Direct Grid-Based Nonadiabatic Dynamics on Machine-Learned Potential Energy Surfaces: Application to Spin-Forbidden Processes.
JOURNAL OF PHYSICAL CHEMISTRY A(2020)
摘要
We have recently shown how high-accuracy wave function grid-based propagation schemes, such as the multiconfiguration time-dependent Hartree (MCTDH) method, can be combined with machine-learning (ML) descriptions of PESs to yield an "on-the-fly" direct dynamics scheme which circumvents potential energy surface (PES) prefitting. To date, our approach has been demonstrated in the ground-state dynamics and nonadiabatic spin-allowed dynamics of several molecular systems. Expanding on this successful previous work, this Article demonstrates how our ML-based quantum dynamics scheme can be adapted to model nonadiabatic dynamics for spin-forbidden processes such as intersystem crossing (ISC), opening up new possibilities for modeling chemical dynamic phenomena driven by spin-orbit coupling. After describing modifications to diabatization schemes to enable accurate and robust treatment or electronic states of different spin-multiplicity, we demonstrate our methodology in applications to modeling ISC in SO2 and thioformaldehyde, benchmarking our results against previous trajectory- and grid-based calculations. As a relatively efficient tool for modeling spin-forbidden nonadiabatic dynamics without demanding any prefitting of PESs, our overall strategy is a potentially powerful tool for modeling important photochemical systems, such as photoactivated pro-drugs and organometallic catalysts.
更多查看译文
AI 理解论文
溯源树
样例
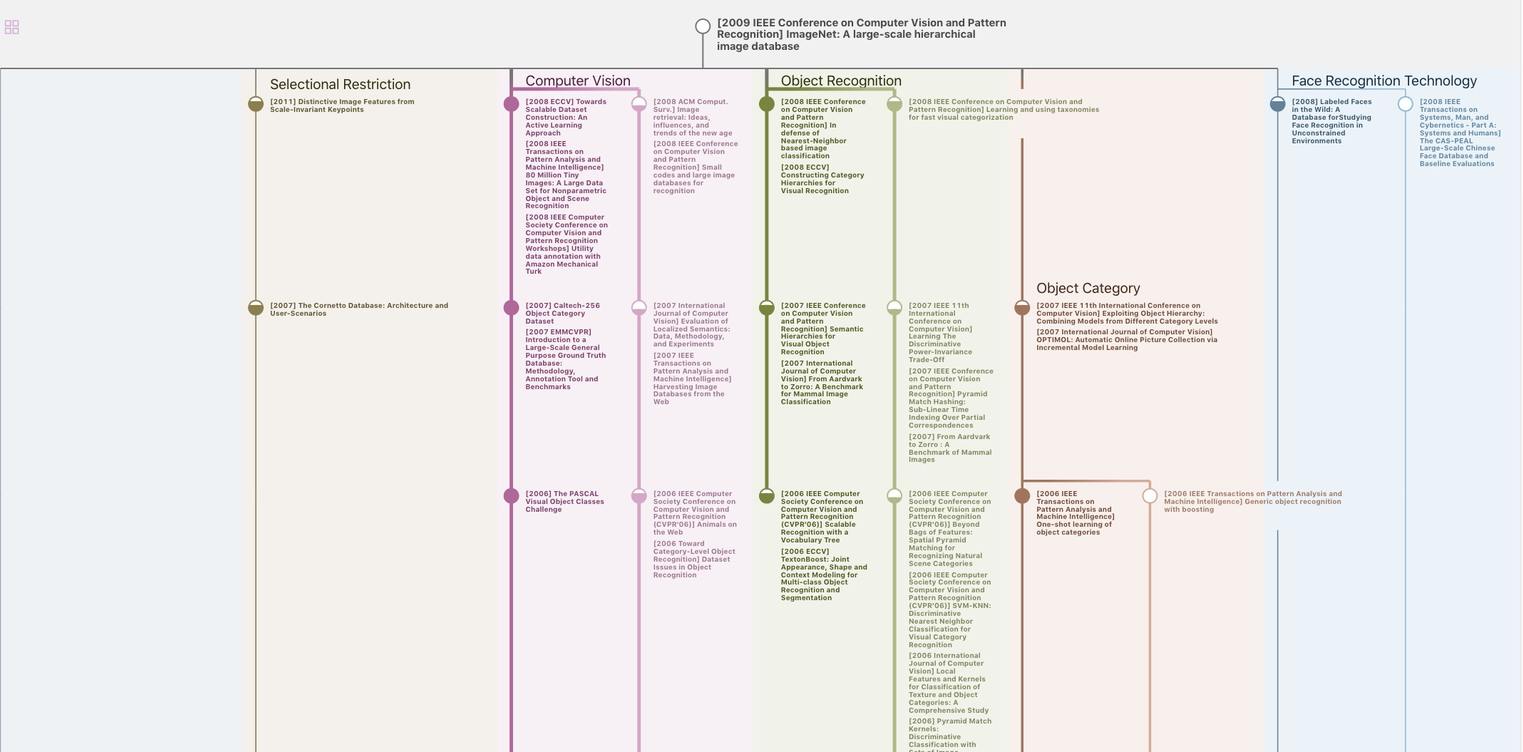
生成溯源树,研究论文发展脉络
Chat Paper
正在生成论文摘要