REDE: End-to-End Object 6D Pose Robust Estimation Using Differentiable Outliers Elimination
IEEE ROBOTICS AND AUTOMATION LETTERS(2021)
摘要
Object 6D pose estimation is a fundamental task in many applications. Conventional methods solve the task by detecting and matching the keypoints, then estimating the pose. Recent efforts bringing deep learning into the problem mainly overcome the vulnerability of conventional methods to environmental variation due to the hand-crafted feature design. However, these methods cannot achieve end-to-end learning and good interpretability at the same time. In this letter, we propose REDE, a novel end-to-end object pose estimator using RGB-D data, which utilizes network for keypoint regression, and a differentiable geometric pose estimator for pose error back-propagation. Besides, to achieve better robustness when outlier keypoint prediction occurs, we further propose a differentiable outliers elimination method that regresses the candidate result and the confidence simultaneously. Via confidence weighted aggregation of multiple candidates, we can reduce the effect from the outliers in the final estimation. Finally, following the conventional method, we apply a learnable refinement process to further improve the estimation. The experimental results on three benchmark datasets show that REDE slightly outperforms the state-of-the-art approaches and is more robust to object occlusion. Our code is available at https://github.com/HuaWeitong/REDE.
更多查看译文
关键词
Deep learning for visual perception,pose estimation,RGB-D perception
AI 理解论文
溯源树
样例
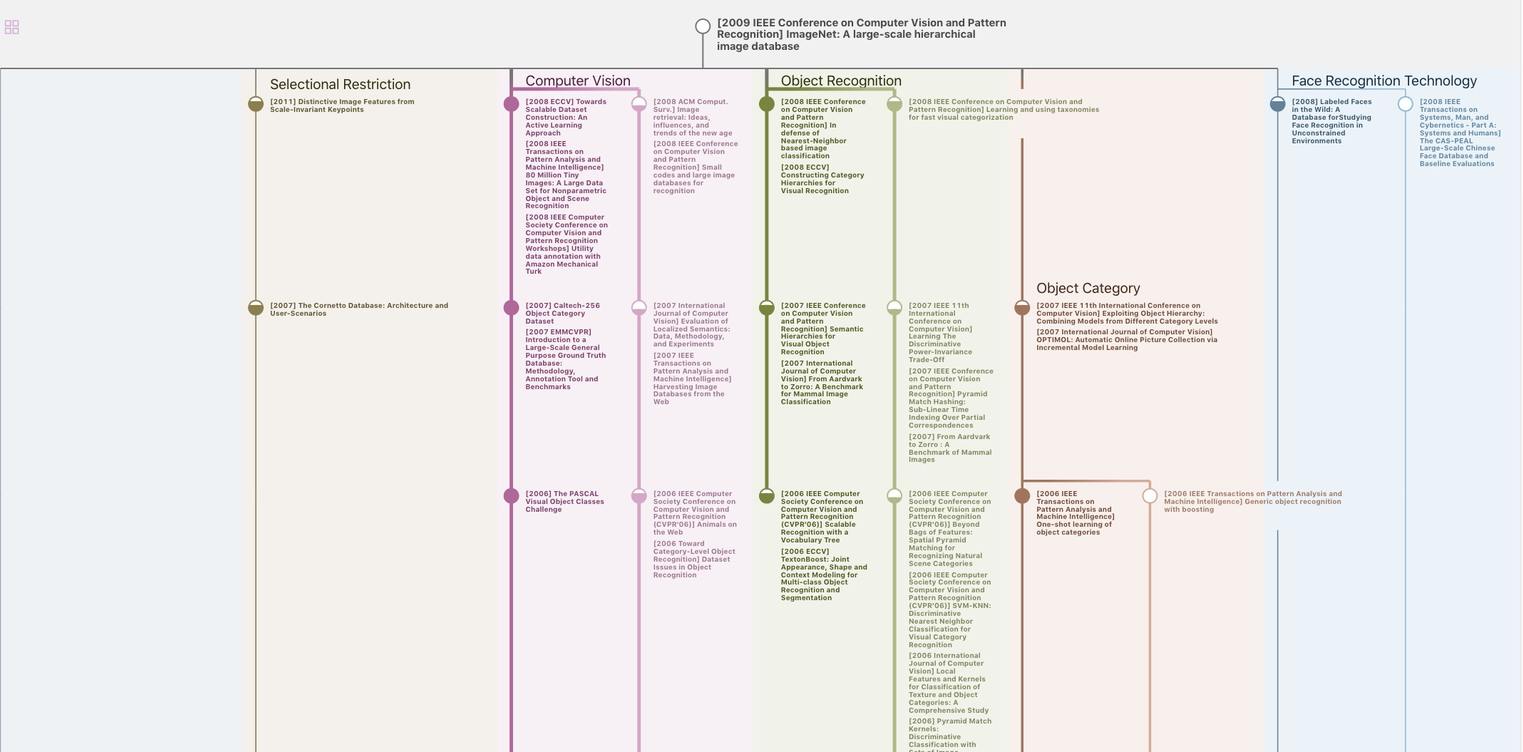
生成溯源树,研究论文发展脉络
Chat Paper
正在生成论文摘要