Point Cloud Attribute Compression via Successive Subspace Graph Transform
VCIP(2020)
摘要
Inspired by the recently proposed successive subspace learning (SSL) principles, we develop a successive subspace graph transform (SSGT) to address point cloud attribute compression in this work. The octree geometry structure is utilized to partition the point cloud, where every node of the octree represents a point cloud subspace with a certain spatial size. We design a weighted graph with self-loop to describe the subspace and define a graph Fourier transform based on the normalized graph Laplacian. The transforms are applied to large point clouds from the leaf nodes to the root node of the octree recursively, while the represented subspace is expanded from the smallest one to the whole point cloud successively. It is shown by experimental results that the proposed SSGT method offers better R-D performances than the previous Region Adaptive Haar Transform (RAHT) method.
更多查看译文
关键词
successive subspace graph
AI 理解论文
溯源树
样例
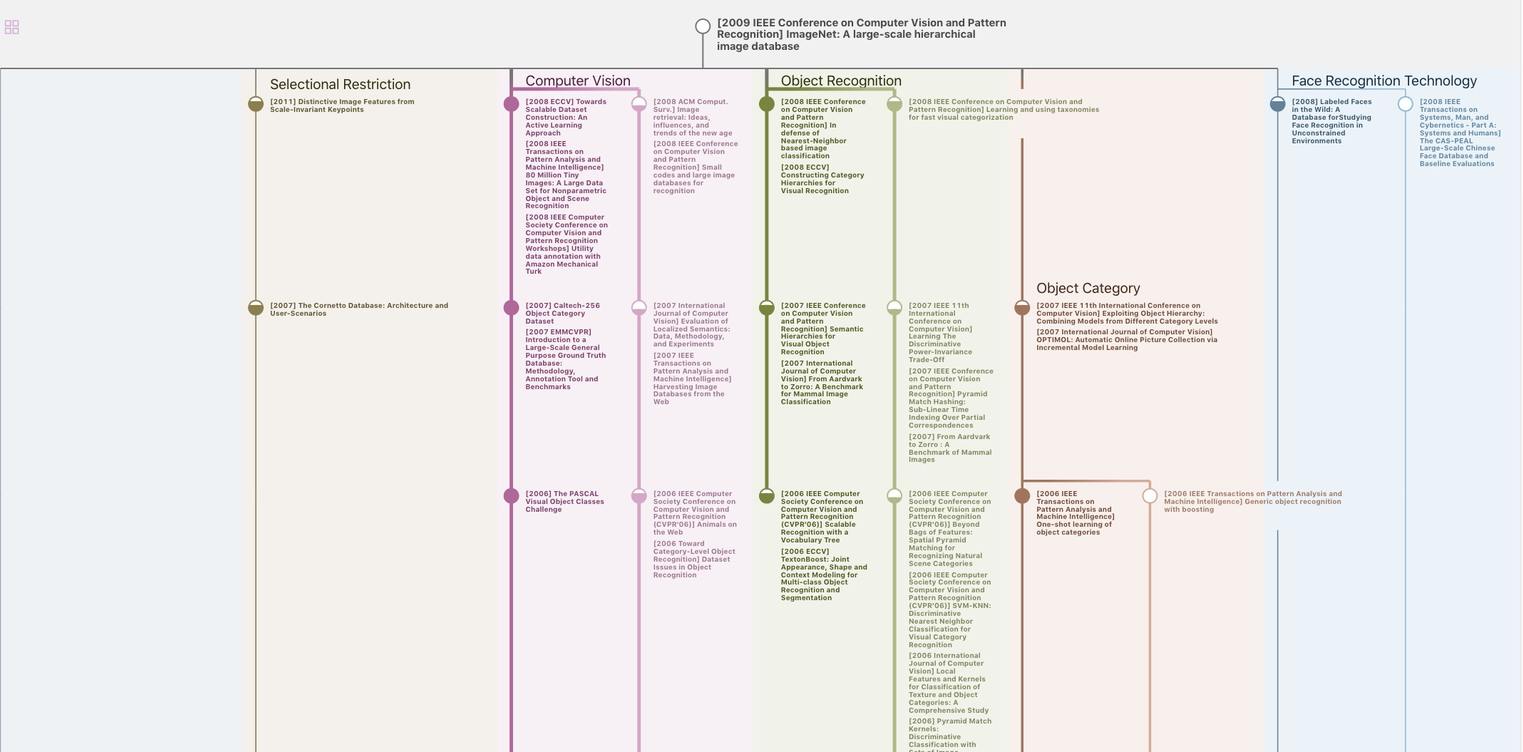
生成溯源树,研究论文发展脉络
Chat Paper
正在生成论文摘要