Wavelet-Based Dual Recursive Network for Image Super-Resolution
IEEE Transactions on Neural Networks and Learning Systems(2022)
摘要
Although remarkable progress has been made on single-image super-resolution (SISR), deep learning methods cannot be easily applied to real-world applications due to the requirement of its heavy computation, especially for mobile devices. Focusing on the fewer parameters and faster inference SISR approach, we propose an efficient and time-saving wavelet transform-based network architecture, where the image super-resolution (SR) processing is carried out in the wavelet domain. Different from the existing methods that directly infer high-resolution (HR) image with the input low-resolution (LR) image, our approach first decomposes the LR image into a series of wavelet coefficients (WCs) and the network learns to predict the corresponding series of HR WCs and then reconstructs the HR image. Particularly, in order to further enhance the relationship between WCs and image deep characteristics, we propose two novel modules [wavelet feature mapping block (WFMB) and wavelet coefficients reconstruction block (WCRB)] and a dual recursive framework for joint learning strategy, thus forming a WCs prediction model to realize the efficient and accurate reconstruction of HR WCs. Experimental results show that the proposed method can outperform state-of-the-art methods with more than a
$2\times $
reduction in model parameters and computational complexity.
更多查看译文
关键词
Computational complexity,model parameters,single-image super-resolution (SISR),time-saving,wavelet coefficients (WCs)
AI 理解论文
溯源树
样例
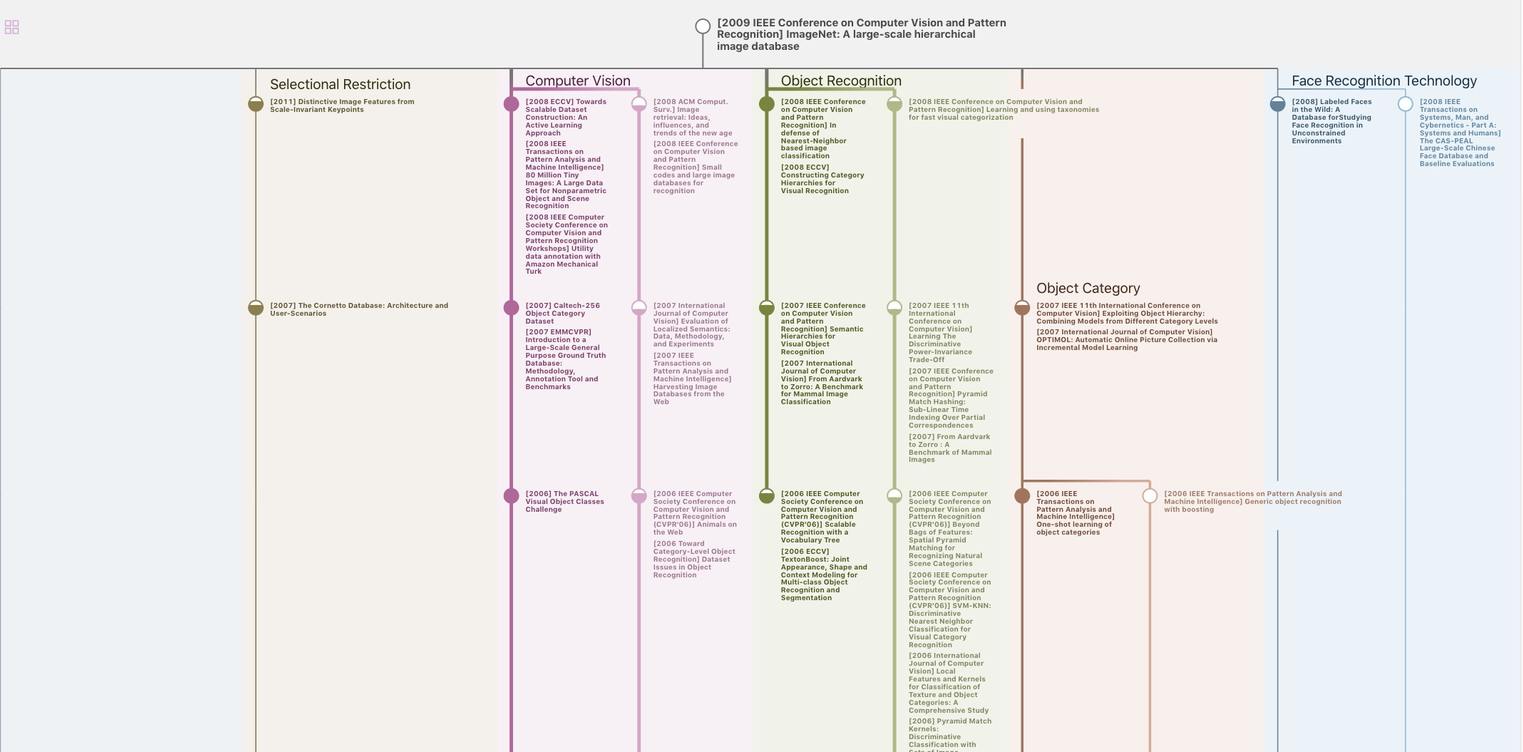
生成溯源树,研究论文发展脉络
Chat Paper
正在生成论文摘要