Dimensionality reduction of parameter-dependent problems through proper orthogonal decomposition
ANNALS OF MATHEMATICAL SCIENCES AND APPLICATIONS(2016)
摘要
The numerical solution of partial differential equations (PDEs) depending on parametrized or random input data is computationally intensive. Reduced order modeling techniques, such as the reduced basis methods, have been developed to alleviate this computational burden, and are nowadays exploited to accelerate real-time analysis, as well as the solution of PDE-constrained optimization and inverse problems. These methods are built upon low-dimensional spaces obtained by selecting a set of snapshots from a parametrically induced manifold. However, for these techniques to be effective, both parameter-dependent and random input data must be expressed in a convenient form. To address the former case, the empirical interpolation method has been developed. In the latter case, a spectral approximation of stochastic fields is often generated by means of a Karhunen-Loeve expansion. In all these cases, a low dimensional space to represent the function being approximated (PDE solution, parametrized data, stochastic field) can be obtained through proper orthogonal decomposition. Here, we review possible ways to exploit this methodology in these three contexts, we recall its optimality properties, and highlight the common mathematical structure beneath.
更多查看译文
关键词
Reduced order modeling,proper orthogonal decomposition,empirical interpolation,Karhunen-Loeve expansion
AI 理解论文
溯源树
样例
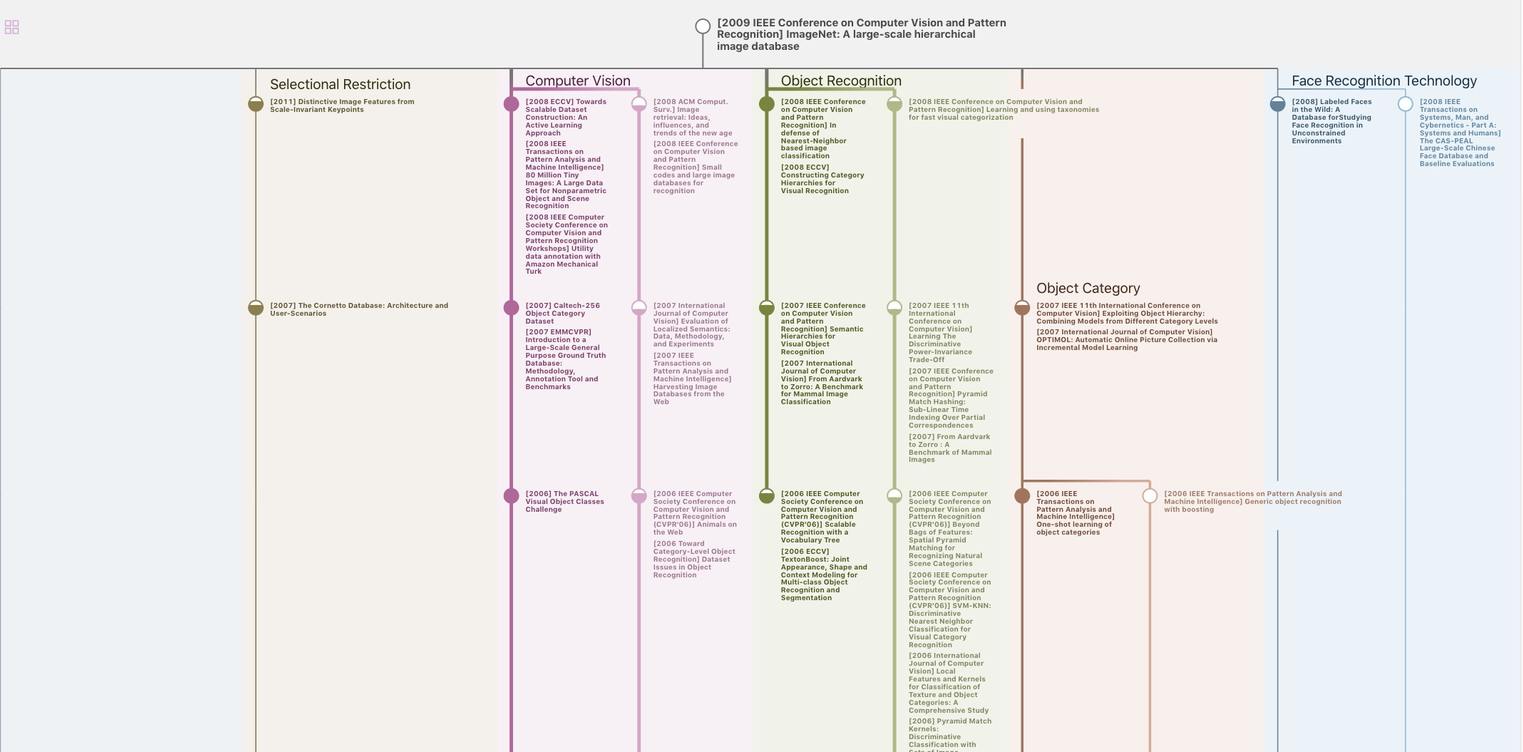
生成溯源树,研究论文发展脉络
Chat Paper
正在生成论文摘要