SUREMAP: PREDICTING UNCERTAINTY IN CNN-BASED IMAGE RECONSTRUCTIONS USING STEIN'S UNBIASED RISK ESTIMATE
2021 IEEE INTERNATIONAL CONFERENCE ON ACOUSTICS, SPEECH AND SIGNAL PROCESSING (ICASSP 2021)(2021)
摘要
Convolutional neural networks (CNN) have emerged as a powerful tool for solving computational imaging reconstruction problems. However, CNNs are generally difficult-to-understand black-boxes. Accordingly, it is challenging to know when they will work and, more importantly, when they will fail. This limitation is a major barrier to their use in safety-critical applications like medical imaging: Is that blob in the reconstruction an artifact or a tumor? In this work we use Stein's unbiased risk estimate (SURE) to develop per-pixel confidence intervals, in the form of heatmaps, for compressive sensing reconstruction using the approximate message passing (AMP) framework with CNN-based denoisers. These heatmaps tell end-users how much to trust an image formed by a CNN, which could greatly improve the utility of CNNs in various computational imaging applications.
更多查看译文
关键词
Compressive Sensing, Approximate Message Passing, CNN, MRI
AI 理解论文
溯源树
样例
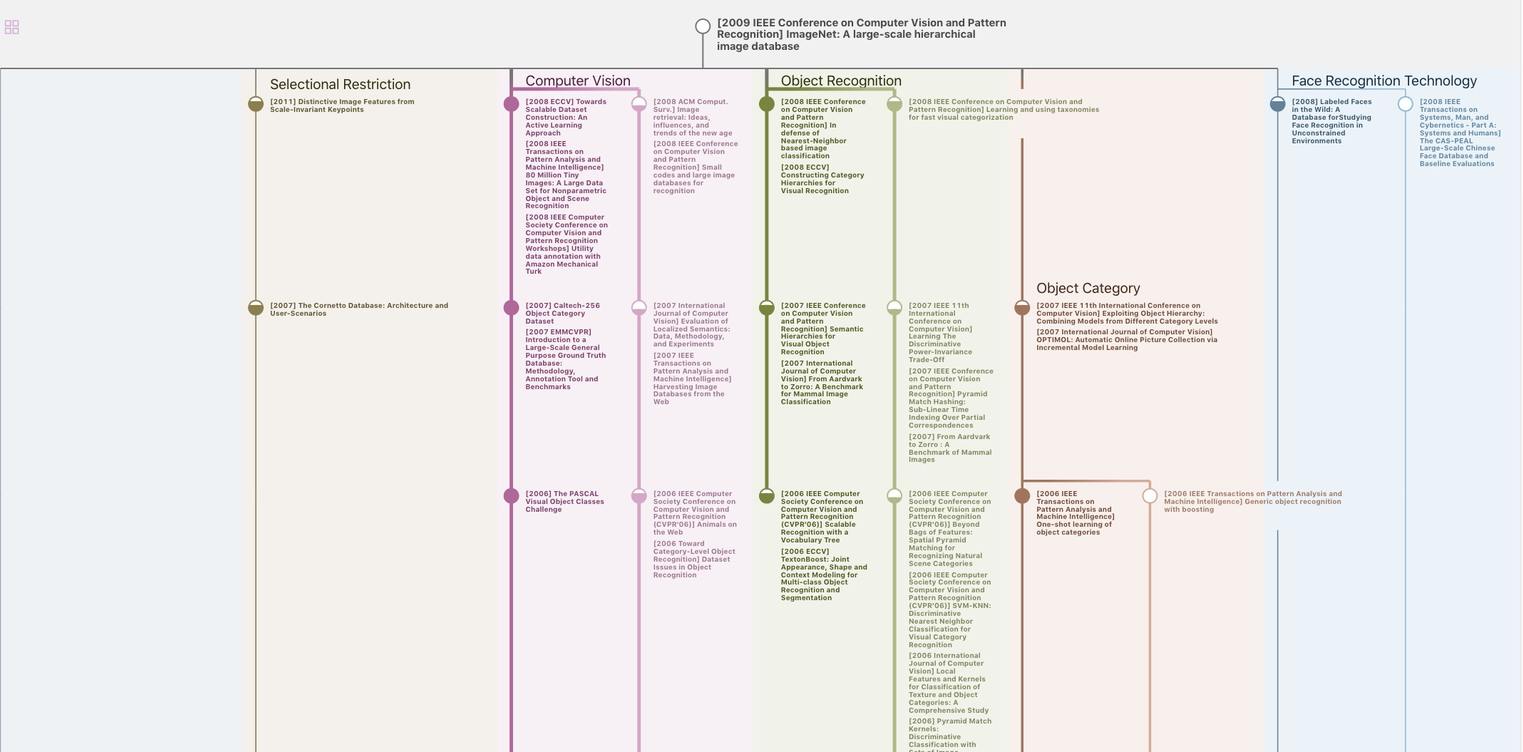
生成溯源树,研究论文发展脉络
Chat Paper
正在生成论文摘要