Explainable Machine Learning for Public Policy: Use Cases, Gaps, and Research Directions
arxiv(2023)
摘要
Explainability is highly-desired in Machine Learning (ML) systems supporting high-stakes policy decisions in areas such as health, criminal justice, education, and employment. While the field of explainable ML has expanded in recent years, much of this work has not taken real-world needs into account. A majority of proposed methods are designed with \textit{generic} explainability goals without well-defined use-cases or intended end-users and evaluated on simplified tasks, benchmark problems/datasets, or with proxy users (e.g., AMT). We argue that these simplified evaluation settings do not capture the nuances and complexities of real-world applications. As a result, the applicability and effectiveness of this large body of theoretical and methodological work in real-world applications are unclear. In this work, we take steps toward addressing this gap for the domain of public policy. First, we identify the primary use-cases of explainable ML within public policy problems. For each use case, we define the end-users of explanations and the specific goals the explanations have to fulfill. Finally, we map existing work in explainable ML to these use-cases, identify gaps in established capabilities, and propose research directions to fill those gaps to have a practical societal impact through ML. The contribution is 1) a methodology for explainable ML researchers to identify use cases and develop methods targeted at them and 2) using that methodology for the domain of public policy and giving an example for the researchers on developing explainable ML methods that result in real-world impact.
更多查看译文
关键词
explainable machine learning,interpretable machine learning,public policy
AI 理解论文
溯源树
样例
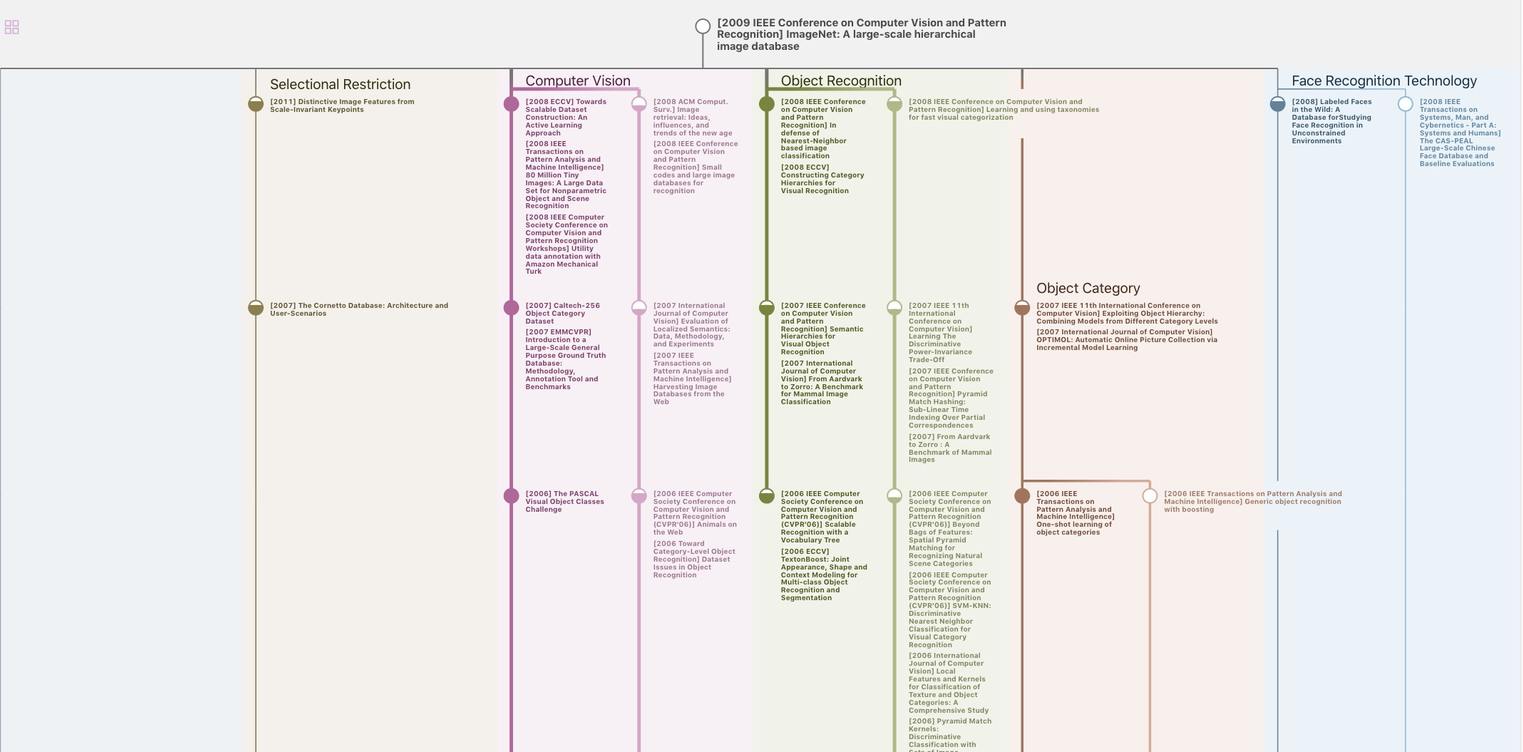
生成溯源树,研究论文发展脉络
Chat Paper
正在生成论文摘要