A Study of Policy Gradient on a Class of Exactly Solvable Models
arxiv(2020)
摘要
Policy gradient methods are extensively used in reinforcement learning as a way to optimize expected return. In this paper, we explore the evolution of the policy parameters, for a special class of exactly solvable POMDPs, as a continuous-state Markov chain, whose transition probabilities are determined by the gradient of the distribution of the policy's value. Our approach relies heavily on random walk theory, specifically on affine Weyl groups. We construct a class of novel partially observable environments with controllable exploration difficulty, in which the value distribution, and hence the policy parameter evolution, can be derived analytically. Using these environments, we analyze the probabilistic convergence of policy gradient to different local maxima of the value function. To our knowledge, this is the first approach developed to analytically compute the landscape of policy gradient in POMDPs for a class of such environments, leading to interesting insights into the difficulty of this problem.
更多查看译文
关键词
policy gradient,solvable models
AI 理解论文
溯源树
样例
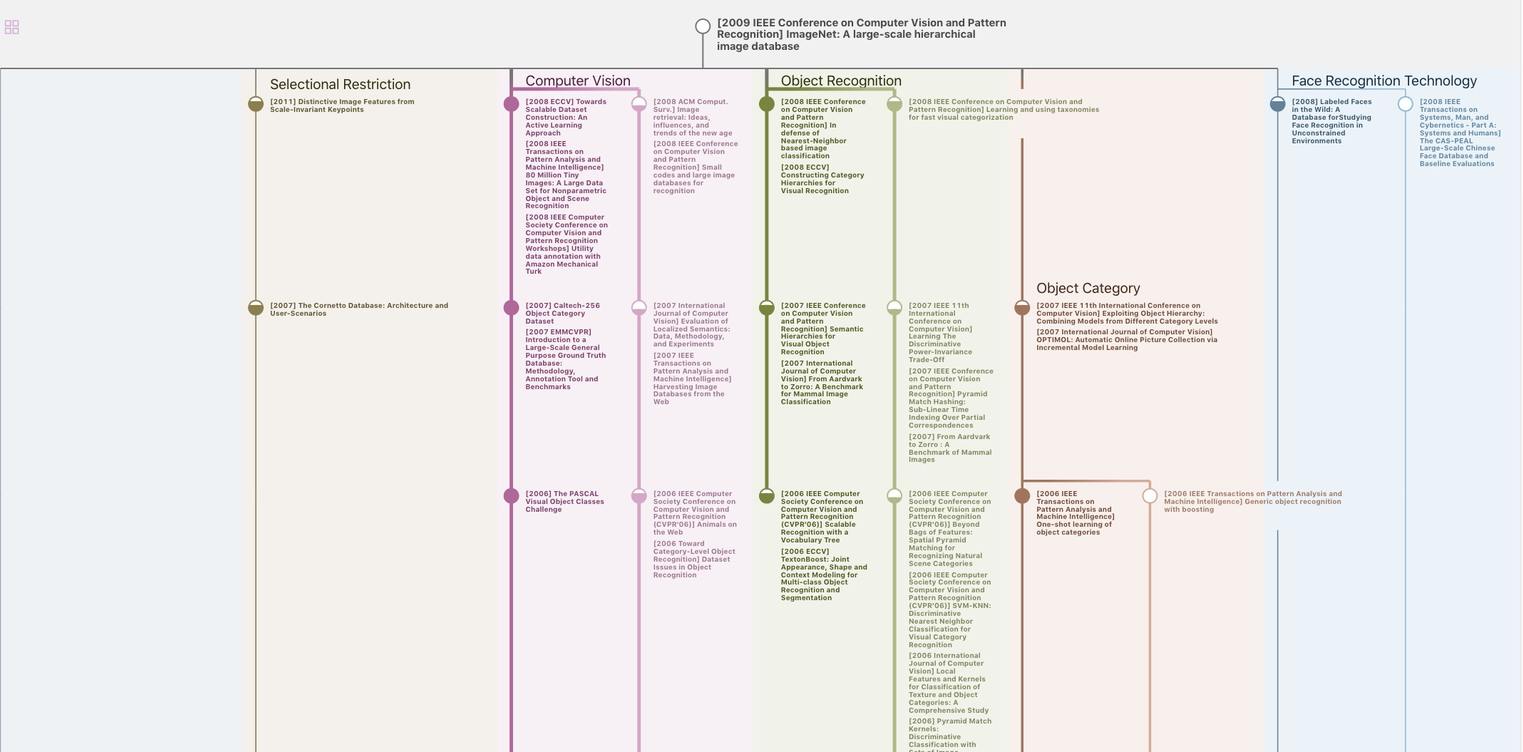
生成溯源树,研究论文发展脉络
Chat Paper
正在生成论文摘要