Learning Fuzzy Automaton’s Event Transition Matrix When Post-Event State Is Unknown
IEEE Transactions on Cybernetics(2022)
摘要
Compared to other system modeling techniques, the fuzzy discrete event systems (FDESs) methodology has the unique capability of modeling a class of event-driven systems as fuzzy automata with ambiguous state and event-invoked state transition. In two recent papers, we developed algorithms for online-supervised learning of the fuzzy automaton’s event transition matrix using fuzzy states before and after the occurrence of fuzzy events. The post-event state was assumed to be readily available while the pre-event state was either directly available or estimatable through learning. This article is focused on algorithm development for learning the transition matrix in a different setting—when the pre-event state is available but the post-event state is not. We suppose the post-event state is described by a fuzzy set that is linked to a (physical) variable whose value is available. Stochastic-gradient-descent-based algorithms are developed that can learn the transition matrix plus the parameters of the fuzzy sets when the fuzzy sets are of the Gaussian type. Computer simulation results are presented to confirm the theoretical development.
更多查看译文
关键词
Fuzzy automata,fuzzy discrete event systems (FDESs),stochastic gradient descent,supervised learning
AI 理解论文
溯源树
样例
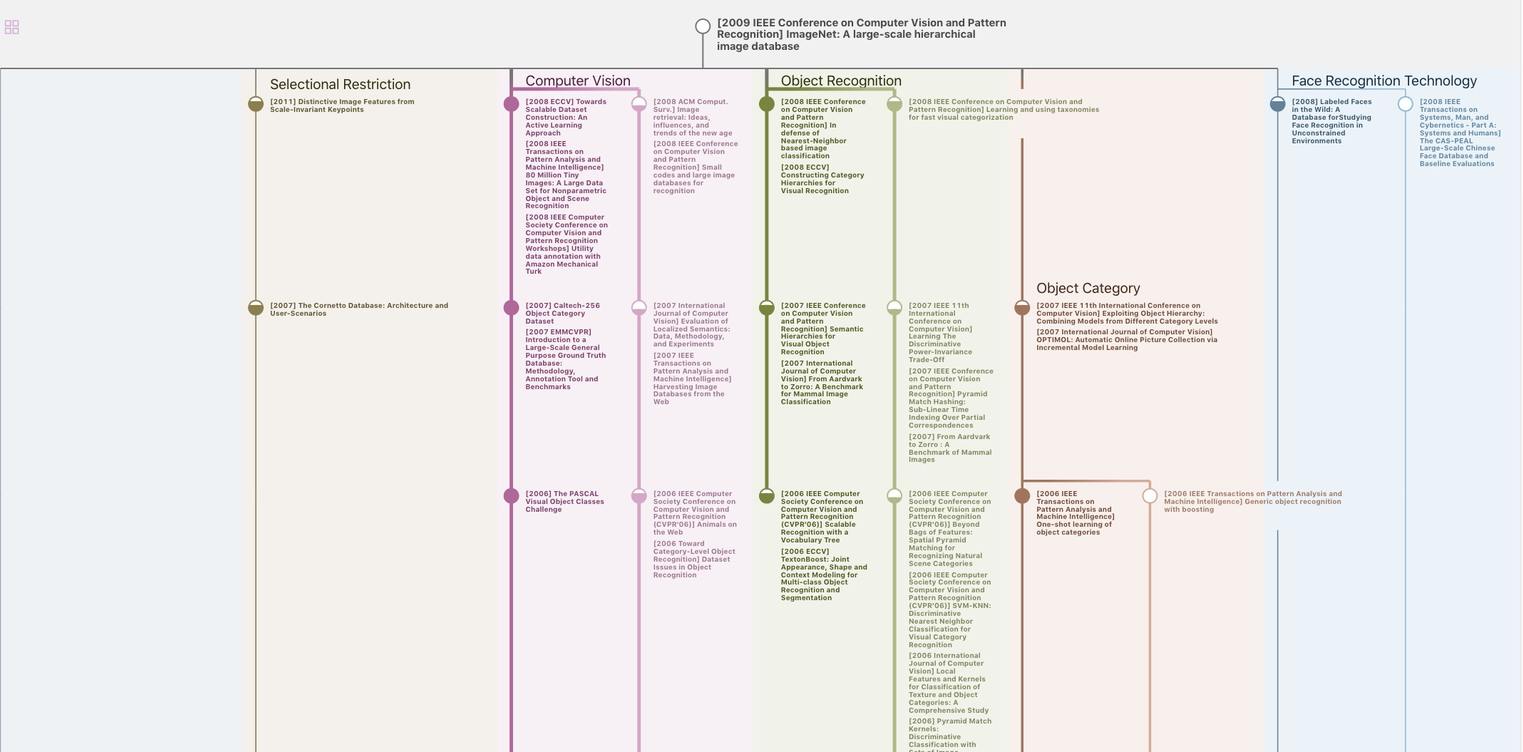
生成溯源树,研究论文发展脉络
Chat Paper
正在生成论文摘要