Implementation of machine learning models for the prediction of vaginal birth after cesarean delivery
JOURNAL OF MATERNAL-FETAL & NEONATAL MEDICINE(2022)
摘要
Objective: Accurate prediction of vaginal birth after cesarean is crucial for selecting women suitable for a trial of labor after cesarean (TOLAC). We sought to develop a machine learning (ML) model for prediction of TOLAC success and to compare its accuracy with that of the MFMU model. Methods: All consecutive singleton TOLAC deliveries from a tertiary academic medical center between February 2017 and December 2018 were included. We developed models using the following ML algorithms: random forest (RF), regularized regression (GLM), and eXtreme gradient-boosted decision trees (XGBoost). For developing the ML models, we disaggregated BMI into height and weight. Similarly, we disaggregated prior arrest of progression into prior arrest of dilatation and prior arrest of descent. We applied a nested cross-validation approach, using 100 random splits of the data to training (80%, 792 samples) and testing sets (20%, 197 samples). We used the area under the precision-recall curve (AUC-PR) as a measure of accuracy. Results: Nine hundred and eighty-nine TOLAC deliveries were included in the analysis with an observed TOLAC success rate of 85.6%. The AUC-PR in the RF, XGBoost and GLM models were 0.351 +/- 0.028, 0.350 +/- 0.028 and 0.336 +/- 0.024, respectively, compared to 0.325 +/- 0.067 for the MFMU-C. The algorithms performed significantly better than the MFMU-C (p-values = .0002, .0004, .0393 for RF, XGBoost, GLM respectively). In the XGBoost model, eight variables were sufficient for accurate prediction. In all ML models, previous vaginal delivery and height were among the three most important predictors of TOLAC success. Prior arrest of descent contributed to prediction more than prior arrest of dilatation, maternal height contributed more than weight. Conclusion: All ML models performed significantly better than the MFMU-C. In the XGBoost model, eight variables were sufficient for accurate prediction. Prior arrest of descent and maternal height contribute to prediction more than prior arrest of dilation and maternal weight.
更多查看译文
关键词
Artificial intelligence, machine learning, TOLAC, vaginal delivery, cesarean delivery
AI 理解论文
溯源树
样例
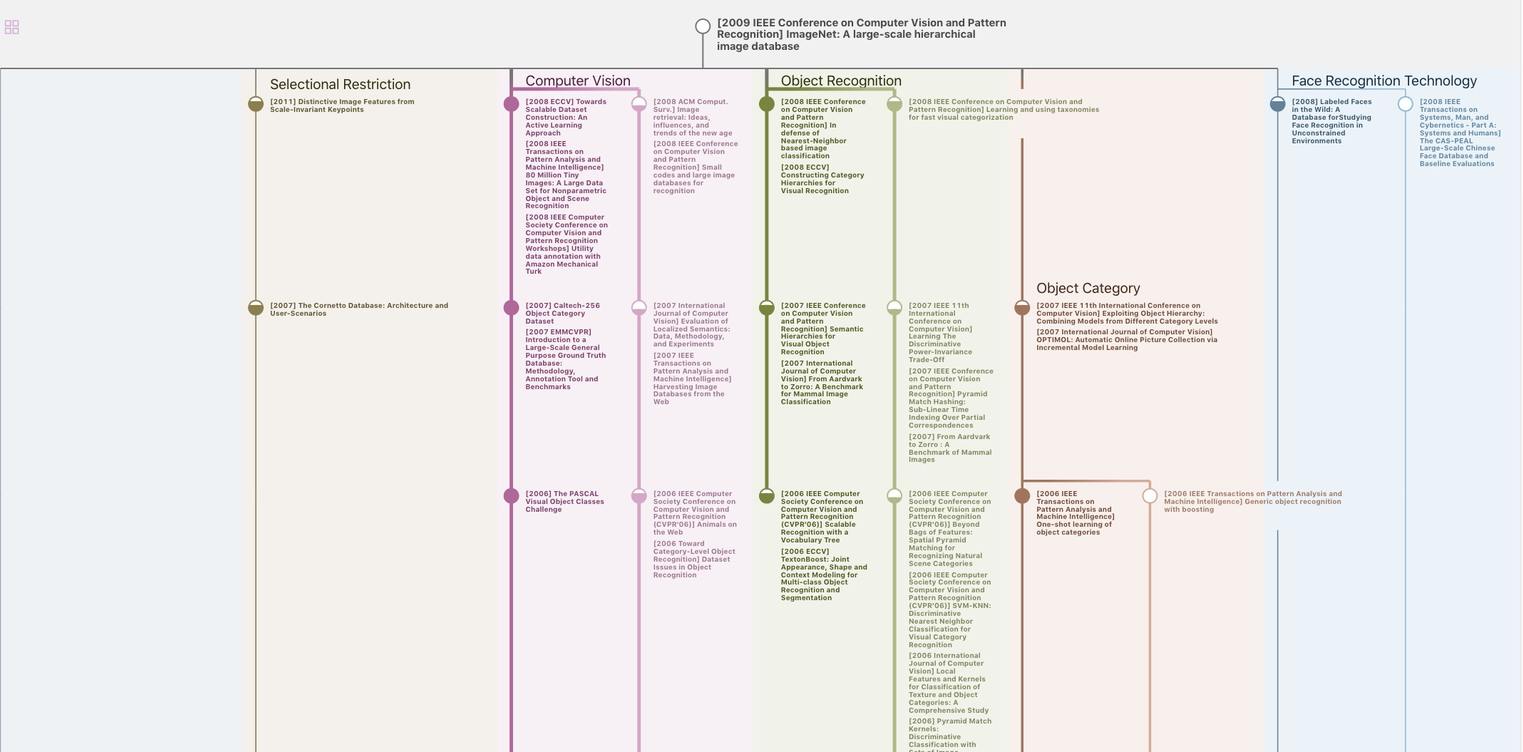
生成溯源树,研究论文发展脉络
Chat Paper
正在生成论文摘要