SEMI-SUPERVISED LEARNING FOR SINGING SYNTHESIS TIMBRE
2021 IEEE INTERNATIONAL CONFERENCE ON ACOUSTICS, SPEECH AND SIGNAL PROCESSING (ICASSP 2021)(2021)
摘要
We propose a semi-supervised singing synthesizer, which is able to learn new voices from audio data only, without any annotations such as phonetic segmentation. Our system is an encoder-decoder model with two encoders, linguistic and acoustic, and one (acoustic) decoder. In a first step, the system is trained in a supervised manner, using a labeled multi-singer dataset. Here, we ensure that the embeddings produced by both encoders are similar, so that we can later use the model with either acoustic or linguistic input features. To learn a new voice in an unsupervised manner, the pretrained acoustic encoder is used to train a decoder for the target singer. Finally, at inference, the pretrained linguistic encoder is used together with the decoder of the new voice, to produce acoustic features from linguistic input. We evaluate our system with a listening test and show that the results are comparable to those obtained with an equivalent supervised approach.
更多查看译文
关键词
Singing synthesis, semi-supervised, encoder-decoder, autoregressive, convolutional
AI 理解论文
溯源树
样例
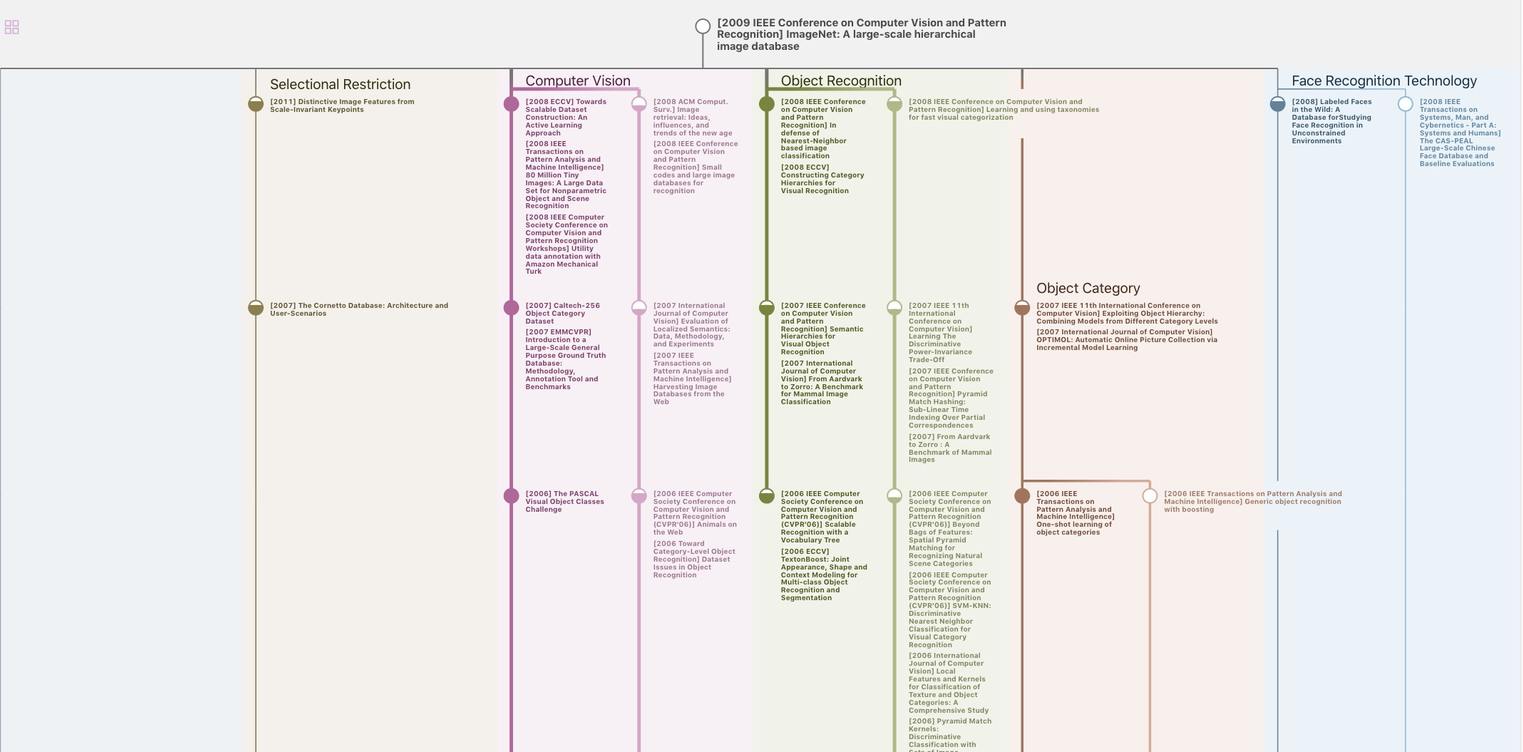
生成溯源树,研究论文发展脉络
Chat Paper
正在生成论文摘要