Deep learning enables discovery of highly potent anti-osteoporosis natural products.
European journal of medicinal chemistry(2020)
摘要
A pre-trained self-attentive message passing neural network (P-SAMPNN) model was developed based on our anti-osteoclastogenesis dataset for virtual screening purpose. Validation processes proved that P-SAMPNN model was significantly superior to the other base line models. A commercially available natural product library was virtually screened by the P-SAMPNN model and resulted in confirmed 5 hits from 10 selected virtual hits. Among the confirmed hits, compounds AP-123/40765213 and AE-562/43462182 are the nanomolar inhibitors against osteoclastogenesis with a new scaffold. Further studies indicate that AP-123/40765213 and AE-562/43462182 significantly suppress the mRNA expression of RANK and downregulate the expressions of osteoclasts-related genes Ctsk, Nfatc1, and Tracp. Our work demonstrated that P-SAMPNN method can guide phenotype-based drug discovery.
更多查看译文
AI 理解论文
溯源树
样例
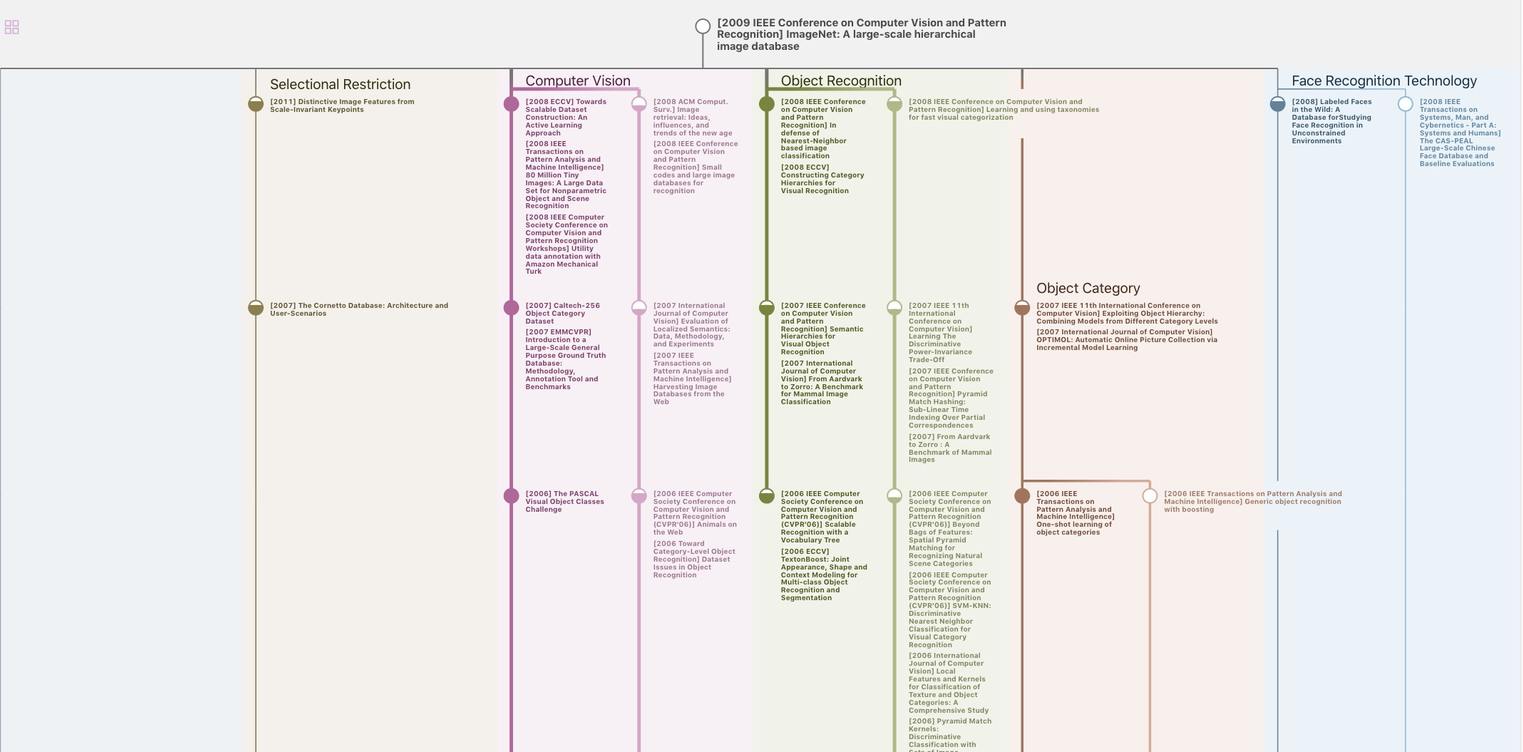
生成溯源树,研究论文发展脉络
Chat Paper
正在生成论文摘要