FEASIBILITY STUDY ON AN AUTOMATED QUANTITATIVE SYSTEM FOR ULTRASOUND JOINT INFLAMMATION ASSESSMENT IN RHEUMATOID ARTHRITIS USING DEEP LEARNING
ANNALS OF THE RHEUMATIC DISEASES(2020)
摘要
Background: The most widely accepted ultrasound (US) joint inflammation scoring system in rheumatoid arthritis (RA) is semi-quantitative in nature. This process involves manual image acquisition followed by image interpretation. The subjectivity inherent in manual scoring may be overcome by the development of an automated quantitative system to measure joint inflammation. Objectives: To develop an automated quantitative system to measure US detected power Doppler (PD) joint inflammation in patients with RA. Methods: The synovial region of interest (sROI) on US images at the metacarpophalangeal joints (MCPJs) and the metatarsophalangeal joint (MTPJs) within the Doppler box is manually segmented by a clinician experienced in musculoskeletal US (figure 1). PD joint inflammation was scored manually semi-quantitatively (0-3). Deep learning based image segmentation was applied to the US images to automatically identify sROI and quantify the amount of PD signals within the sROI (figure 1) to obtain a computer derived PD reading reflecting the extent of PD vascularity within the sROI. The performance of computer derived PD reading was evaluated in comparison with the clinician’s manual scoring. Results: 820 joints from bilateral 1st to 5th MCPJs and MTPJs in 41 adult RA patients (baseline characteristics: 75.6% Chinese; 73.2% female; mean (SD) DAS28, 4.23 (1.25); mean (SD) disease duration, 73.3 (57.8) months) were evaluated in this cross-sectional study. The respective mean (SD)/ median (IQR) computer derived PD readings were 0.13 (0.75)/0.04 (0.08), 1.62 (1.77)/1.21 (1.19) and 10.12 (6.86)/7.51 (5.24) for manual score 0, 1 and 2 (no joints had manual score 3), with statistically significant differences found among the different manual score classes (for non-normally distributed data, Kruskal-Wallis H-test, p=1.69 x 10-92, Mann-Whitney Test: manual score 0 versus 1, p=1.04 x 10-62; manual score 0 versus 2, p=3.28 x 10-43; manual score 1 versus 2, p=1.53 x 10-28). Area under the ROC curve (AUC) based on computer derived PD reading cut-off of 0.26 to identify manual score 0 versus 1 was 0.98, while AUC based on computer derived PD reading cut-off of 3.37 to identify manual score 1 versus 2 was 0.98. The overall agreement of the score classes (0, 1 and 2) based on computer prediction using the above cut-offs versus manual scores of 0, 1 and 2 is 791/820=96.46%. Table 1 summarizes the performance of computer prediction using the above cut-offs when compared to clinician evaluation (i.e. score 0 versus 1, comparing computer prediction with clinician evaluation, sensitivity=99.14% and specificity=97.00%; score 1 versus 2, comparing computer prediction with clinician evaluation, sensitivity=97.14% and specificity=93.97%). Conclusion: An automated quantitative system for US PD joint inflammation assessment using deep learning showed high sensitivity and specificity when results from computer prediction were compared to clinician evaluation. Further validation in a larger RA cohort with a longitudinal study design would be required. References: Nil Disclosure of Interests: None declared
更多查看译文
AI 理解论文
溯源树
样例
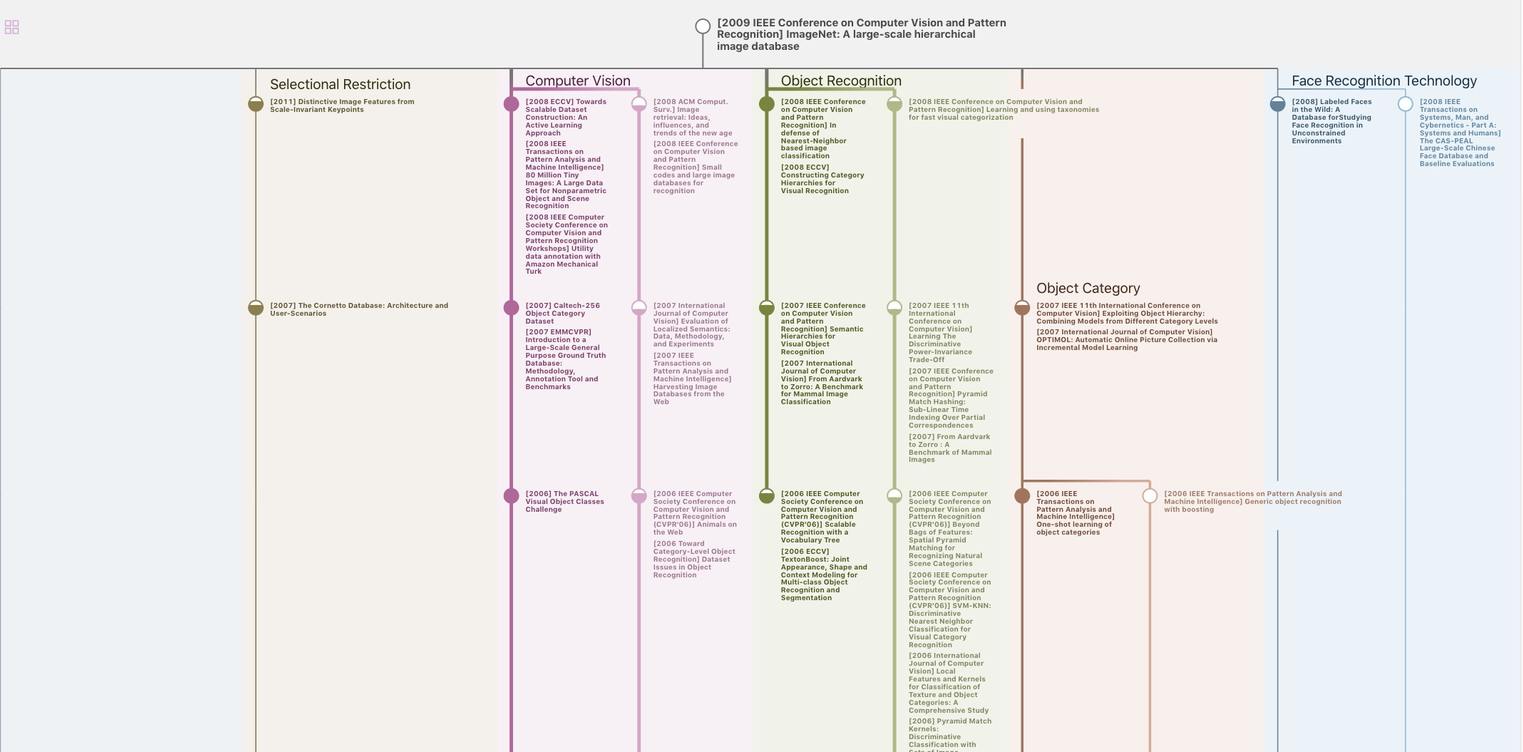
生成溯源树,研究论文发展脉络
Chat Paper
正在生成论文摘要