View selection in multi-view stacking: choosing the meta-learner
Advances in Data Analysis and Classification(2024)
摘要
Multi-view stacking is a framework for combining information from different views (i.e. different feature sets) describing the same set of objects. In this framework, a base-learner algorithm is trained on each view separately, and their predictions are then combined by a meta-learner algorithm. In a previous study, stacked penalized logistic regression, a special case of multi-view stacking, has been shown to be useful in identifying which views are most important for prediction. In this article we expand this research by considering seven different algorithms to use as the meta-learner, and evaluating their view selection and classification performance in simulations and two applications on real gene-expression data sets. Our results suggest that if both view selection and classification accuracy are important to the research at hand, then the nonnegative lasso, nonnegative adaptive lasso and nonnegative elastic net are suitable meta-learners. Exactly which among these three is to be preferred depends on the research context. The remaining four meta-learners, namely nonnegative ridge regression, nonnegative forward selection, stability selection and the interpolating predictor, show little advantages in order to be preferred over the other three.
更多查看译文
关键词
Multi-view learning,Stacked generalization,Feature selection,Classification,62,68
AI 理解论文
溯源树
样例
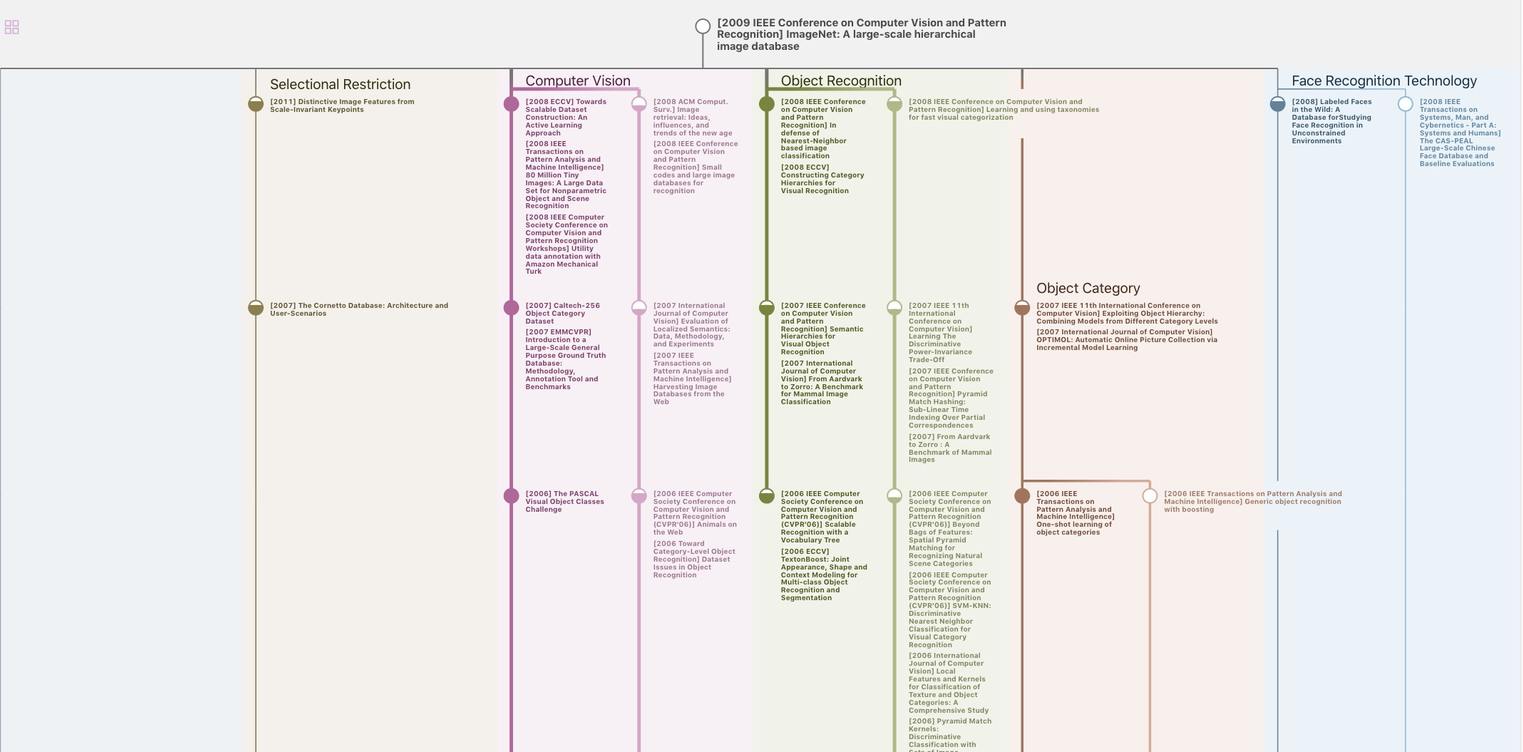
生成溯源树,研究论文发展脉络
Chat Paper
正在生成论文摘要