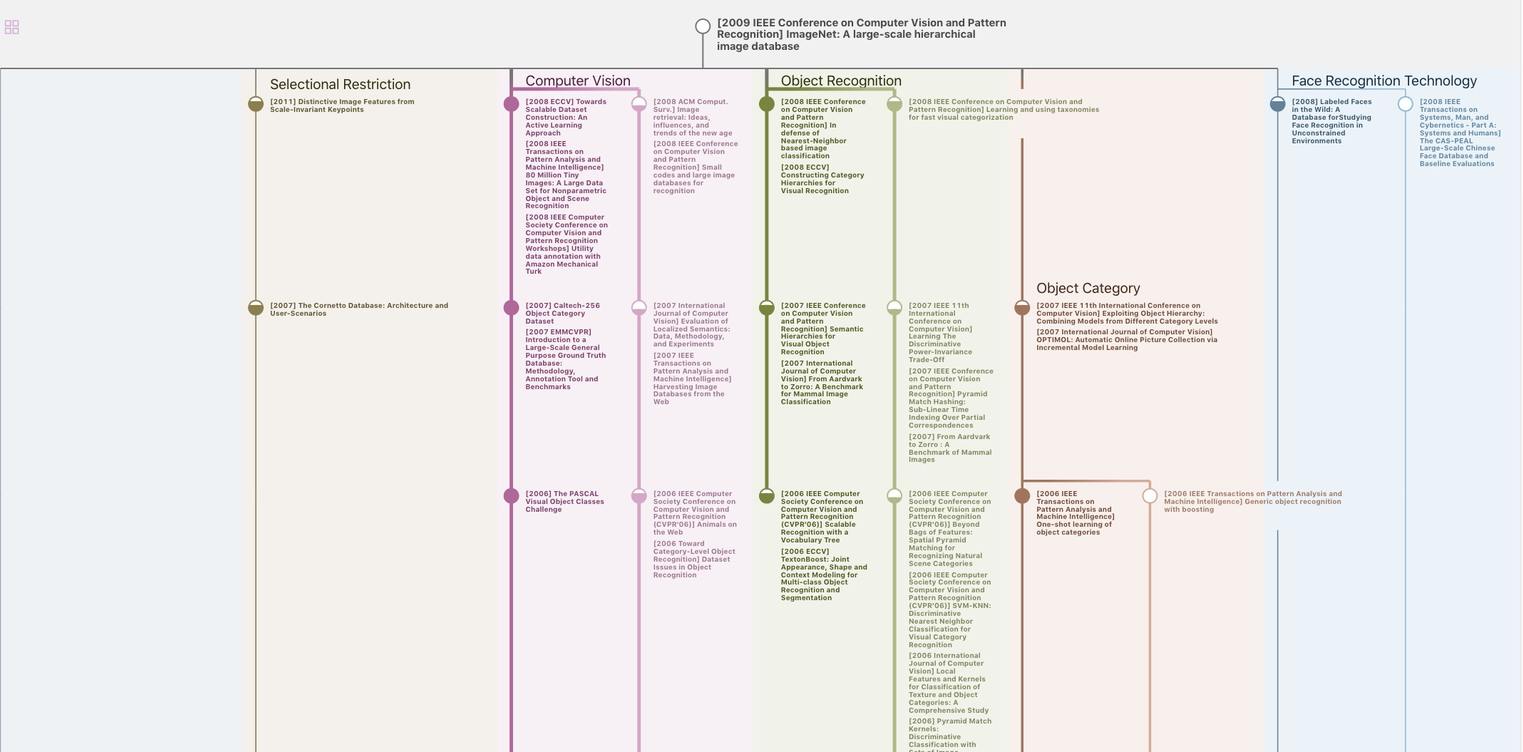
生成溯源树,研究论文发展脉络
Chat Paper
正在生成论文摘要