Rethinking embedding coupling in pre-trained language models
ICLR(2021)
摘要
We re-evaluate the standard practice of sharing weights between input and output embeddings in state-of-the-art pre-trained language models. We show that decoupled embeddings provide increased modeling flexibility, allowing us to significantly improve the efficiency of parameter allocation in the input embedding of multilingual models. By reallocating the input embedding parameters in the Transformer layers, we achieve dramatically better performance on standard natural language understanding tasks with the same number of parameters during fine-tuning. We also show that allocating additional capacity to the output embedding provides benefits to the model that persist through the fine-tuning stage even though the output embedding is discarded after pre-training. Our analysis shows that larger output embeddings prevent the model\u0027s last layers from overspecializing to the pre-training task and encourage Transformer representations to be more general and more transferable to other tasks and languages. Harnessing these findings, we are able to train models that achieve strong performance on the XTREME benchmark without increasing the number of parameters at the fine-tuning stage.
更多查看译文
关键词
language models,coupling,pre-trained
AI 理解论文
溯源树
样例
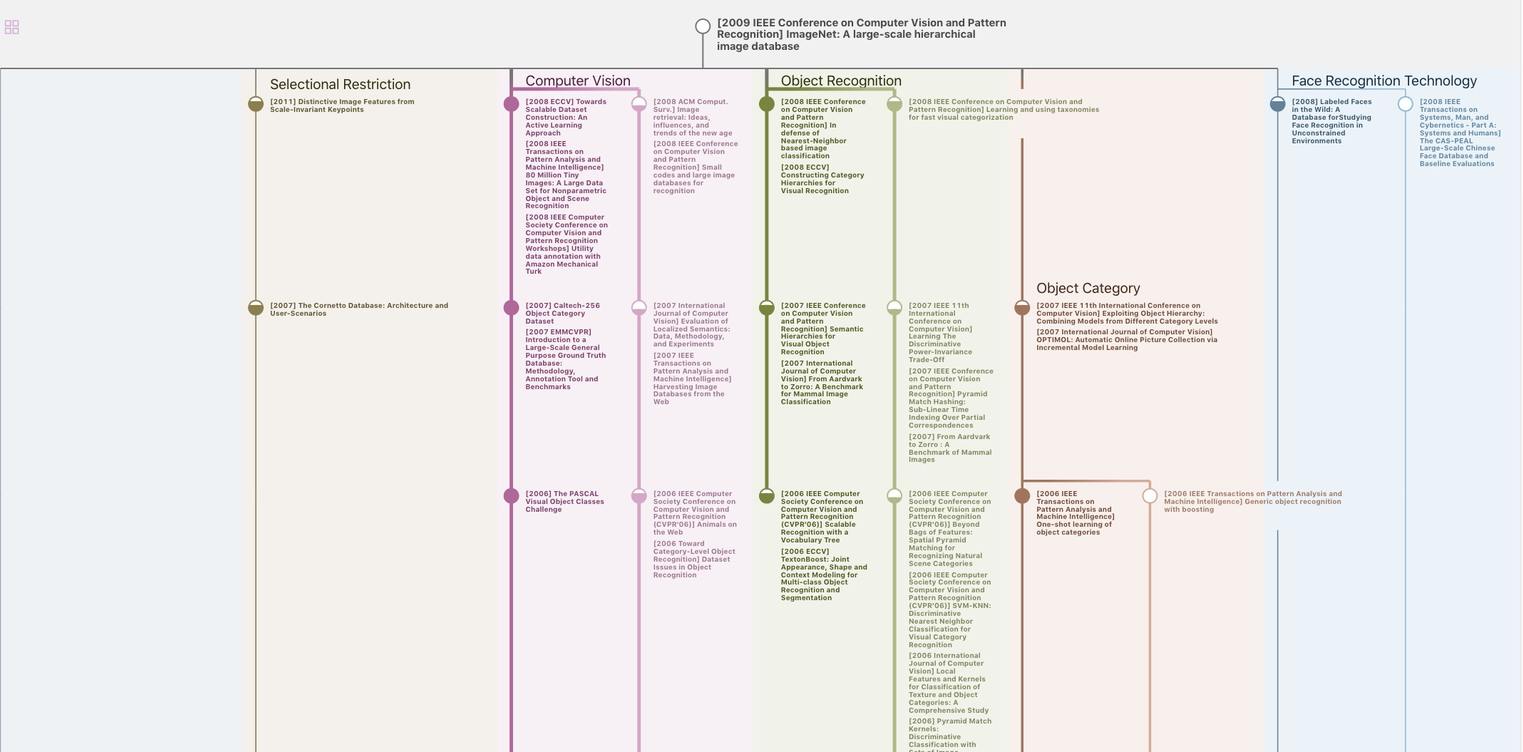
生成溯源树,研究论文发展脉络
Chat Paper
正在生成论文摘要