Learning to Infer Unseen Attribute-Object Compositions
arxiv(2020)
摘要
The composition recognition of unseen attribute-object is critical to make machines learn to decompose and compose complex concepts like people. Most of the existing methods are limited to the composition recognition of single-attribute-object, and can hardly distinguish the compositions with similar appearances. In this paper, a graph-based model is proposed that can flexibly recognize both single- and multi-attribute-object compositions. The model maps the visual features of images and the attribute-object category labels represented by word embedding vectors into a latent space. Then, according to the constraints of the attribute-object semantic association, distances are calculated between visual features and the corresponding label semantic features in the latent space. During the inference, the composition that is closest to the given image feature among all compositions is used as the reasoning result. In addition, we build a large-scale Multi-Attribute Dataset (MAD) with 116,099 images and 8,030 composition categories. Experiments on MAD and two other single-attribute-object benchmark datasets demonstrate the effectiveness of our approach.
更多查看译文
关键词
learning,attribute-object
AI 理解论文
溯源树
样例
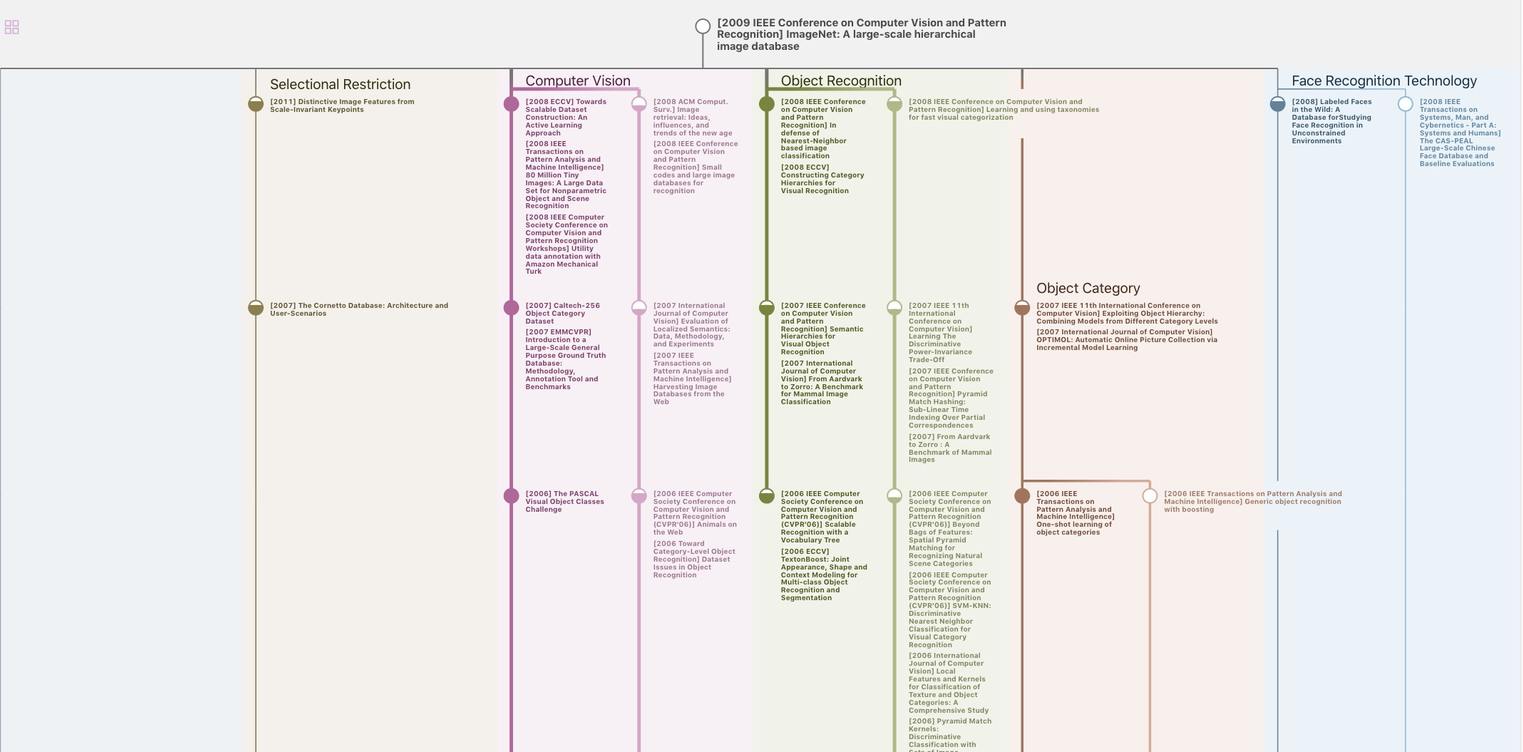
生成溯源树,研究论文发展脉络
Chat Paper
正在生成论文摘要