Correspondence Matrices are Underrated
2020 International Conference on 3D Vision (3DV)(2020)
摘要
Point-cloud registration (PCR) is an important task in various applications such as robotic manipulation, augmented and virtual reality, SLAM, etc. PCR is an optimization problem involving minimization over two different types of interdependent variables: transformation parameters and point-to-point correspondences. Recent developments in deep-learning have produced computationally fast approaches for PCR. The loss functions that are optimized in these networks are based on the error in the transformation parameters. We hypothesize that these methods would perform significantly better if they calculated their loss function using correspondence error instead of only using error in transformation parameters. We define correspondence error as a metric based on incorrectly matched point pairs. We provide a fundamental explanation for why this is the case and test our hypothesis by modifying existing methods to use correspondence-based loss instead of transformation-based loss. These experiments show that the modified networks converge faster and register more accurately even at larger misalignment when compared to the original networks.
更多查看译文
关键词
registration,point cloud registration,deep learning,3D reconstruction,Partial point cloud registration,Point cloud,outlier,3D perception,correspondence,point to point correspondence,global registration,global point cloud registration,partial point cloud
AI 理解论文
溯源树
样例
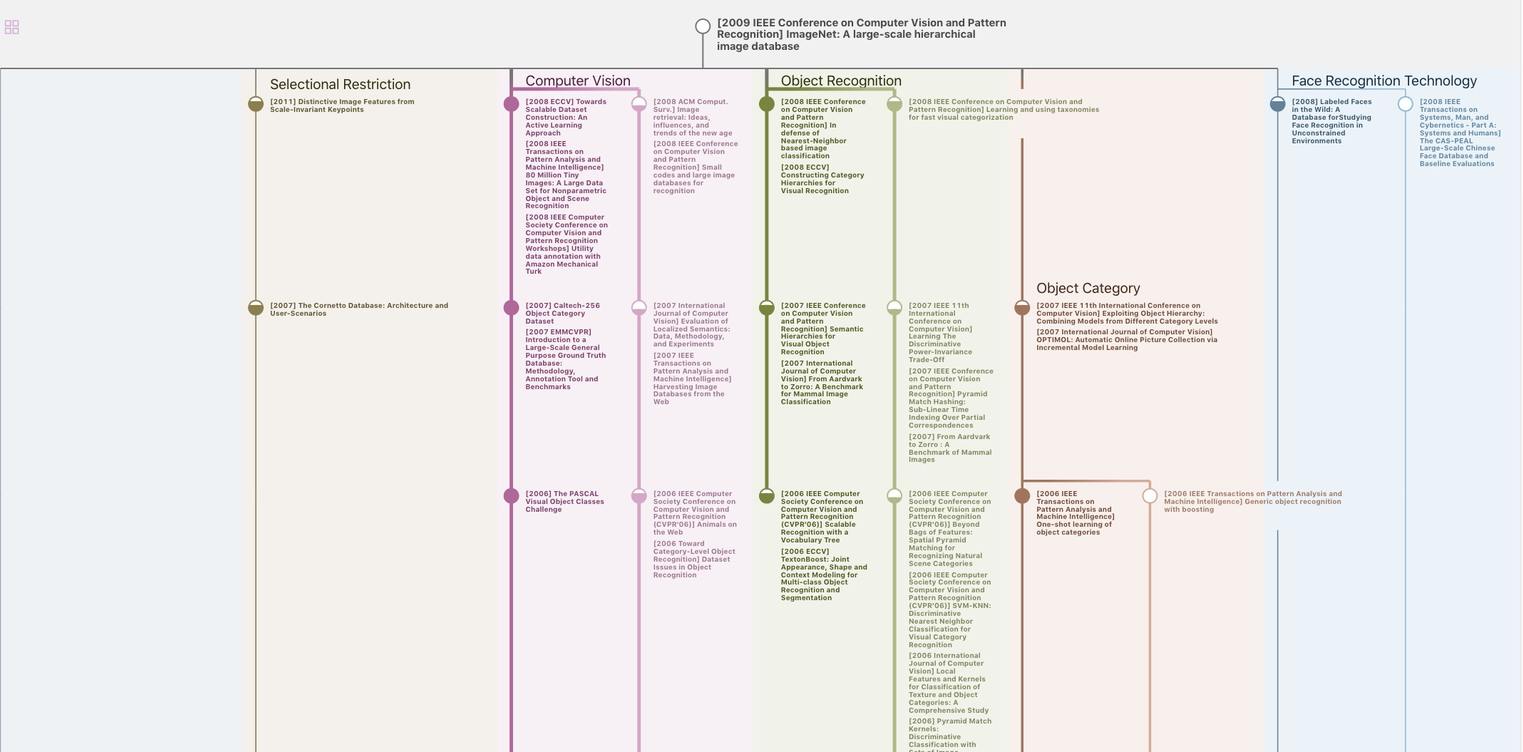
生成溯源树,研究论文发展脉络
Chat Paper
正在生成论文摘要