Towards Accountability for Machine Learning Datasets: Practices from Software Engineering and Infrastructure
FAccT(2021)
摘要
Rising concern for the societal implications of artificial intelligence systems has inspired demands for greater transparency and accountability. However the datasets which empower machine learning are often used, shared and re-used with little visibility into the processes of deliberation which led to their creation. Which stakeholder groups had their perspectives included when the dataset was conceived? Which domain experts were consulted regarding how to model subgroups and other phenomena? How were questions of representational biases measured and addressed? Who labeled the data? In this paper, we introduce a rigorous framework for dataset development transparency which supports decision-making and accountability. The framework uses the cyclical, infrastructural and engineering nature of dataset development to draw on best practices from the software development lifecycle. Each stage of the data development lifecycle yields a set of documents that facilitate improved communication and decision-making, as well as drawing attention the value and necessity of careful data work. The proposed framework is intended to contribute to closing the accountability gap in artificial intelligence systems, by making visible the often overlooked work that goes into dataset creation.
更多查看译文
关键词
Accountability,Data science,Computer science
AI 理解论文
溯源树
样例
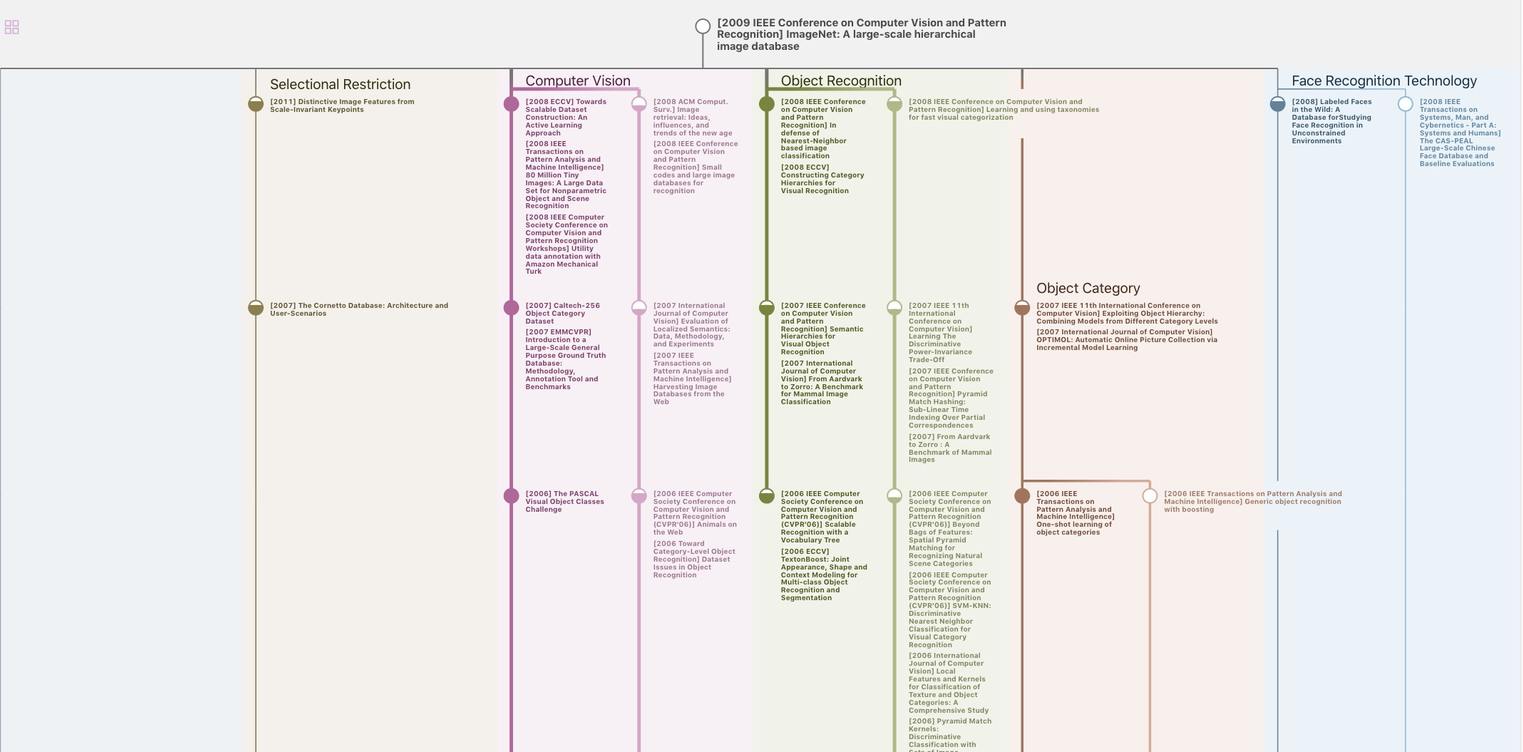
生成溯源树,研究论文发展脉络
Chat Paper
正在生成论文摘要