Implicit Under-Parameterization Inhibits Data-Efficient Deep Reinforcement Learning
ICLR(2021)
摘要
We identify a fundamental implicit under-parameterization phenomenon in value-based deep RL methods that use bootstrapping: when value functions, approximated using deep neural networks, are trained with gradient descent using iterated regression onto target values generated by previous instances of the value network, more gradient updates decrease the expressivity of the current value network. We characterize this loss of expressivity via a rank collapse of the learned value network features and show that it corresponds to a drop in performance. We demonstrate this phenomenon on popular domains including Atari and Gym benchmarks and in both offline and online RL settings. We formally analyze this phenomenon and show that it results from a pathological interaction between bootstrapping and gradient-based optimization. Finally, we show that mitigating implicit under- parameterization by controlling rank collapse improves performance.
更多查看译文
关键词
reinforcement learning,deep,under-parameterization,data-efficient
AI 理解论文
溯源树
样例
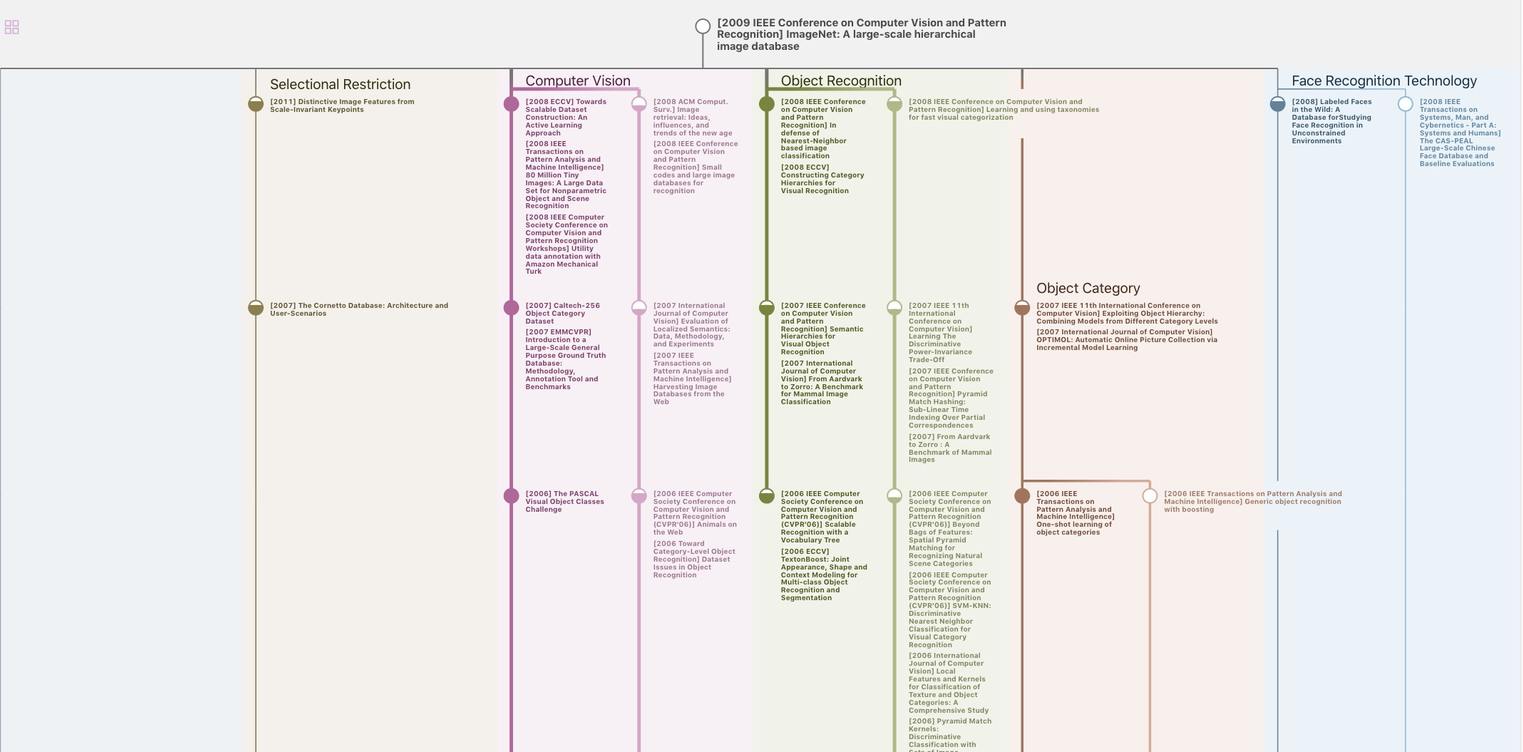
生成溯源树,研究论文发展脉络
Chat Paper
正在生成论文摘要