Learning Implicit Generative Models by Teaching Density Estimators
MACHINE LEARNING AND KNOWLEDGE DISCOVERY IN DATABASES, ECML PKDD 2020, PT II(2021)
摘要
Implicit generative models are difficult to train as no explicit density functions are defined. Generative adversarial nets (GANs) present a minimax framework to train such models, which however can suffer from mode collapse due to the nature of the JS-divergence. This paper presents a learning by teaching (LBT) approach to learning implicit models, which intrinsically avoids the mode collapse problem by optimizing a KL-divergence rather than the JS-divergence in GANs. In LBT, an auxiliary density estimator is introduced to fit the implicit model's distribution while the implicit model teaches the density estimator to match the data distribution. LBT is formulated as a bilevel optimization problem, whose optimal generator matches the true data distribution. LBT can be naturally integrated with GANs to derive a hybrid LBT-GAN that enjoys complimentary benefits. Finally, we present a stochastic gradient ascent algorithm with unrolling to solve the challenging learning problems. Experimental results demonstrate the effectiveness of our method.
更多查看译文
关键词
Deep generative models, Generative adversarial nets, Mode collapse problem
AI 理解论文
溯源树
样例
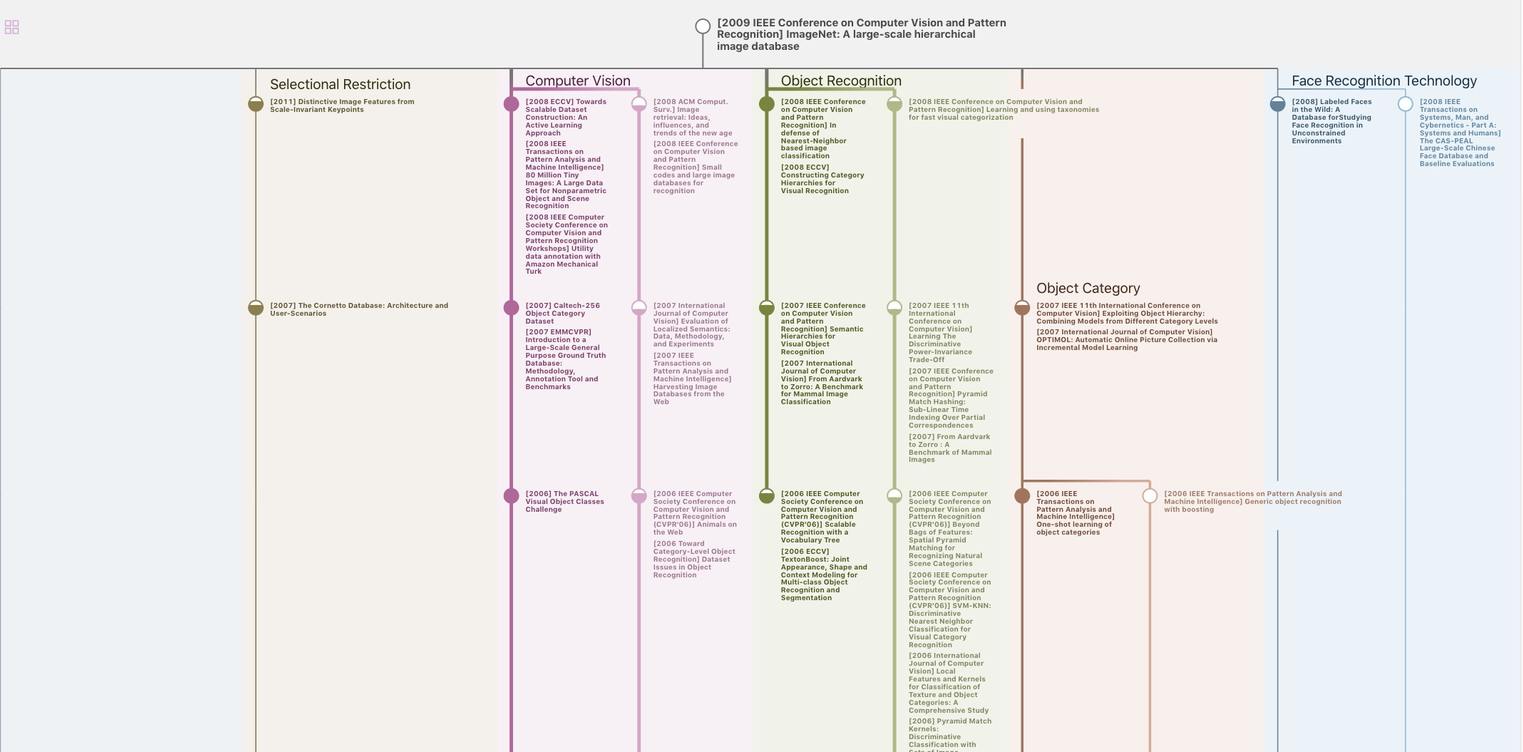
生成溯源树,研究论文发展脉络
Chat Paper
正在生成论文摘要