DEVELOP A MACHINE LEARNING MODEL AND ALGORITHM BASED ON SMART SYSTEM OF DISEASE MANAGEMENT (SSDM) BIG DATA FOR RA FLARE PREDICTION
ANNALS OF THE RHEUMATIC DISEASES(2020)
摘要
Background: Flare, relapse from status of treat-to-target (T2T, DAS28 Objectives: To develop and validate machine learning algorithms for flare prediction in RA. Methods: Patients were trained using SSDM and input their data, including demographic, comorbidities (COMBs), lab test, medications and monthly self-assessments, including DAS28, HAQ, SF-36, Hospital Anxiety and Depression Scale (HADS). The data was uploaded to cloud and synchronized to the mobile of authorized rheumatologists. The COMBs were by ICD-9, and medications were listed as cDMARDs, Bio (BioDMARDs), NSAIDs, Steroid, FS (food supplements), MC (medicine for COMBs), TCM (Traditional Chinese Medicine), and combinations. Results: From Jan of 2015 to Jan of 2020, 8811 RA patients, 85% female and 15% male, used to reach T2T. 4556 were flare-free and 4255 suffering at least one flare. The average 160 attributes were extracted from each flare-free patient at time of reaching T2T, and each flare patients at time of 3 months before the flare. Patients were randomly assigned as model setup (training) group (70%) and validation (testing) group30%. For training, data were processed using Python with statistical analyses in R. In R, random forests were implemented. Logistic regression via glm in base R. The random forest comprises a set of decision trees. “Splits” in the decision trees reflect binary (i.e., yes/no) respect to attributors. Bootstrapping was used to assess, quantify, and adjust for model optimism. Model performance was evaluated using AUC, precision and recall metrics. Brier scores for accuracy of probabilistic predictions ranged from 0 to 1 (0 is perfect discrimination). The testing showed model performance for prediction windows are 0.78 for AUC (95% CI), 0.71 for Recall (sensitivity), 0.195 for Brier score, and 0.68 for precision (true positive 893, false positive 417, false negative 367, true negative 966). Based on weighing in the random forest, the top 10 pro-flare attributes were CRP, swollen joint count (SJC), tender joint count (TJC), HAQ, DAS28, morning stiffness, gout, MCTD, OA, duration; while top 10 anti-flare attributes were cDMARDs+Bio, cDMARDs+steroid+NSAIDs, stable on HAQ, on morning stiffness, on SJC, medicine on COMBs, cDMARDs+TCM, stable on TJC, on ESR, income at 100-200k (Fig.1). The top weighing COMBs for pro-flaring were gout (0.81), MRD (0.75), OA (0.56), AS (0.48). The monotherapies with either Bio or NSAIDs, or steroid, or TCM was pro-flare; while with cDMARDs was anti-flare (-0.21). Conclusion: The attempt to develop a machine learning algorithm for RA flare prediction is successful. The discrimination was acceptable. The attributes of both pro-flare and anti-flare are identified, which may inspire the proactive intervention. Acknowledgments: SSDM was developed by Shanghai Gothic Internet Technology Co., Ltd. Disclosure of Interests: None declared
更多查看译文
AI 理解论文
溯源树
样例
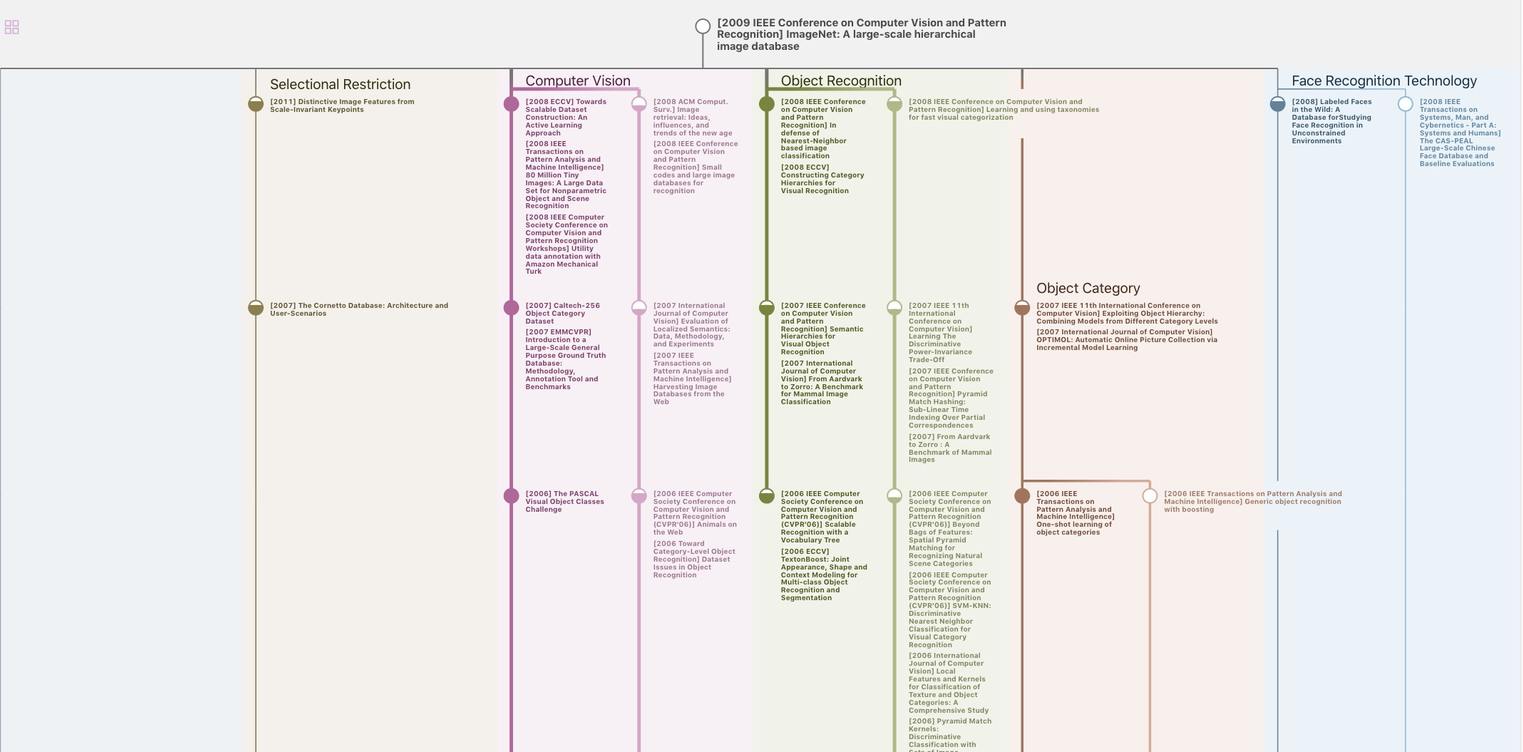
生成溯源树,研究论文发展脉络
Chat Paper
正在生成论文摘要