Speech SIMCLR: Combining Contrastive and Reconstruction Objective for Self-supervised Speech Representation Learning
arxiv(2020)
摘要
Self-supervised visual pretraining has shown significant progress recently. Among those methods, SimCLR greatly advanced the state of the art in self-supervised and semi-supervised learning on ImageNet. The input feature representations for speech and visual tasks are both continuous, so it is natural to consider applying similar objective on speech representation learning. In this paper, we propose Speech SimCLR, a new self-supervised objective for speech representation learning. During training, Speech SimCLR applies augmentation on raw speech and its spectrogram. Its objective is the combination of contrastive loss that maximizes agreement between differently augmented samples in the latent space and reconstruction loss of input representation. The proposed method achieved competitive results on speech emotion recognition and speech recognition. When used as feature extractor, our best model achieved 5.89% word error rate on LibriSpeech test-clean set using LibriSpeech 960 hours as pretraining data and LibriSpeech train-clean-100 set as fine-tuning data, which is the lowest error rate obtained in this setup to the best of our knowledge.
更多查看译文
关键词
unsupervised pretraining,speech recognition,speech emotion recognition,simclr,reconstruction objective
AI 理解论文
溯源树
样例
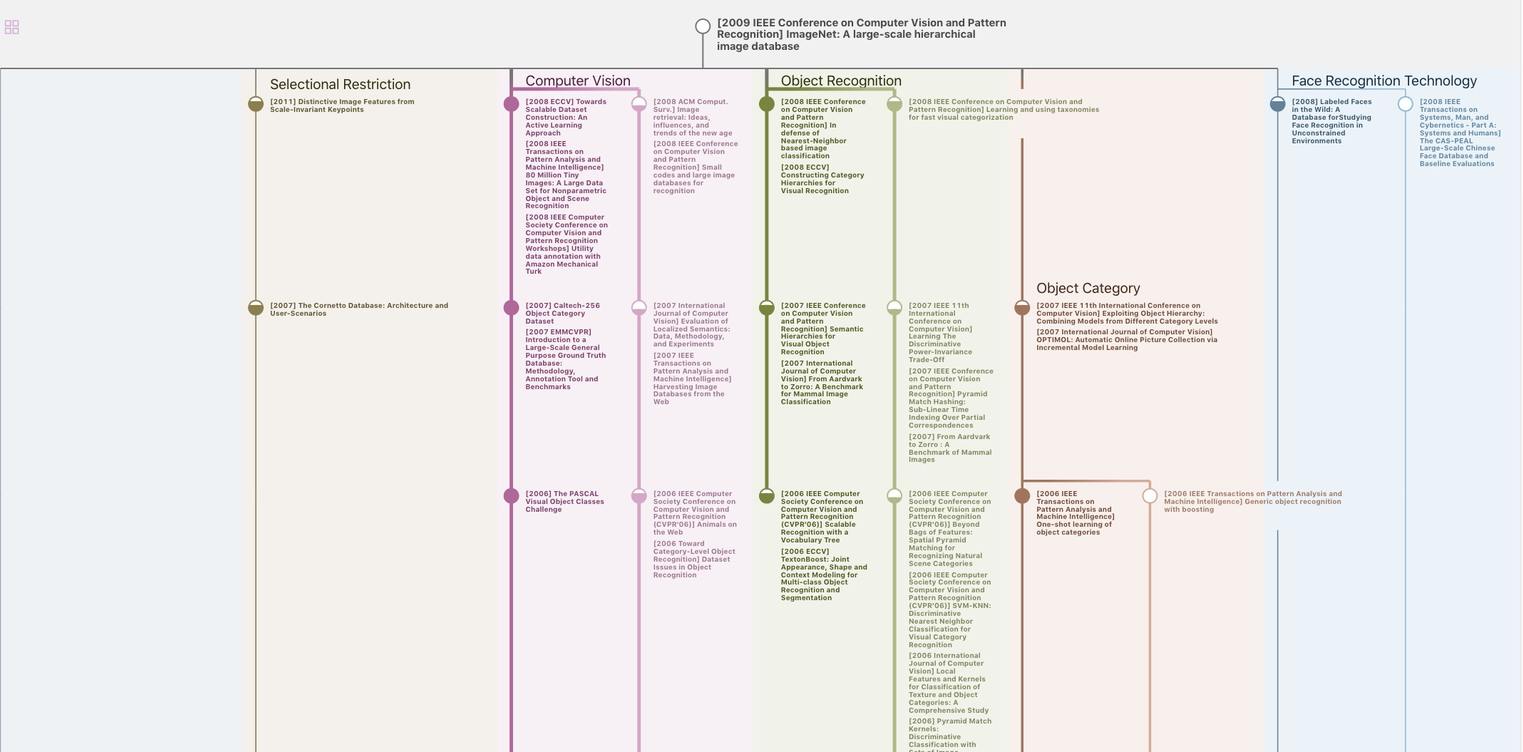
生成溯源树,研究论文发展脉络
Chat Paper
正在生成论文摘要