Image-To-Image Translation For Automatic Ink Removal In Whole Slide Images
JOURNAL OF MEDICAL IMAGING(2020)
摘要
Purpose: Deep learning models are showing promise in digital pathology to aid diagnoses. Training complex models requires a significant amount and diversity of well-annotated data, typically housed in institutional archives. These slides often contain clinically meaningful markings to indicate regions of interest. If slides are scanned with the ink present, then the downstream model may end up looking for regions with ink before making a classification. If scanned without the markings, the information regarding where the relevant regions are located is lost. A compromise solution is to scan the slide with the annotations present but digitally remove them.Approach: We proposed a straightforward framework to digitally remove ink markings from whole slide images using a conditional generative adversarial network based on Pix2Pix.Results: The peak signal-to-noise ratio increased 30%, structural similarity index increased 20%, and visual information fidelity increased 200% relative to previous methods.Conclusions: When comparing our digital removal of marked images with rescans of clean slides, our method qualitatively and quantitatively exceeds current benchmarks, opening the possibility of using archived clinical samples as resources to fuel the next generation of deep learning models for digital pathology. (C) 2020 Society of Photo Optical Instrumentation Engineers (SPIE)
更多查看译文
关键词
ink removal, whole slide image, image to image translation
AI 理解论文
溯源树
样例
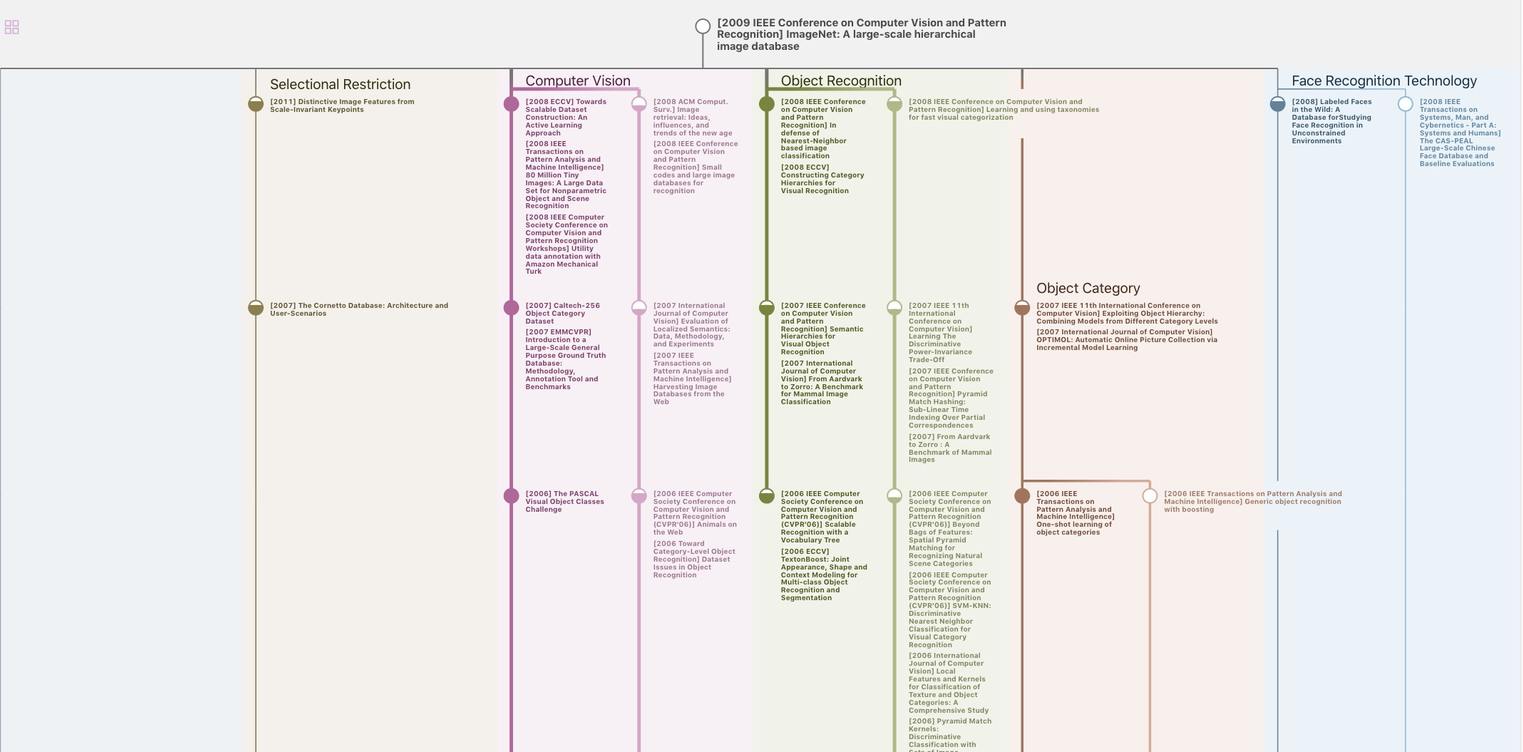
生成溯源树,研究论文发展脉络
Chat Paper
正在生成论文摘要