Learning Vector-valued Functions with Local Rademacher Complexity and Unlabeled Data
arXiv(2019)
摘要
We consider a general family of problems of which the output space admits vector-valued structure, covering a broad family of important domains, e.g. multi-label learning and multi-class classification. By using local Rademacher complexity and unlabeled data, we derived novel data-dependent excess risk bounds for vector-valued functions in both linear space and kernel space. The proposed bounds are much sharper than existing bounds and can be applied into specific vector-valued tasks in terms of different hypotheses sets and loss functions. Theoretical analysis motivates us to devise a unified learning framework for vector-valued functions based which is solved by proximal gradient descent on the primal, achieving a much better tradeoff between accuracy and efficiency. Empirical results on several benchmark datasets show that the proposed algorithm outperforms compared methods significantly, which coincides with our theoretical analysis.
更多查看译文
关键词
local rademacher complexity,learning,functions,vector-valued
AI 理解论文
溯源树
样例
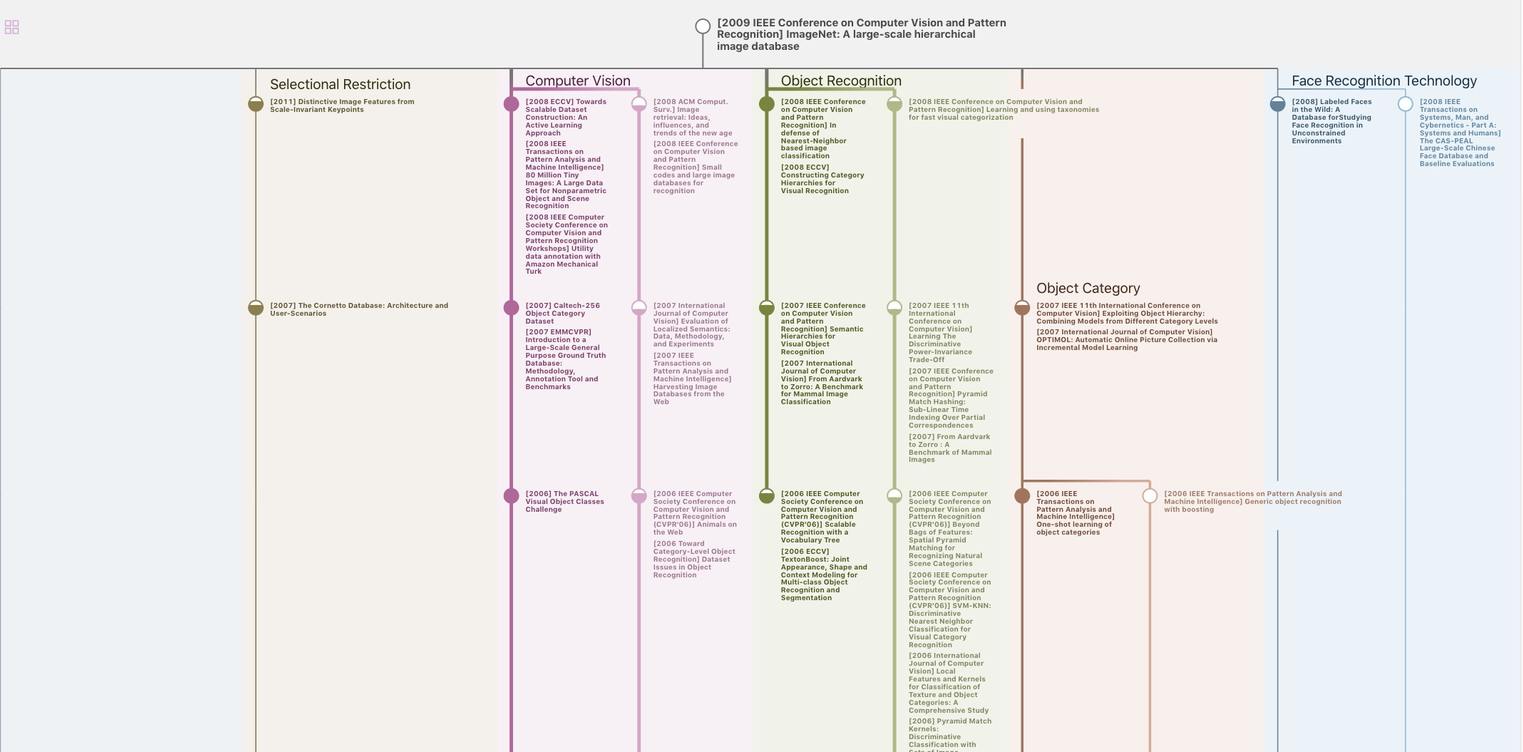
生成溯源树,研究论文发展脉络
Chat Paper
正在生成论文摘要