MINIMUM BAYES RISK TRAINING FOR END-TO-END SPEAKER-ATTRIBUTED ASR
2021 IEEE INTERNATIONAL CONFERENCE ON ACOUSTICS, SPEECH AND SIGNAL PROCESSING (ICASSP 2021)(2021)
摘要
Recently, an end-to-end speaker-attributed automatic speech recognition (E2E SA-ASR) model was proposed as a joint model of speaker counting, speech recognition and speaker identification for monaural overlapped speech. In the previous study, the model parameters were trained based on the speaker-attributed maximum mutual information (SA-MMI) criterion, with which the joint posterior probability for multi-talker transcription and speaker identification are maximized over training data. Although SA-MMI training showed promising results for overlapped speech consisting of various numbers of speakers, the training criterion was not directly linked to the final evaluation metric, i.e., speaker-attributed word error rate (SA-WER). In this paper, we propose a speaker-attributed minimum Bayes risk (SA-MBR) training method where the parameters are trained to directly minimize the expected SA-WER over the training data. Experiments using the LibriSpeech corpus show that the proposed SA-MBR training reduces the SA-WER by 9.0 % relative compared with the SA-MMI-trained model.(1)
更多查看译文
关键词
Speech recognition, speaker identification, speech separation, speaker counting, minimum Bayes risk training
AI 理解论文
溯源树
样例
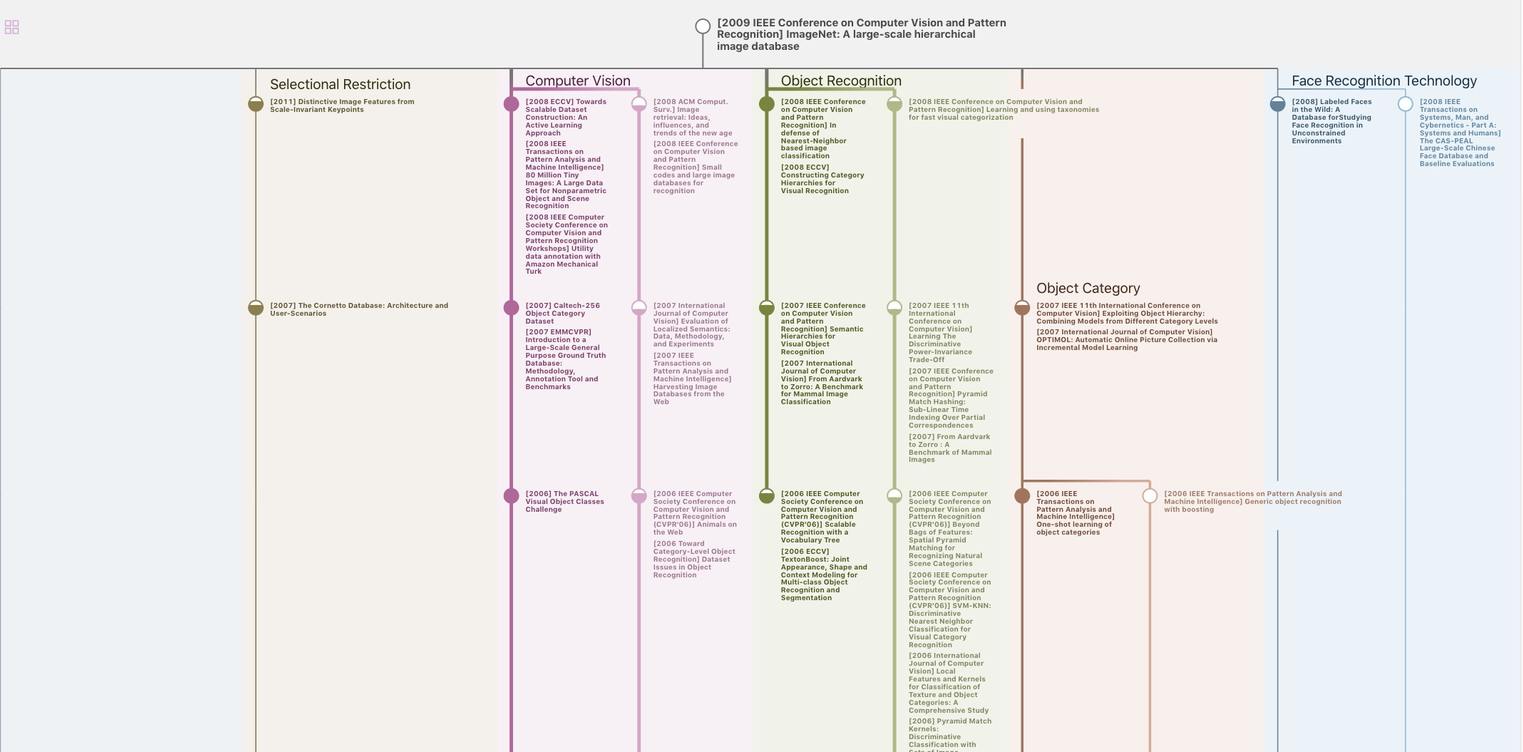
生成溯源树,研究论文发展脉络
Chat Paper
正在生成论文摘要