Neural Networks for Approximate DNF Counting: An Abridged Report
user-5f1696ff4c775ed682f5929f(2020)
摘要
Weighted model counting (WMC) has emerged as a prevalent approach for probabilistic inference. In its most general form, WMC is# P-hard. Weighted DNF counting (weighted# DNF) is a special case where approximations with probabilistic guarantees are obtained in O (nm), where n denotes the number of variables, and m the number of clauses of the input DNF, but this is not scalable in practice. In this paper, we propose a novel approach for weighted# DNF that combines approximate model counting with deep learning, and accurately approximates model counts in linear time when width is bounded. We conduct experiments to validate our method, and show that our model learns and generalizes very well to large-scale# DNF instances.
更多查看译文
AI 理解论文
溯源树
样例
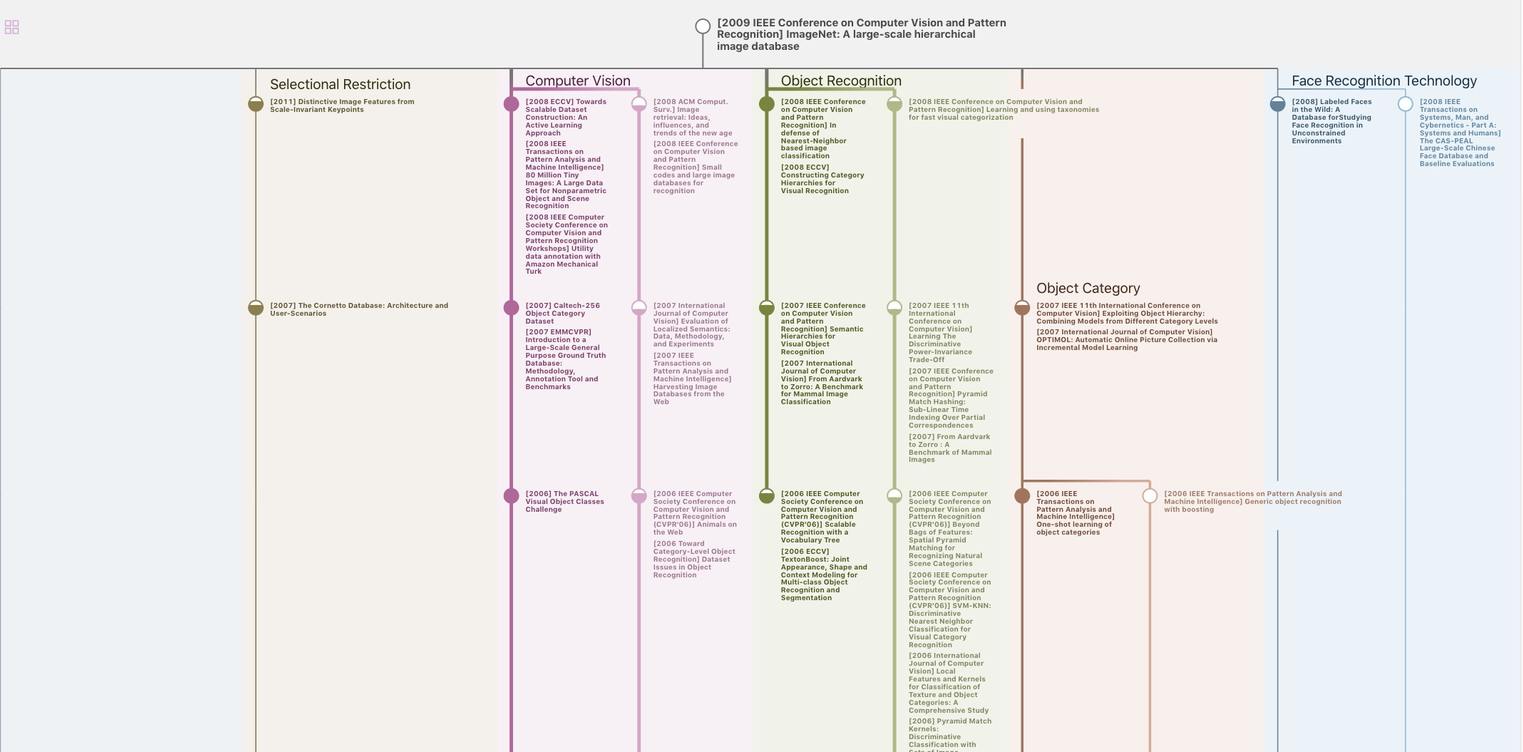
生成溯源树,研究论文发展脉络
Chat Paper
正在生成论文摘要