LCFI: A Fault Injection Tool for Studying Lossy Compression Error Propagation in HPC Programs
2020 IEEE INTERNATIONAL CONFERENCE ON BIG DATA (BIG DATA)(2020)
摘要
Error-bounded lossy compression is becoming more and more important to today's extreme-scale HPC applications because of the ever-increasing volume of data generated. Error-bounded lossy compressors have been widely used in in situ visualization, data stream intensity reduction, storage reduction, I/O performance improvement, checkpoint/restart acceleration, memory footprint reduction, etc. Although many works have optimized ratio, quality, and performance for different error-bounded lossy compressors, there is none of the existing works attempting to systematically understand the impact of lossy compression errors on HPC application due to error propagation. In this paper, we propose and develop a lossy compression fault injection tool, called LCFI. To the best of our knowledge, this is the first tool that help both lossy compressor developers and users to systematically and comprehensively understand the impact of lossy compression errors on any given HPC programs. The contributions of this work are threefold. (1) We propose an efficient approach to inject lossy compression faults according to a statistical analysis of compression errors for different state-of-the-art error-bounded lossy compressors. (2) We build a fault injector which is highly applicable, customizable, ease-to-use in generating top-down comprehensive results. We use a simple sample program to demonstrate the use of LCFI. (3) We evaluate LCFI on four representative HPC benchmark programs with different lossy compression errors and derive several important insights based on our observations.
更多查看译文
关键词
LCFI,lossy compression error propagation,error-bounded lossy compression,extreme-scale HPC applications,data stream intensity reduction,error-bounded lossy compressors,HPC application,lossy compression fault injection tool
AI 理解论文
溯源树
样例
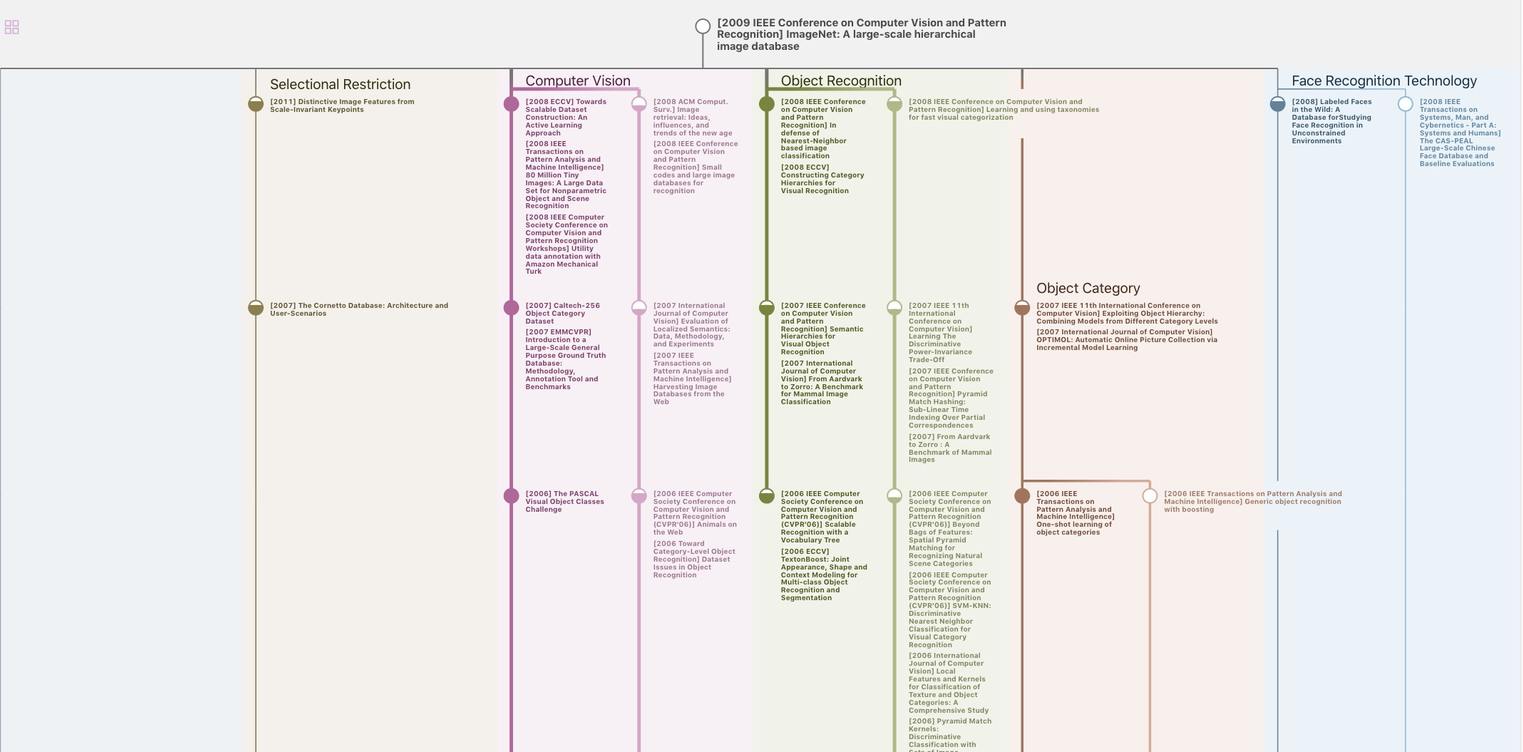
生成溯源树,研究论文发展脉络
Chat Paper
正在生成论文摘要