Semi-Disentangled Representation Learning in Recommendation System
arxiv(2020)
摘要
Disentangled representation has been widely explored in many fields due to its maximal compactness, interpretability and versatility. Recommendation system also needs disentanglement to make representation more explainable and general for downstream tasks. However, some challenges slow its broader application -- the lack of fine-grained labels and the complexity of user-item interactions. To alleviate these problems, we propose a Semi-Disentangled Representation Learning method (SDRL) based on autoencoders. SDRL divides each user/item embedding into two parts: the explainable and the unexplainable, so as to improve proper disentanglement while preserving complex information in representation. The explainable part consists of $internal\ block$ for individual-based features and $external\ block$ for interaction-based features. The unexplainable part is composed by $other\ block$ for other remaining information. Experimental results on three real-world datasets demonstrate that the proposed SDRL could not only effectively express user and item features but also improve the explainability and generality compared with existing representation methods.
更多查看译文
AI 理解论文
溯源树
样例
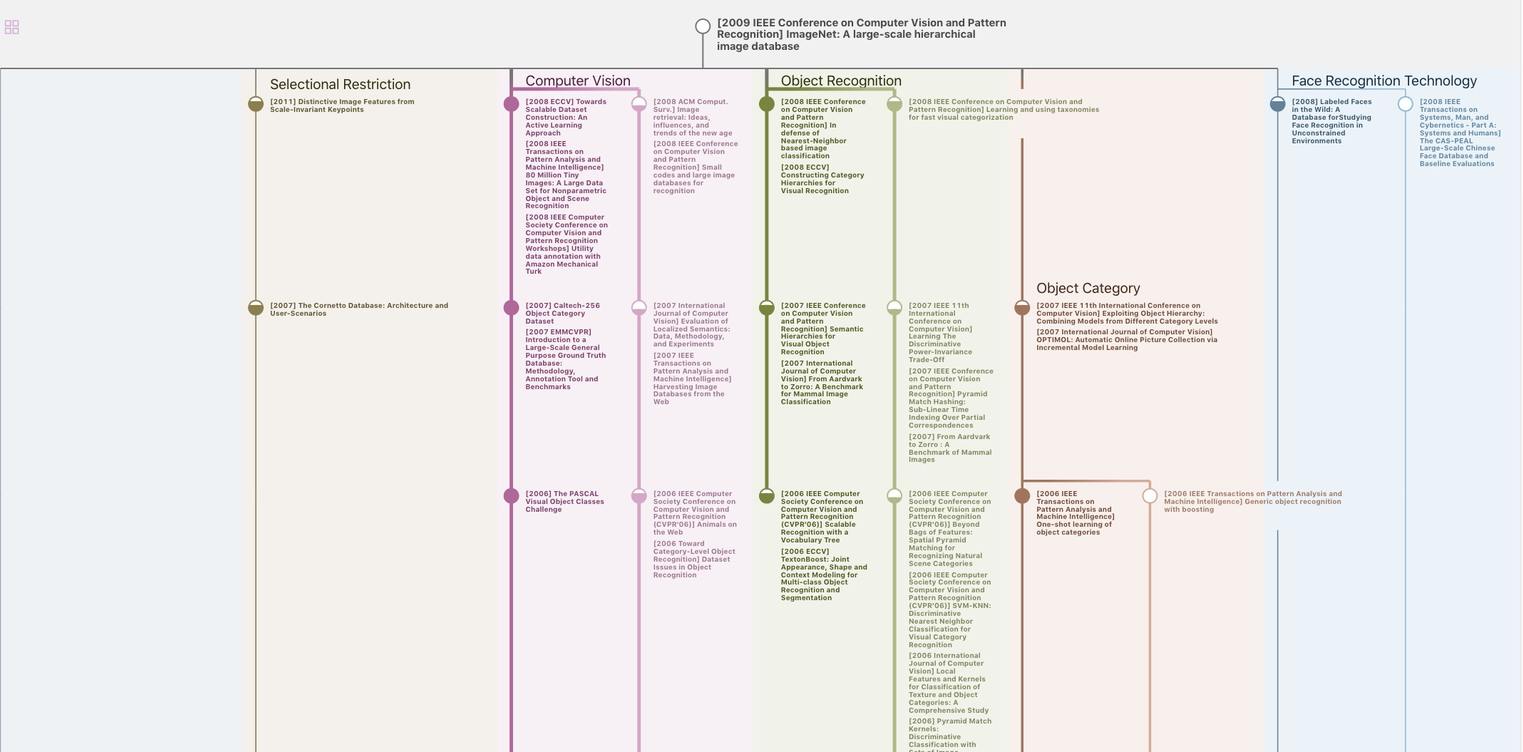
生成溯源树,研究论文发展脉络
Chat Paper
正在生成论文摘要