Comparative analysis of deep transfer learning performance on crop classification
SIGSPATIAL '20: 28th International Conference on Advances in Geographic Information Systems Seattle Washington November, 2020(2020)
摘要
Building accurate machine learning models for mapping crops using remote sensing imagery is a challenging task. Traditional solutions include per-pixel based and object-based classification solutions using coarse resolution imagery such as MODIS and LANDSAT. These models also require the development of hand-crafted features. In addition, these methods are computationally inefficient when used with very high resolution (VHR) imagery. Deep learning methods, with their ability to automatically learn relevant features provide an efficient alternative. However, deep learning methods are data-hungry, and require large amounts of labeled data sets to achieve accurate results. This is a challenge to the remote sensing community, since there is a lack of large labeled data sets in the domain. Transfer learning is a strategy that has been widely adapted across several domains and has allowed for the usage of deep neural networks even with limited labeled data. In this paper, we perform a comparative analysis of various transfer learning strategies for the domain of crop classification. We perform our experiments using three well-known neural networks and evaluate various strategies such as: (i) training the model from scratch using random weight initialization, (ii) simply using the pretrained models as feature extractors, (iii) finetuning deep CNNs by freezing the early layers of a pretrained CNN, and (iv) using the Imagenet pretrained weights as initialization, and their impact on classification performance.
更多查看译文
AI 理解论文
溯源树
样例
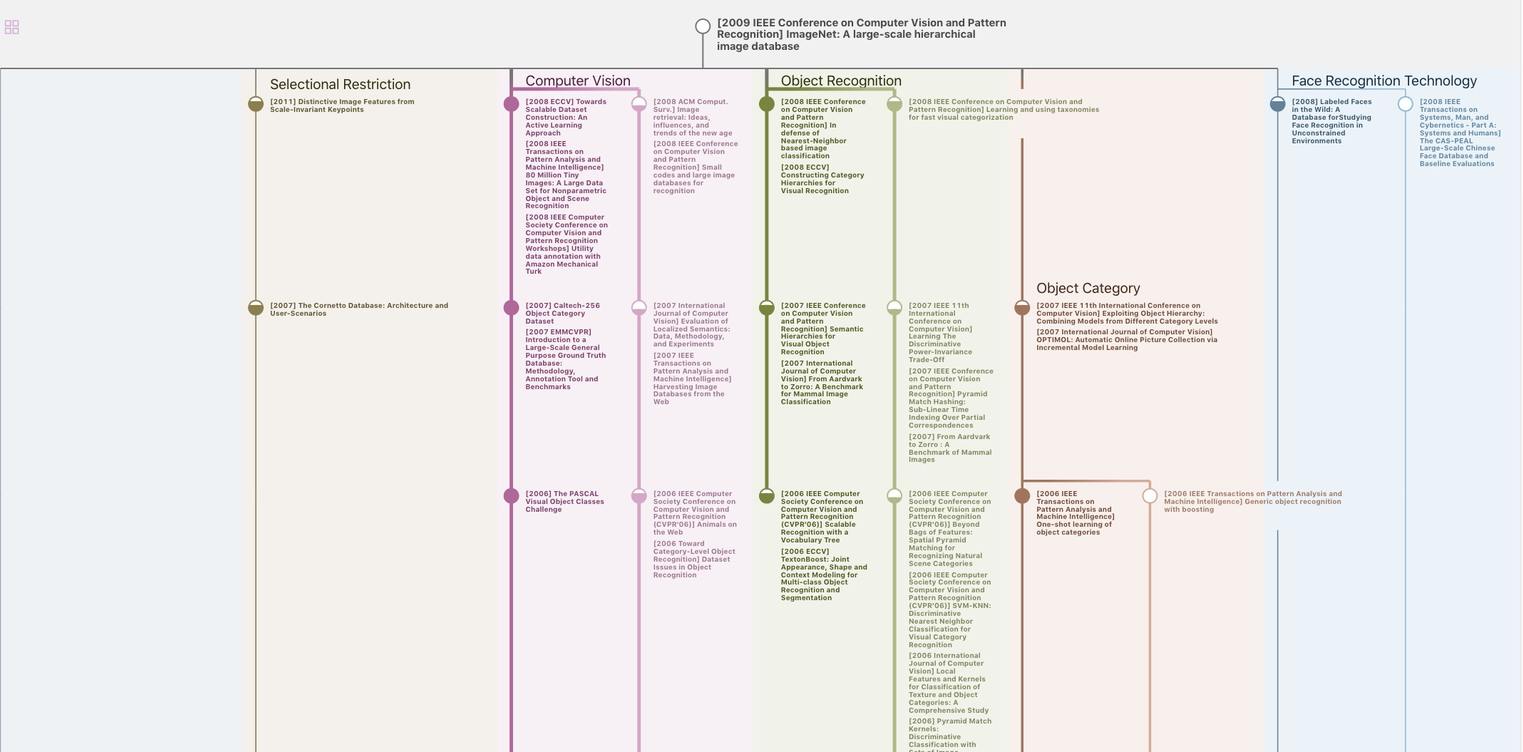
生成溯源树,研究论文发展脉络
Chat Paper
正在生成论文摘要